Data is the most precious currency for businesses today — but obtaining high-quality data can be a real challenge. It can be difficult for a number of reasons, including cost, privacy concerns, and more. Think about medical imaging, sensitive and harmful content, rare manufacturing defects, and financial data — there are always privacy pitfalls, ethical concerns, and general availability challenges. The promising solution to this conundrum — that more and more businesses are exploring — is synthetic data generation using generative AI.
It entails the process of creating artificial data which closely resembles real-world datasets, making it useful for training and testing machine learning models. Synthetic data is generated through algorithms to mimic the structure and statistical features of authentic data — but without the privacy issues, the high cost, and the potential bias.
Let’s delve into the details of what synthetic data generation is, what are its advantages and practical applications, and how at Imagga we make the best of the possibilities it offers.
Contents
What Is Synthetic Data, Really?
Generation of synthetic data is based on the premise of mimicking real datasets to create artificial data that can be used in various contexts. Synthetic data is modeled to have similar characteristics, such as structure, statistical properties and patterns, without employing real-world data items — but instead relying on computer simulations.
Real vs. Synthetic Data Generation
The gathering and analysis of data is a challenging process, but the value of the collected datasets is immense.
Real-world data used for business and software development purposes is usually collected from tracking and recording user interactions, financial transactions, and the like. Its analysis is then the solid ground for obtaining precious insights into patterns, user behavior, trends, and much more. Massive datasets are also necessary for AI training.
But sometimes real data is simply not available, is very difficult to obtain, is very costly, or there is a legal or ethical obstacle to its collection and management. Real data is also subject to various (and very necessary) data protection laws that protect people’s privacy. There are also the concerns about data bias and diversity in datasets, as well as the ownership of the data, which can be a big issue for some companies.
This is the pain point experienced by a myriad of businesses that synthetic data generation can address. Since it does not contain actual data points from the real-world, it is not owned or accessed by anyone else. It’s also quicker to obtain, can be modeled to a specific use case scenario, and is not subject to privacy laws.
Creating artificial data, of course, doesn’t come without its challenges. The subtleties and nuances in our lives, understandably, are not easy to recreate in synthetic data — and it still relies on real-world data, but to a much lesser extent.
The technological jumps in the generation methods, however, have made it possible for synthetic data today to be as close to reality as possible. Synthetic data now offers a high level of efficiency and freedom of use. Due to these advancements, it can be used in various scenarios for testing different types of systems and training AI models.
The Benefits of Using Synthetic Data
Synthetic data generation allows for the creation of massive volumes of data that can be used for various purposes. In particular it is becoming a game changer for software testing and training and refining of machine learning models.
Using synthetic data helps avoid issues with privacy protection. Since it mimics real-world data, but does not contain actual information about real individuals, it doesn’t fall under such restrictions. This makes it particularly useful in fields like healthcare and finance where a lot of sensitive data has to be handled.
Cost efficiency and scalability are surely two more big advantages of synthetic data. Gathering, organizing and managing real data is cost- and resource-heavy, plus has limitations in terms of volumes. Computer-generated data can address both the price tag and the scalability, since it is easier to obtain and can be produced in massive amounts.
With the use of synthetic data, companies can generate representative datasets and thus overcome data scarcity and lower data quality issues. Synthetic data generation can also augment real datasets to bring them closer to the required level.
The process of creating synthetic data can be tailored to avoid data bias and to offer the necessary level of different scenarios, ensuring fairness and diversity in the datasets.
Due to all these advantages, synthetic data is particularly useful for accelerating the training process of various AI models. It also presents promising opportunities for testing and validation of particularly challenging scenarios through the creation of controlled environments.
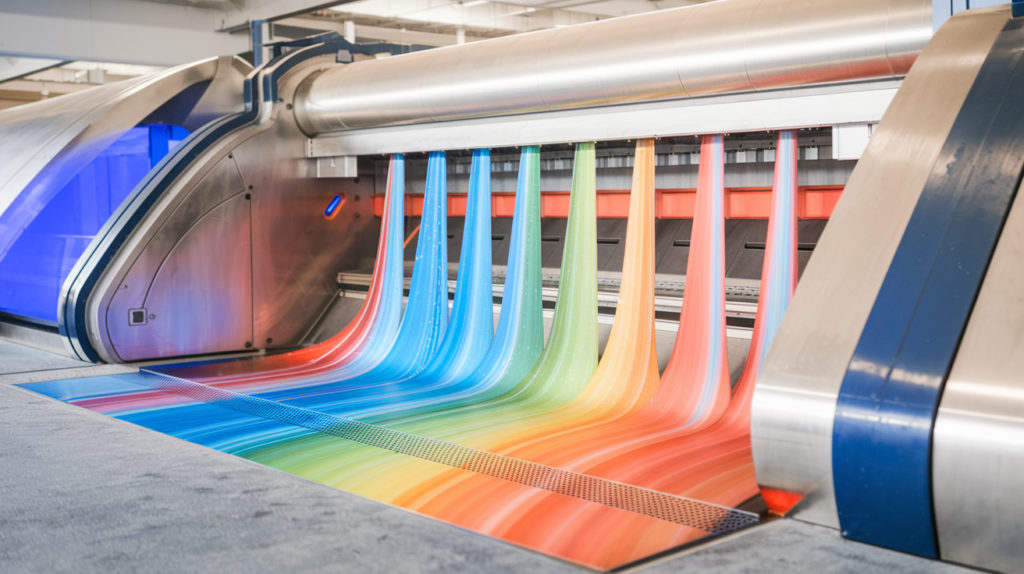
Real-World Applications of Synthetic Data
Synthetic data is already being used across industries. Its applications are wide, and its potential is growing.
Healthcare
Healthcare is a prime example of this. Since the field is highly sensitive and subject to various regulations, including HIPAA and the like, using real-world data can be quite challenging.
With the help of synthetic data, researchers and medical professionals can gain important insights, while patient protection is ensured. This is especially relevant in areas like medical imaging, AI diagnostics and similar innovative uses of AI in healthcare.
Retail and E-commerce
The applications of synthetic data in retail and e-commerce are also promising. It can be used to get valuable insights about customer behavior and to devise adequate pricing models.
Synthetic data can also come in handy in improving marketing automation models, as well as in improving product suggestions with the help of image recognition, and the like.
Autonomous Vehicles
The role of synthetic data in developing self-driving vehicles is significant. It allows for creation of simulation environments for in-depth testing — without the risks of conducting such experiments in reality.
Using synthetic data, specialists can observe the behavior of autonomous vehicles under different conditions. The application of simulations is also crucial in the aerospace and defense industries.
Finance
In finance, data privacy and protection can also be problematic and is subject to various regulations, including GDPR and CCPA. Synthetic data can be thus employed to overcome privacy restrictions by not using data that contains personally identifiable information (PII).
With its help, finance professionals can gain insights into financial trends, as well as test financial models and trading algorithms. In the finance industry, synthetic data is also useful for developing fraud detection models, simulating financial crises, stress testing, customer behavior analysis, legal impact analysis, and predictive analytics, among others.
Machine Learning and AI Training
The use of synthetic data is already widespread in machine learning and AI training. For example, it offers great potential for training image recognition models that are being used in a variety of industries and contexts. They power up features like facial recognition, object detection, and overall analysis of images, videos, and livestreams.
In Natural Language Processing (NLP), synthetic data can be used for improving translation between languages, as well as for text summaries and analysis.
Content Moderation
Content moderation has now become a must for digital platforms with user-generated content, but the process may still be challenging. Automated content moderation previously relied on training from real-world data, but can now also make use of the benefits of synthetic data.
Creating realistic and more varied artificial datasets is of immense benefit for improving the machine learning algorithms on which content moderation is based. Synthetic data generation can be used to improve training data, repair damaged or incomplete sets, and create additional data to complement limited sets.
In addition to these applications, synthetic data is also being widely used in various other types of software testing, robotics simulations, and more. It’s especially useful in fields where spotting trends and patterns in real-world data is difficult, inapplicable or has serious legal and ethical implications.
Imagga’s Custom Models and Synthetic Data Generation
At Imagga, we’re always exploring and applying cutting-edge technology in our solutions.
In particular, we’re leveraging the possibilities that synthetic data generation offers in the custom model training that we develop on demand for our customers.
AI image custom models are perfect for cases when generic models are not able to handle specialized or nuanced tasks that deviate from the training scope of the models. But custom models also have to be trained on datasets — and synthetic data presents a huge promise in overcoming a number of issues with providing the most appropriate training data.
Ready to explore the novel possibilities of machine learning algorithms in your business processes? Get in touch with us to find out how Imagga’s AI-powered solutions can revolutionize your operations.