Content moderation is the practice of monitoring and regulating user-generated content on digital platforms to ensure it meets established guidelines and community standards. From social media posts and comments to product reviews and forum discussions, content moderation plays a crucial role in maintaining online safety, preventing the spread of harmful content, and creating positive user experiences. As online platforms continue to grow and evolve, effective content moderation has become essential for businesses and organizations to protect their users, maintain brand reputation, and comply with increasingly stringent digital regulations. In this blog post we’ll go through the types of content moderation, the benefits and disadvantages of each and their use cases.
To get a deeper understanding of the fundamentals of content moderation, check out our in-depth guide: What is Content Moderation?
Contents
- Importance of Understanding Content Moderation Types
- Pre-Moderation
- Post-Moderation
- Reactive Moderation
- Distributed Moderation
- Automated Moderation
- What to Consider When Choosing a Content Moderation Strategy?
- AI Integration in Content Moderation
- Benefits of AI Integration
- Challenges and Considerations
- Effective Content Moderation in Action
- Future Trends in Content Moderation
- Conclusion
Importance of Understanding Content Moderation Types
Different platforms require different types of content moderation approaches based on their size, content type, and user base. A social media network with millions of active users will require a different strategy than a niche discussion forum. Understanding the various types of moderation allows platform owners to make informed decisions about how to safeguard their communities while ensuring seamless user experiences.
Pre-Moderation
Pre-moderation is a proactive approach where all user-generated content is reviewed and approved before being published on a platform. This method creates a queue of pending content that moderators must evaluate against community guidelines and acceptable content standards before allowing it to go live.
Advantages
This approach provides maximum content control, preventing harmful content and ensuring high-quality standards. It builds user trust by demonstrating a strong commitment to content safety.
Disadvantages
Pre-moderation causes publication delays, limiting real-time engagement. It requires significant human resources, making it costly and hard to scale. The waiting period may also frustrate users, reducing participation.
Use Cases
Ideal for platforms prioritizing safety and quality, such as children’s sites, educational forums, enterprise communication, and healthcare communities. It’s also valuable for branded communities, regulated industries, and platforms handling sensitive or confidential topics.
Post-Moderation
Post-moderation is a reactive approach where content is published immediately and reviewed afterward, either through user reports, automated flagging, or routine content audits. This method allows for immediate content visibility while maintaining oversight through subsequent review processes.
Advantages
Supports real-time interaction and engagement by allowing content to flow freely. The post-moderation approach reduces operational bottlenecks, scales efficiently, and is more cost-effective than pre-moderation by focusing on flagged content. Immediate publication keeps users engaged and active.
Disadvantages
Risks exposure to harmful content before removal, which can harm reputation and trust. Problematic content may go viral before moderation teams can act. Post-publication removals can frustrate users and lead to censorship concerns.
Use Cases
Best for high-volume platforms requiring real-time interaction, like social media, news comments, and large forums. Works well with mature audiences and automated filtering. Ideal for fast-paced environments like crisis communication and breaking news discussions.
Reactive Moderation
Reactive moderation is a user-driven approach where content is reviewed only after being flagged or reported by community members. This method relies heavily on user vigilance and community participation to identify potentially problematic content that requires moderator attention.
Advantages
Reactive moderation encourages user involvement, fostering a sense of ownership and shared responsibility. It’s cost-effective, requiring fewer moderators, and scales well as user reports grow with content volume. It also highlights issues most relevant to the community.
Disadvantages
Effectiveness depends on user engagement and guideline awareness, which can vary. Harmful content may remain visible until reported. Users may hesitate to report due to fear of retaliation, and malicious reporting can be exploited to harass others.
Use Cases
Best for mature communities with strong self-governance, like professional networks and niche forums. Works well when users understand and support platform standards. Reactive moderation is often used alongside other moderation methods for a balanced approach.
Distributed Moderation
Distributed moderation, also known as community-based moderation, delegates content review responsibilities across a network of trusted community members or volunteer moderators. This democratic approach combines the wisdom of the crowd with structured oversight to manage content at scale.
Advantages
This highly scalable system leverages community expertise to handle large content volumes. It fosters engagement by giving members a role in platform governance and ensures diverse perspectives in content evaluation. Distributed moderation is cost-effective, relying on passionate volunteers instead of paid staff. With moderators across time zones, it provides round-the-clock coverage without the expense of a full-time team.
Disadvantages
Moderation quality can be inconsistent due to individual biases and guideline interpretation. Managing volunteers requires coordination and training, and burnout or turnover can disrupt enforcement. Conflicts among moderators may arise, affecting community stability. Volunteers may also lack the training needed for complex or sensitive moderation decisions.
Use Cases
Ideal for large platforms with distinct subcommunities, such as Reddit or specialized forums. Works well where subject matter expertise is crucial, like technical or knowledge-sharing communities. Effective for platforms with strong community cultures, including gaming communities, open-source forums, and special interest groups with engaged users willing to moderate.
Automated Moderation
Automated content moderation employs artificial intelligence, machine learning algorithms, and rule-based systems to automatically screen, filter, and moderate content in real-time. This technology-driven approach uses natural language processing, computer vision, and pattern recognition to identify and handle potentially problematic content without human intervention.
Advantages
Automated content moderation offers unmatched speed and scalability, processing vast amounts of content instantly. It ensures 24/7 rule enforcement without fatigue or bias, maintaining consistency. These systems handle multiple formats (text, images, video) and languages, making them ideal for global platforms. They reduce reliance on human moderators, cutting costs while improving efficiency. Additionally, AI can detect patterns in violations, helping refine moderation strategies over time.
Disadvantages
AI struggles with context, nuance, and cultural differences, leading to false positives or overlooked violations. It may fail to detect sophisticated rule-breaking tactics that require human judgment. Implementation and maintenance are costly, demanding technical expertise and frequent updates. Users can also learn to bypass automated filters, and excessive reliance on automation may create an impersonal user experience.
Use Cases
Automated moderation is best suited for high-traffic platforms like social media, streaming services, and online marketplaces. Effective for detecting clear violations such as spam, explicit content, and banned material. Works well alongside human moderation, automating routine screening while escalating complex cases. Essential for platforms requiring real-time filtering, such as live-streaming or chat applications, where speed is critical for user safety.
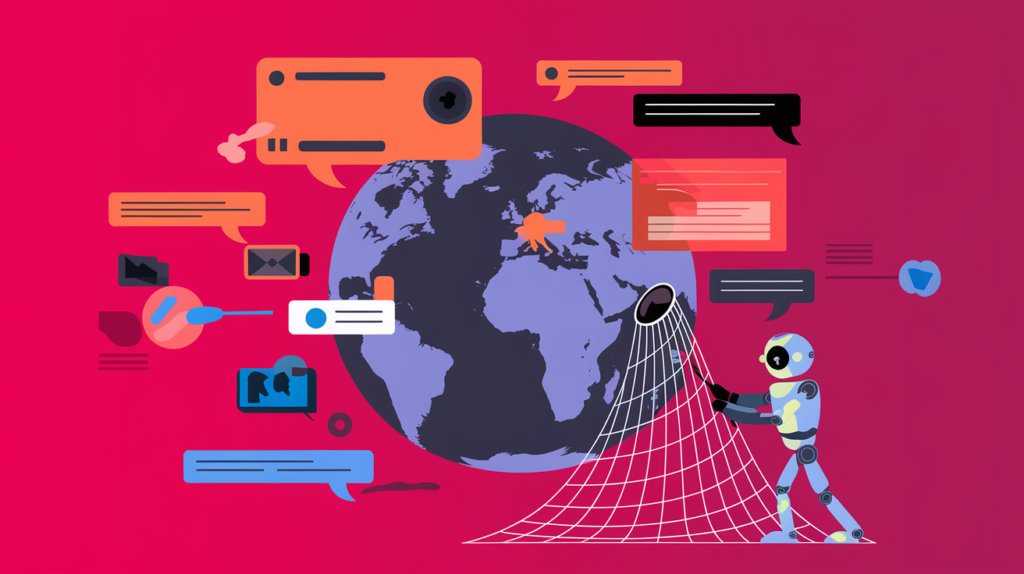
What to Consider When Choosing a Content Moderation Strategy?
Every platform must carefully evaluate its needs before implementing a content moderation strategy. The choice of moderation approach can significantly impact user satisfaction, platform growth, and community health. Several key factors influence this decision:
Platform Size and User Base
Larger platforms rely on automated moderation for scale, with human oversight for complex cases. Smaller platforms may prefer manual moderation to maintain quality while growing. User demographics matter – stricter pre-moderation suits younger audiences, while professional communities thrive with reactive moderation.
Content Type
Content type shapes moderation strategy. Text platforms use NLP for screening, while image and video sites need AI and human review. Live streaming requires real-time moderation, while forums benefit from pre-moderation process. Multi-format platforms often combine approaches for full coverage.
Community Guidelines
Community guidelines shape moderation needs. Strict platforms may require pre-moderation or strong AI filtering, while flexible ones can use post-moderation or community oversight. Complex rules demand more moderator training and refined automation. Clear guidelines for managing users generated content ensure consistent enforcement.
Resource Availability
Budget and resources shape moderation strategy. Automation has high upfront costs but scales efficiently, while human teams need ongoing training and management. Platforms must factor in infrastructure, tools, and expertise in AI, community management, and content policy.
AI Integration in Content Moderation
Advancements in artificial intelligence are transforming content moderation. AI-powered systems enhance efficiency, consistency, and accuracy, enabling platforms to handle vast amounts of content at scale while reducing reliance on human moderators. These technologies help detect and address harmful content more effectively, improving user experience and platform safety.
Machine Learning Algorithms
AI-driven machine learning models continuously refine their ability to detect harmful content by analyzing large datasets. These systems learn from historical moderation decisions, adapting to evolving trends in inappropriate or harmful material. As they process more data, they become better at distinguishing between acceptable content and violations, reducing false positives and negatives. This adaptability makes AI moderation increasingly effective over time, particularly in identifying new forms of harmful behavior, such as emerging slang or disguised hate speech.
Natural Language Processing (NLP)
NLP allows AI to analyze text, detecting hate speech, spam, and misinformation with contextual understanding. It recognizes sarcasm, coded language, and prioritizes flagged content by severity. Essential for moderating social media, forums, and news discussions.
Image and Video Recognition
AI-powered image and video recognition tools analyze visual content to detect explicit imagery, violent content, and other policy violations. These systems can identify nudity, graphic violence, and even manipulated media in images and videos. Advanced models also recognize context, differentiating between harmful visuals and legitimate content, such as medical or educational imagery. By automating visual content moderation, AI significantly reduces the burden on human moderators while ensuring faster and more consistent enforcement of platform guidelines.
Benefits of AI Integration
AI-powered moderation systems offer significant advantages, improving the efficiency, accuracy, and speed of content review processes. By leveraging machine learning, natural language processing, and image recognition, AI helps platforms maintain a safe and engaging environment while reducing the workload for human moderators.
Scalability
One of AI’s biggest strengths is its ability to handle vast amounts of content at scale. Unlike human moderation teams, which are limited by time and resources, AI can analyze millions of text, image, and video submissions simultaneously. This is crucial for large social media platforms, forums, and e-commerce sites, where user-generated content is continuously uploaded. AI ensures that platforms remain manageable even as user engagement grows, allowing businesses to expand without compromising content oversight.
Consistency
AI moderation applies content standards uniformly, reducing the subjectivity and bias that can come with human decision-making. While human moderators may interpret policies differently, AI enforces rules with strict adherence to predefined guidelines. This ensures fair and predictable moderation of harmful content, reducing the risk of disputes over inconsistent enforcement. Platforms benefit from a more reliable user experience where moderation outcomes remain steady across different cases, languages, and regions.
Real-Time Moderation
Speed is essential in content moderation, and AI provides near-instantaneous analysis of content, identifying harmful material the moment it is posted. This rapid response helps prevent the spread of inappropriate content, misinformation, or harmful behavior before it gains traction. AI’s ability to detect and act on violations in real time is especially valuable in live-streaming, chat platforms, and news comment sections, where quick intervention is necessary to maintain a safe and respectful environment.
Challenges and Considerations
While AI-powered moderation offers efficiency and scalability, it also presents challenges that platforms must carefully address. From understanding context to ethical concerns, these issues require a balanced approach that often combines automation with human oversight.
Contextual Understanding
AI struggles with interpreting nuance, sarcasm, and cultural variations in communication. A phrase that is offensive in one context may be harmless or even humorous in another. Language evolves quickly, and AI may misinterpret emerging slang, coded speech, or regional dialects. Without human review, automated systems risk making moderation decisions that feel arbitrary or unfair, potentially alienating users.
False Positives/Negatives
Automated systems are not perfect and may incorrectly flag benign content (false positives) or fail to detect harmful material (false negatives). Overly strict filters can mistakenly remove legitimate discussions, while lenient models may allow inappropriate content to slip through. Striking the right balance requires continuous refinement, user feedback loops, and hybrid moderation approaches where AI flags content for human review in ambiguous cases.
Ethical Implications
AI moderation raises concerns about censorship and user privacy. Overly aggressive content filtering can suppress free expression, leading to accusations of bias or unfair content removal. On the other hand, automated moderation often involves analyzing user-generated content, raising questions about data privacy and surveillance. Platforms must navigate these concerns transparently, ensuring that moderation policies align with ethical guidelines and user rights.
Effective Content Moderation in Action
Imagga AI-driven Content Moderation Platform provides a state-of-the-art solution for online platforms to automatically filter offensive, harmful, and illegal visual content as well as to detect AI-generated visual content. Engineered to meet the trust and safety policies of online platforms, it ensures user protection and mitigates organizational reputational risks. The solution is versatile, deployable in the Cloud, on-premise, or on edge/mobile devices, making it ideal for any platform that needs robust visual content moderation.
Future Trends in Content Moderation
As content moderation evolves, several key trends are shaping the future:
AI and Machine Learning Innovations
Improvements in AI technology will enhance contextual understanding and decision-making accuracy.
User Empowerment Tools
New features will allow users to customize their content experience, offering greater control over what they see.
Regulatory Developments
Governments and organizations will continue refining legal frameworks to address content moderation challenges and responsibilities. Check out our comprehensive guide to content moderation regulations.
Conclusion
Choosing the right moderation strategy is essential for ensuring user safety, content integrity, and platform growth. Whether leveraging AI, community-driven approaches, or human moderators, platforms must strike the right balance between oversight and engagement.
Platforms consider factors such as user base size, content type, and compliance requirements to determine the best moderation strategy.
Yes, many platforms combine different methods, such as AI filtering with human review, to ensure a balanced approach.
Users contribute by reporting violations, participating in distributed moderation, and shaping platform guidelines.
The three main types are pre-moderation, post-moderation, and reactive moderation, each serving different needs and objectives.