Synthetic Data Generation & How You Can Leverage It
Data is the most precious currency for businesses today — but obtaining high-quality data can be a real challenge. It can be difficult for a number of reasons, including cost, privacy concerns, and more. Think about medical imaging, sensitive and harmful content, rare manufacturing defects, and financial data — there are always privacy pitfalls, ethical concerns, and general availability challenges. The promising solution to this conundrum — that more and more businesses are exploring — is synthetic data generation using generative AI.
It entails the process of creating artificial data which closely resembles real-world datasets, making it useful for training and testing machine learning models. Synthetic data is generated through algorithms to mimic the structure and statistical features of authentic data — but without the privacy issues, the high cost, and the potential bias.
Let’s delve into the details of what synthetic data generation is, what are its advantages and practical applications, and how at Imagga we make the best of the possibilities it offers.
What Is Synthetic Data, Really?
Generation of synthetic data is based on the premise of mimicking real datasets to create artificial data that can be used in various contexts. Synthetic data is modeled to have similar characteristics, such as structure, statistical properties and patterns, without employing real-world data items — but instead relying on computer simulations.
Real vs. Synthetic Data Generation
The gathering and analysis of data is a challenging process, but the value of the collected datasets is immense.
Real-world data used for business and software development purposes is usually collected from tracking and recording user interactions, financial transactions, and the like. Its analysis is then the solid ground for obtaining precious insights into patterns, user behavior, trends, and much more. Massive datasets are also necessary for AI training.
But sometimes real data is simply not available, is very difficult to obtain, is very costly, or there is a legal or ethical obstacle to its collection and management. Real data is also subject to various (and very necessary) data protection laws that protect people’s privacy. There are also the concerns about data bias and diversity in datasets, as well as the ownership of the data, which can be a big issue for some companies.
This is the pain point experienced by a myriad of businesses that synthetic data generation can address. Since it does not contain actual data points from the real-world, it is not owned or accessed by anyone else. It’s also quicker to obtain, can be modeled to a specific use case scenario, and is not subject to privacy laws.
Creating artificial data, of course, doesn’t come without its challenges. The subtleties and nuances in our lives, understandably, are not easy to recreate in synthetic data — and it still relies on real-world data, but to a much lesser extent.
The technological jumps in the generation methods, however, have made it possible for synthetic data today to be as close to reality as possible. Synthetic data now offers a high level of efficiency and freedom of use. Due to these advancements, it can be used in various scenarios for testing different types of systems and training AI models.
The Benefits of Using Synthetic Data
Synthetic data generation allows for the creation of massive volumes of data that can be used for various purposes. In particular it is becoming a game changer for software testing and training and refining of machine learning models.
Using synthetic data helps avoid issues with privacy protection. Since it mimics real-world data, but does not contain actual information about real individuals, it doesn’t fall under such restrictions. This makes it particularly useful in fields like healthcare and finance where a lot of sensitive data has to be handled.
Cost efficiency and scalability are surely two more big advantages of synthetic data. Gathering, organizing and managing real data is cost- and resource-heavy, plus has limitations in terms of volumes. Computer-generated data can address both the price tag and the scalability, since it is easier to obtain and can be produced in massive amounts.
With the use of synthetic data, companies can generate representative datasets and thus overcome data scarcity and lower data quality issues. Synthetic data generation can also augment real datasets to bring them closer to the required level.
The process of creating synthetic data can be tailored to avoid data bias and to offer the necessary level of different scenarios, ensuring fairness and diversity in the datasets.
Due to all these advantages, synthetic data is particularly useful for accelerating the training process of various AI models. It also presents promising opportunities for testing and validation of particularly challenging scenarios through the creation of controlled environments.
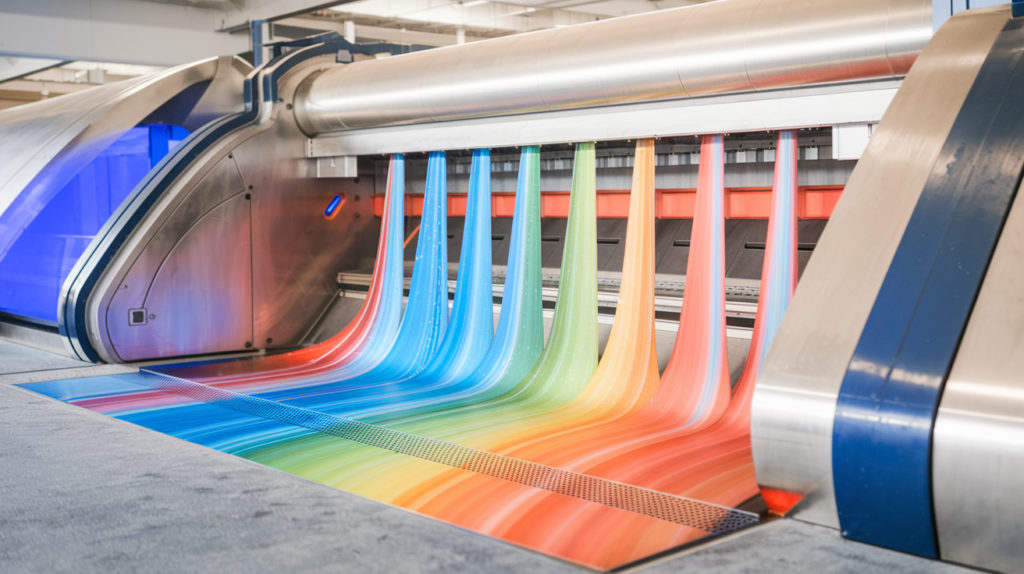
Real-World Applications of Synthetic Data
Synthetic data is already being used across industries. Its applications are wide, and its potential is growing.
Healthcare
Healthcare is a prime example of this. Since the field is highly sensitive and subject to various regulations, including HIPAA and the like, using real-world data can be quite challenging.
With the help of synthetic data, researchers and medical professionals can gain important insights, while patient protection is ensured. This is especially relevant in areas like medical imaging, AI diagnostics and similar innovative uses of AI in healthcare.
Retail and E-commerce
The applications of synthetic data in retail and e-commerce are also promising. It can be used to get valuable insights about customer behavior and to devise adequate pricing models.
Synthetic data can also come in handy in improving marketing automation models, as well as in improving product suggestions with the help of image recognition, and the like.
Autonomous Vehicles
The role of synthetic data in developing self-driving vehicles is significant. It allows for creation of simulation environments for in-depth testing — without the risks of conducting such experiments in reality.
Using synthetic data, specialists can observe the behavior of autonomous vehicles under different conditions. The application of simulations is also crucial in the aerospace and defense industries.
Finance
In finance, data privacy and protection can also be problematic and is subject to various regulations, including GDPR and CCPA. Synthetic data can be thus employed to overcome privacy restrictions by not using data that contains personally identifiable information (PII).
With its help, finance professionals can gain insights into financial trends, as well as test financial models and trading algorithms. In the finance industry, synthetic data is also useful for developing fraud detection models, simulating financial crises, stress testing, customer behavior analysis, legal impact analysis, and predictive analytics, among others.
Machine Learning and AI Training
The use of synthetic data is already widespread in machine learning and AI training. For example, it offers great potential for training image recognition models that are being used in a variety of industries and contexts. They power up features like facial recognition, object detection, and overall analysis of images, videos, and livestreams.
In Natural Language Processing (NLP), synthetic data can be used for improving translation between languages, as well as for text summaries and analysis.
Content Moderation
Content moderation has now become a must for digital platforms with user-generated content, but the process may still be challenging. Automated content moderation previously relied on training from real-world data, but can now also make use of the benefits of synthetic data.
Creating realistic and more varied artificial datasets is of immense benefit for improving the machine learning algorithms on which content moderation is based. Synthetic data generation can be used to improve training data, repair damaged or incomplete sets, and create additional data to complement limited sets.
In addition to these applications, synthetic data is also being widely used in various other types of software testing, robotics simulations, and more. It’s especially useful in fields where spotting trends and patterns in real-world data is difficult, inapplicable or has serious legal and ethical implications.
Imagga’s Custom Models and Synthetic Data Generation
At Imagga, we’re always exploring and applying cutting-edge technology in our solutions.
In particular, we’re leveraging the possibilities that synthetic data generation offers in the custom model training that we develop on demand for our customers.
AI image custom models are perfect for cases when generic models are not able to handle specialized or nuanced tasks that deviate from the training scope of the models. But custom models also have to be trained on datasets — and synthetic data presents a huge promise in overcoming a number of issues with providing the most appropriate training data.
Ready to explore the novel possibilities of machine learning algorithms in your business processes? Get in touch with us to find out how Imagga’s AI-powered solutions can revolutionize your operations.
Imagga Joins CLAIRE: A Major Step Toward Strengthening European AI Excellence
Imagga, a leading AI and image recognition company, is proud to announce its membership in the Confederation of Laboratories for Artificial Intelligence Research in Europe (CLAIRE). This significant step aligns with Imagga's commitment to advancing AI research and innovation within Europe and contributing to the continent's leadership in the global AI landscape.
CLAIRE Vision for Europe
Launched in 2018, CLAIRE (Confederation of Laboratories for Artificial Intelligence Research in Europe) is a visionary initiative to foster European excellence in AI research and innovation. CLAIRE's extensive network of AI research labs forms a pan-European confederation dedicated to ensuring that Europe remains at the forefront of AI advancements. The initiative draws inspiration from the success of CERN, aiming for similar brand recognition and impact, but in the AI domain.
CLAIRE's mission is to develop trustworthy AI technologies that augment human intelligence rather than replace it, benefiting European citizens and society. The organization seeks to address grand societal challenges, including climate change, energy, mobility, food security, healthcare, and inclusive, secure societies.
Imagga's Contribution to European AI Ecosystem
By joining CLAIRE, Imagga reinforces its dedication to contributing to the European AI ecosystem. This collaboration will enhance Imagga's ability to leverage CLAIRE's extensive network and state-of-the-art facilities, driving innovation and promoting knowledge exchange among Europe's brightest AI minds.
“Imagga is excited to join CLAIRE and contribute to the collective effort of making ‘AI made in Europe’ a global standard,” said Georgi Kadrev, CEO of Imagga. “We believe that our expertise in image recognition and AI solutions will complement CLAIRE’s mission to foster innovation and excellence in AI research across Europe.”
The Importance of European Leadership in AI
AI is a transformative technology with the potential to drive progress across all sectors of the economy and society. However, Europe has lagged in turning new AI technologies into global products and services, increasing its dependence on non-European AI solutions. This dependency poses risks to Europe’s economic, strategic, and cultural sovereignty.
CLAIRE aims to reverse this trend by establishing a pan-European network of Centres of Excellence in AI and a central facility with Google-scale, CERN-like infrastructure. This initiative will support existing talent, promote new research opportunities, and ensure that AI developments align with European values and needs.
CLAIRE's Vision for the Future
CLAIRE envisions a future where Europe leads in AI research and innovation, with state-of-the-art AI technologies developed and controlled within Europe. This vision includes:
- Enhanced Funding and Infrastructure: Advocating for significant investments in AI-specific computational infrastructure and research networks.
- Collaborative Research Environment: Creating an inclusive environment where researchers from various stages of their careers can collaborate and exchange knowledge.
- Public and Private Sector Collaboration: Engaging with stakeholders to foster innovation, citizen engagement, and industry collaboration.
- Human-Centered AI: Focusing on AI technologies that augment human capabilities and are developed with transparency and societal values in mind.
Imagga's membership in CLAIRE marks a significant milestone in the company's journey to advance AI research and innovation. Together with CLAIRE, Imagga is poised to contribute to Europe's ambition to become a global leader in AI, ensuring that AI technologies developed in Europe are competitive, trustworthy, and beneficial to society.
Imagga at the CEPIC Congress 2024: Embracing Authenticity in the Age of AI
Imagga participated in CEPIC Congress 2024, the largest networking hub for visual media businesses worldwide. This year's event took place in the picturesque Juan les Pins, Antibes, in the South of France, a fitting location given its historical significance as the birthplace of photography. From May 15th to 17th, industry leaders, innovators, and professionals gathered to explore the theme "Authenticity in the Age of AI."
Imagga's Presence and Participation
Imagga's CEO, Georgi Kadrev, was honored to be a panelist in one of the discussions at the congress. His insights on the diversity of industrial applications of AI were well-received, highlighting the evolving landscape of the professional photo industry and the growing integration of AI technologies.
"It's great to see how the professional photo industry embraces AI and adapts to emerging trends. While fair legislation is necessary, the industry must also adapt to this new reality. Imagga's participation in CEPIC underscores our commitment to staying at the forefront of industry developments. We believe that by engaging with peers and exploring new technologies, we can continue to innovate and provide cutting-edge solutions to our clients", said Georgi Kadrev, CEO of Imagga.
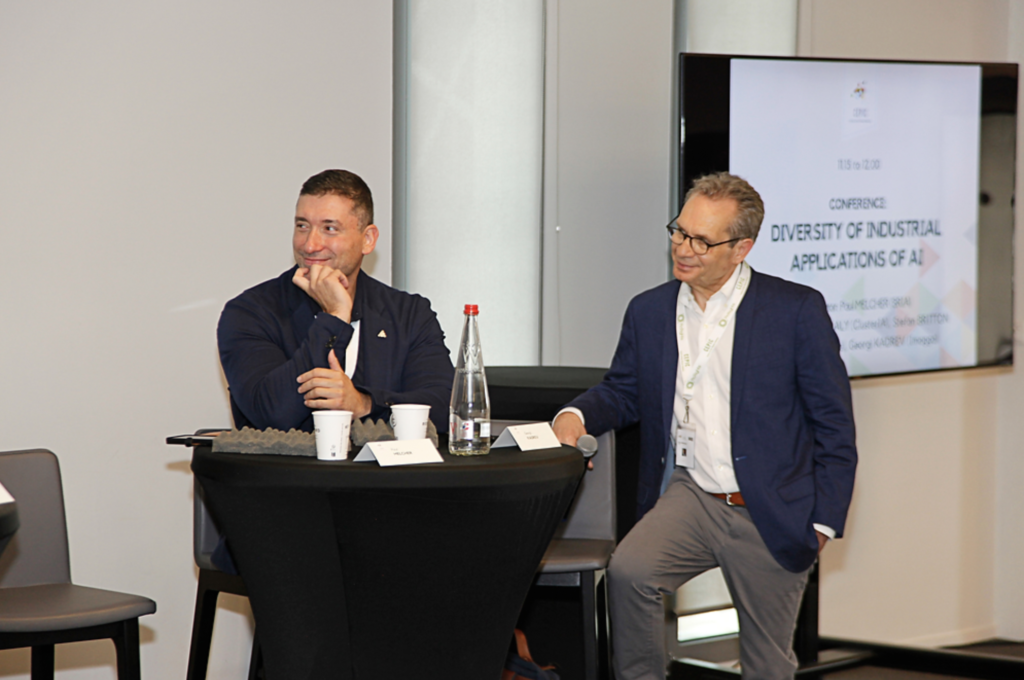
Key Takeaways from the Panel Discussions
Georgi Kadrev shared his observations from the event, emphasizing the industry's proactive approach to adopting new technologies.
The discussions at CEPIC covered a wide array of topics, reflecting the rapid technological shifts and the advent of new business models. A significant focus was on the augmentation of professional images and the ethical implications of AI in content creation. The industry is grappling with the challenge of generative AI, particularly concerning the use of photographers' images for training these models. Proposed solutions included licensing deals with generative AI companies to ensure fair compensation and rights protection.
One of the most fascinating moments was co-panelist Stefan Britton's presentation on a groundbreaking startup. This venture, which he supports as an angel investor, is working on converting brain waves into images. By analyzing brain waves from multiple subjects viewing 10,000 images, they aim to reverse-engineer the process to generate images directly from brain activity. This innovative approach exemplifies AI’s potential to push visual media’s boundaries.
The Importance of Networking and Collaboration
The CEPIC Congress is renowned for its networking opportunities, bringing together visual media professionals from over 30 countries. Attendees had the chance to engage in meaningful discussions, attend informative panels, and participate in specialized workshops. The event also featured pitch sessions, an Olympics Photo Exhibition, and the Annual Industry Party, sponsored by Shutterstock, fostering a vibrant community atmosphere.
Looking Ahead: Embracing AI with Authenticity
As we reflect on our experience at CEPIC 2024, we are optimistic about the future of the visual media industry. The integration of AI offers exciting possibilities, from enhancing image authenticity to creating entirely new forms of visual content. However, it is crucial to balance innovation with ethical considerations, ensuring that the rights of content creators are respected and protected.
We look forward to continuing our journey in the visual media landscape, leveraging AI to drive authenticity and creativity. Stay tuned for more updates from Imagga as we explore new horizons and push the boundaries of what is possible in the world of visual media.
Thank you to CEPIC for hosting such a fantastic event and to all the attendees who made it an unforgettable experience. See you next year!
CounteR Project Successfully Develops Innovative Tool for Detecting Radical Content Online
Horizon Europe-funded Project Completes Three-Year Mission to Combat Radicalization
30 April 2024 marks the conclusion of the CounteR Project, titled "Countering radicalization for a safer world: privacy-first situational awareness platform for violent terrorism and crime prediction, counter radicalization and citizen protection."
The project's standout achievement is the Counter Platform, an advanced early-warning system designed to identify radical content online. Operational in 12 languages, the CounteR Platform offers proactive content monitoring across various online platforms, including the open, deep, and dark webs. It is equipped to detect and moderate radical content, extremist ideologies, and hate speech, employing text and image analysis. This tool aids law enforcement agencies, internet providers, and social media platforms in identifying and addressing radicalization threats effectively.
“Unlike tools that only focus on one form of extremism, CounteR detects content across a variety of radicalization domains, including jihadism and extremist ideologies. This ensures a comprehensive and holistic approach to monitoring and combating radical content online. The Platform is definitely not the only project result. The multidisciplinary team of CounteR is also proud of the valuable research findings produced within the project that helped tremendously for understanding the psychological and sociological dynamics of radicalization”, CounteR’s project coordinator, Catalin Trufin from Assist Software, commented.
Representing our country within the CounteR consortium is Imagga Technologies, part of a network comprising 19 esteemed organizations across 11 member states.
"Data science specialists meticulously analyzed extensive datasets to uncover patterns and trends. Leveraging advanced algorithms, project experts developed sophisticated tools for detecting and predicting indicators of radicalization. Utilizing Social Network Analysis (SNA) methodologies, our experts facilitated the identification of radicalization hotspots, key nodes, and communities within these networks," stated Georgi Kostadinov from Imagga Technologies.
Building on the groundwork laid during the project, the consortium remains committed to harnessing its findings and further refining them into a practical, finalized product. Proactive measures are underway to secure additional funding, ensuring the sustained development of the CounteR solution and its progression to the next phase.
For more information on the project, follow CounteR on X and LinkedIn, visit the CounteR website, and explore the full set of project newsletters.
The CounteR project is funded through the European Union's Horizon 2020 program and is presented under call for proposals H2020-SU-SEC-2020 with project ID 101021607.
The Benefits of AI Image Custom Model Training
Training custom machine learning models have become a crucial and powerful aspect of processing, understanding, and monetizing visual data today. Based on Artificial Intelligence (AI), this approach allows the fast and effective categorization of massive amounts of images and videos according to the particular needs of a business.
In contrast to traditional image classification, custom models provide an important layer of flexibility and adapt to the specifics of your company and your industry. The newly trained models operate based on categories that you set — and can thus analyze and organize your image and video database in the best possible way for your particular case.
In a nutshell, with custom ai model training, your system is taught to recognize concepts from your visual data — the concepts you care about and that hold potential for your business development. You can set any type of category for the classification process, as long as the categories are clear and don’t overlap with each other. This makes you the architect of your visual data classification.
In the sections below, we’ll go over the basics about machine learning and image categorization, and we’ll delve into how custom ai model training based on machine learning actually works. Read on for the full details.
What Is AI Machine Learning?
Machine learning is a section of computer science and AI that has been gaining exponential popularity. The main focus is the employment of algorithms through which technology mimics the manner in which human beings learn. So, it means that technology can learn and get better at its job with time. Platforms based on machine learning have the capacity to expand their capabilities and knowledge in unprecedented ways — with precision and accuracy growing with every bit of new data processed.
In layman's terms, the machine learning term entails ‘learning by doing.’ In technical terms, machine learning algorithms are taught to classify data and make predictions based on statistical methods. This makes them a powerful tool for unraveling insights from data that would take years to process otherwise. Equipped with these in-depth insights, business leaders and managers can make well-informed decisions that drive business growth and development.
Some popular uses of machine learning that we are already experiencing — even in daily life — include recommendation engines (like the series and film suggestions you get from your streaming platform) and self-driving motor vehicles.
1. Machine Learning and Deep Learning
Going a step further in understanding ai machine learning, it’s good to present the term ‘deep learning’, too. Often, machine learning and deep learning are seen as identical, but in fact, deep learning is a sub-field of machine learning. It entails a different learning process and has been referred to as ‘scalable machine learning’ by Lex Fridman.
The main difference between the two is that deep learning doesn’t require labeled datasets to learn. It can process data in raw and unstructured form, too. This makes it more independent from human input — and able to process larger amounts of data. Traditional machine learning, on the other hand, needs more actions from humans, and, in particular, more structured sets of data for learning.
What Is AI Image Categorization?
Image categorization, sometimes also referred to as image classification, is powered by computer vision. It employs machine learning and image processing to sort images and videos by distributing them into categories, which are usually set in advance. It may as well be one of the most significant elements of digital image analysis today.
Image categorization is widely used in a number of fields. Most notably, it’s the basic tool for automating content moderation online. However, it has numerous other uses, such as database sorting, product discovery in the field of commerce and retail, and asset management in technology and cloud services, among many others.
In essence, the powerful business use of image categorization is that it allows you to gain control over huge image sets. The engine is taught to discern different categories through a set of local and global visual features. Once it learns them, it’s able to spot the precise category for a new visual that it processes.
To get an idea of how Imagga’s image categorization engine works, you can check out our Visual Categorization Demo. Our Image Categorization API boasts a couple of powerful features: it’s accurate in its classification; it’s scalable even for enterprises; it’s simple and adaptable for cloud, on-premise, or edge; and it’s customizable to the specific needs of your business.
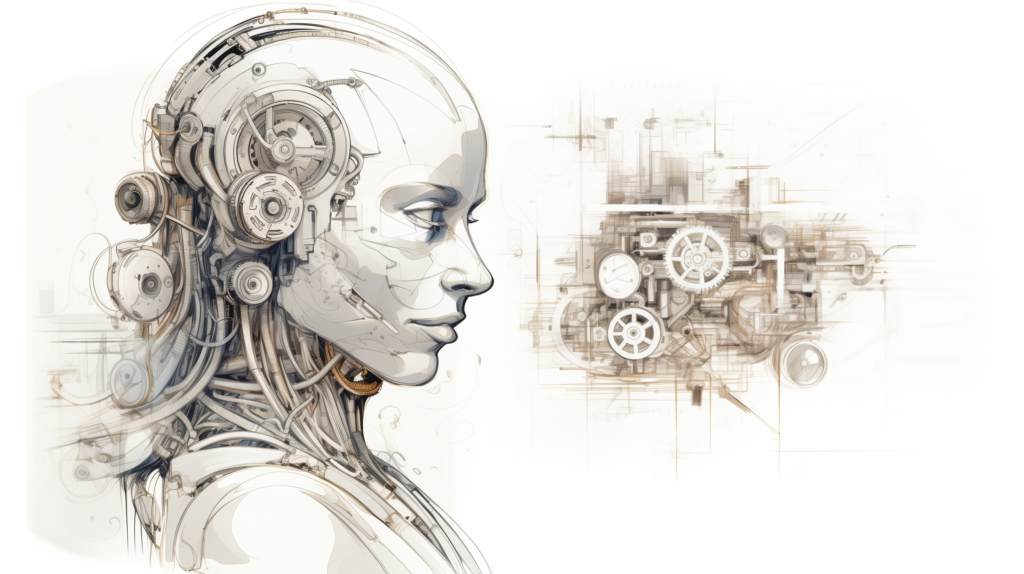
How Does AI Image Custom Machine Learning Model Training Work?
Creating custom models is at the heart of performing effective analysis of specific visual data that businesses need today. Pre-set categories don’t always satisfy these needs — and hence, customization becomes key.
With AI image custom model training, you can specify precisely the categories that your visual content has to be distributed to. The number of categories is limited, theoretically, but in practice, Imagga’s custom training can handle training with tens of thousands of categories.
The custom-trained model, tailored to the specifics of your business, can then be paired with the Image Categorization API, providing you with a powerful tool to classify visual data and maximize its use and impact.
1. AI Image Custom Machine Learning Model Training Steps
- Feeding with datasets: The training datasets have to be inserted into the engine, containing sample visual data for each category. The categories have to be non-overlapping and straightforward.
- AI Model training: Our machine learning experts build a deep learning classification model based on your data and the specific categories that you set. Content that has already been classified is used for the training so that the engine gets accurate principles for categorization. Afterwards, it can process new content and automatically classify it according to the newly created categories.
- Deployment in your systems: The newly trained model is embedded in an API that is seamlessly integrated with the systems and workflows that your business is already using. Through a customer demo, you can evaluate the model in advance.
PlantSnap Case Study: Image Categorization and Custom Training at Work
A great example of the powerful combination between Imagga’s Image Categorization API and Custom Training is the case study of our work for PlantSnap.
PlantSnap is an app that helps people identify any plant anywhere on the planet. It’s an amazing knowledge base where you can find all kinds of flora species. You just take a photo of the plant you want to identify — and the app provides you with its name and information about it.
To power up the plant recognition, PlantSnap needed an image categorizer that could handle a massive amount of categories — as there are 320,000 different species worldwide. Most image recognition providers couldn’t address this need, as they couldn’t train such a huge amount of categories, and couldn’t guarantee accuracy decreasing due to the large volume.
At Imagga, we decided we were up to the challenge. We invested in getting the DGX Station from NVIDIA, powerful hardware that we paired with our outstanding computer vision technology. The result is all that we expected it to be.
The custom model that we built for PlantSnap is ten times faster in training and doesn’t compromise accuracy. It’s combined with our state-of-the-art image categorization API that boosts high accuracy rates.
In the deployment process, we successfully resolved another challenge: plant look-alikes. Even plants with similar visual characteristics can now be identified and discerned by Imagga’s custom-trained model.
As a result of our efforts, Imagga is now a core technology in the PlantSnap app — boosting accurate plant recognition for all types of flora species worldwide: 320,000 plant classifications with a 90% precision rate for the top 5 results of each search.
Get Started with Your Image Custom AI Model Training
Due to our extensive experience in providing image recognition and categorization tools for companies from a wide variety of industries, we’re equipped to create powerful, tailor-made solutions.
Want to start building your custom-trained model for image classification? Get in touch to learn more about our solutions from Imagga’s experts.
Frequently Asked Questions
Custom training of image AI models relies on machine learning to train the engine to discern visual data from data assets and classify it into custom categories.
The process involves three steps. The first one includes providing existing datasets and concisely formulated, non-overlapping categories for their classification. Next, deep learning is used to build a classification model with the custom data, based on existing classified content. Then, the model is plugged into an easily embeddable API — which can start processing new visual data. The more images are analyzed and classified, the better the engine becomes at categorization.
Image custom training based on AI brings unseen advantages to businesses from a number of venues. Companies that operate with huge amounts of visual data, such as user-generated images and videos or crawled visual content, need effective ways to sort and arrange the data. Manual categorization and processing is unthinkable and overwhelming due to the massive quantities.
This makes machine learning algorithms indispensable help in this process. Image custom training, in particular, offers tailor-made solutions for the specific needs of a company. Instead of using pre-set categories for image classification, it allows a business to take control of its visual datasets by providing it with a deep-learning classification model that is trained to work with its specific categories and data. The deployment of AI image custom training is robust and can work with any dataset size, while also being flexible and adaptable to cloud or on-premise solutions.
Imagga Technologies Awarded Prestigious €2.1M Grant Funding for AI-Driven Content Moderation Platform Development
Imagga Technologies is excited to announce its receipt of a pivotal grant under the "Support for Innovative Enterprises awarded with 'Seal of Excellence'" program, a constituent of Bulgaria’s Recovery and Resilience Plan. This esteemed endorsement empowers Imagga, a leader in image recognition and artificial intelligence solutions, to forge ahead with its innovative AI-Mode project.
The AI-Mode project aims to create a state-of-the-art Content Moderation Platform, designed to revolutionize how companies approach and automate their content moderation workflows. By harnessing advanced AI capabilities, the platform will facilitate proficient image, video, and live streaming moderation, providing an indispensable tool in maintaining the sanctity of user-generated content (UGC) platforms and safeguarding brand integrity.
The Imagga Content Moderation Platform is a full-circle content moderation solution combining the best of artificial and human intelligence. This robust system is proficient in identifying and filtering a broad spectrum of inappropriate content, including explicit imagery, offensive symbols, regulated substances, violence, and propaganda, thereby reinforcing a brand’s digital ecosystem. The innovative use of machine learning significantly amplifies efficiency, potentially reducing the volume of concerning content by a factor of twenty through proactive AI-assisted filtering and iterative refinement from human moderators.
This development project originates from Imagga’s successful application and subsequent distinction under the European Innovation Council (EIC) Accelerator program, which has been operational since 2018. The EIC Accelerator is a cornerstone of the European Union's commitment to fostering innovation, offering cutting-edge enterprises the chance to compete for up to €2.5 million in grant financing, complemented by an opportunity for an equity investment of up to €15 million. Despite the rigorous competition with over 75,000 applicants, Imagga has emerged as a standard-bearer of innovation.
The Seal of Excellence is awarded to project proposals submitted under a Horizon Europe call for proposals and ranked above predefined quality thresholds but not funded by Horizon Europe due to budgetary constraints.
The Seal of Excellence is a quality label first introduced during Horizon 2020, the EU’s research and innovation framework program (2014-2020). It has gradually become a key instrument in recognizing innovation on EU level, which is later on funded by local government grant schemes.
So far, only 12 Bulgarian companies have received SoE, and the funding will come as a grant under the fourth measure of Bulgaria’s Recovery and Resilience Plan - "Support for Innovative Enterprises awarded with "Seal of Excellence" and co-financed by NextGenerationEU. It’s worth mentioning all applicant projects are assessed by four independent remote assessors (among 1,500 professionals authorized by the EIC after assessment of relevant expertise). Their assessments are combined in a general report with a maximum integral assessment of up to 15 points. Companies with a score of more than 13 points are awarded the 'Seal of Excellence'.
Fuelled by the financial stimulus of the AI-Mode grant, Imagga is committed to developing a sophisticated Content Moderation Platform that aligns with stringent EU content regulation standards and pioneers real-time moderation capabilities. This development underscores Imagga's commitment to fostering a safer, more respectful digital space, fortifying its position at the forefront of AI-driven technological solutions.
This publication was created with the financial support of the European Union - NextGenerationEU. All responsibility for the document’s content rests with Imagga Technologies OOD. Under no circumstances can it be assumed that this document reflects the official opinion of the European Union and the Bulgarian Ministry of Innovation and Growth.
CounteR Technical Meeting in Sofia
At Imagga, we were proud to co-host CounteR's fifth technical meeting in Sofia alongside the European Institute Foundation. For three days (September 26-28, 2023), all consortium partners reviewed the progress of the CounteR Project and further tested and validated the software tool in collaboration with our law enforcement partners. We took a deep dive into evaluating our past and current efforts and strategized for the upcoming tasks within our various work packages (WPs).
Our kick-off day was particularly illuminating, with an insightful review of WP2 – "Social and Psychological Factors in the Radicalisation Process.” We noticed an interesting paradigm shift in the research approach towards preventing radicalization. Instead of solely focusing on individual radicalized identities, the emphasis is gradually leaning towards understanding the underlying issues of radicalization hubs. Our discussions extended to WPs 3 to 6, where we explored enhancements in data collectors, image processing, social network analysis, and the more technical facets like semantic reasoning, deep reinforcement learning, and network algorithms for illicit content removal.
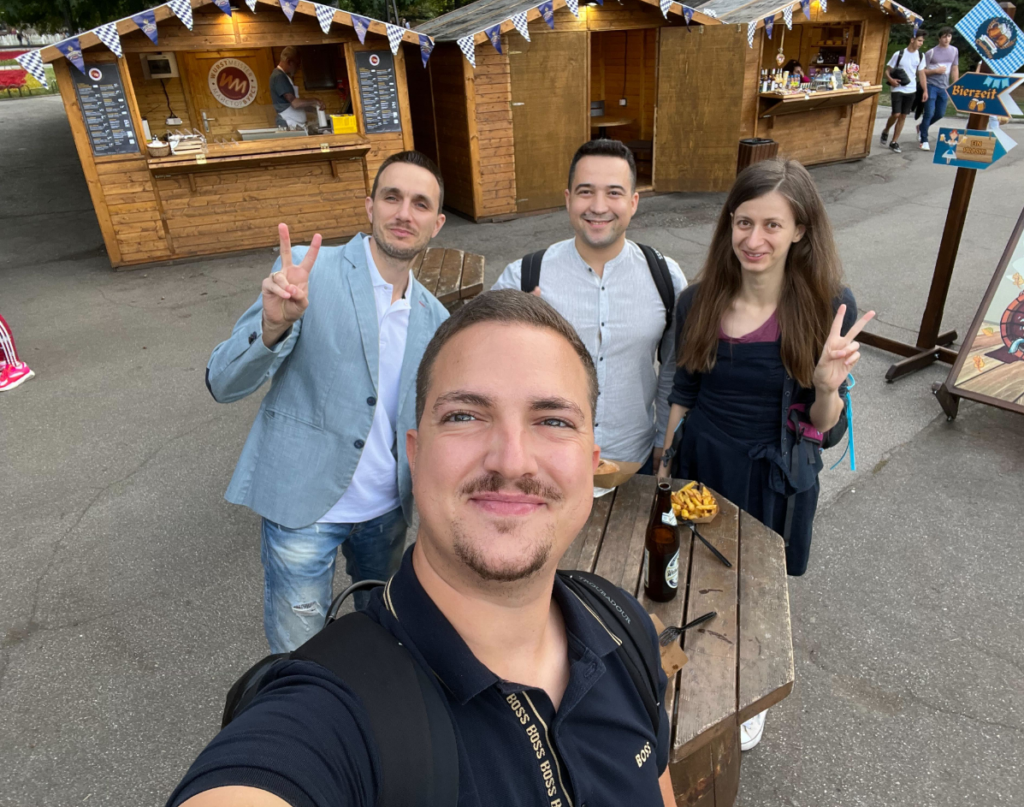
Day Two saw us delving into the intricacies of WPs 7 to 9, emphasizing data privacy and ethical standards. We reflected on the insights gained from our first pilot in Milan in June 2023 and used those learnings to refine our approach for the imminent second piloting session.
The culmination, Day Three, was primarily hands-on. Through various test scenarios and datasets, the tool's efficacy was assessed. A successful stress test was performed after the law enforcement representatives uploaded the synthetic datasets.
A significant portion of the testing was centered on the NLP analysis module, an excellent work by INRIA. Collaborative discussions between Insikt and us at Imagga enriched the process, especially concerning report issuance for image analysis. This was pivotal in enhancing the system's subsequent versions. Insikt and Imagga discussed the appropriate ways to issue reports for image analysis to have better and richer user feedback.
The culmination of these sessions wasn't just progress checks. We robustly tested our system alongside our law enforcement agency partners, gearing up for its grand release.
Furthermore, we dedicated a reasonable amount of time in Sofia to deliberate on CounteR's communication strategies and commercialization prospects. By the project's 36th month, we aim to launch a comprehensive business strategy to promote the CounteR solution. The knowledge amassed from CounteR isn't just technological; it spans across social, legal, and policy sectors – all of which are pivotal for our future roadmap.
A Day of Innovation and Insights: Imagga at the AI4Media Workshop in Amsterdam
Amsterdam was abuzz with action and innovation, and we at Imagga were right at the heart of it!
As part of the transformative AI4Media project, the Speculative Design Workshop was convened, and Georgi Konstantinov, CTO of Imagga, had the honor of representing us at this prestigious event. Our mission? To showcase Imagga’s cutting-edge solutions for content moderation and organization. We explored current uses of our technology and ventured into the tantalizing possibilities of what the future might hold.
But the real magic of the workshop was how it became a melting pot of analytical reasoning and out-of-the-box creativity. A lot of this was thanks to the unparalleled skills of our facilitator, Nina Pavlovska from ZEZA. Under her guidance, a cascade of innovative ideas emerged, promising to shape the direction of the AI4Media project in ways we had only dreamed of.
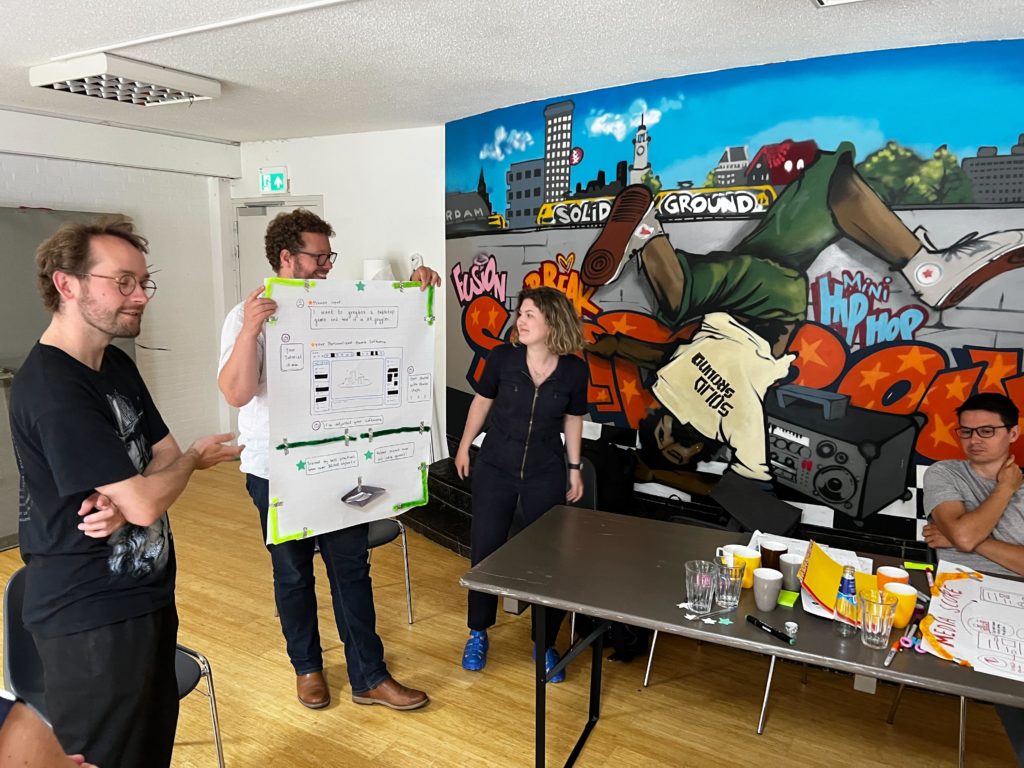
But the excitement didn’t end there! As fortune would have it, the workshop’s timing overlapped with the Mozilla Festival Amsterdam. This serendipitous occurrence allowed us a golden opportunity to test and validate our freshly minted concepts with a broader audience.
In a surprising twist to an already exhilarating day, Georgi stepped onto the stage for ‘The People Speak’ – a live talk show. Representing our work at Kelvin Health, he shared insights into AI’s pivotal role in healthcare. The conversation was both enlightening and humbling, emphasizing AI’s vast potential and responsibility in shaping our healthcare systems.
Reflecting on the day was a whirlwind, a marathon of thoughts, ideas, and interactions. But it also underscored the limitless horizons that lie ahead for AI, Imagga, and the larger digital community. Here’s to many more such days of discovery and growth!
The Evolution, Importance, and Challenges of Automated Media Organisation - White Paper
The modern digital age has ushered in revolutionary advancements in Machine Learning (ML) that have reshaped the media landscape. These advancements have significantly fueled the potential of automating extensive media collections, promising efficiency and precision like never before. A mere decade ago, the ambit of image recognition was limited to basic shapes and objects. Contrast that with today, where our image recognition AI allows instant recognition of a plethora of visual content.
However, despite these impressive strides, the journey of AI and ML in media isn’t without its stumbling blocks. Imagga’s eye-opening survey indicated that only 7% of media companies leverage automated solutions for visual content organisation. The majority still rely on the age-old manual cataloging methods, essentially anchoring themselves to human limitations.
Addressing Industrial Needs and Overcoming Technological Hurdles
Every media entity, regardless of size, grapples with the daunting task of efficiently organising the sheer volume of visual content it generates or receives. This content could range from in-house photo and video collections to user-generated content (UGC), which has exploded in popularity and scale in recent years.
Traditionally, media houses have resorted to manual tagging and categorization. However, such practices have proven to be sub-optimal, especially in a world where speed and accuracy are paramount. A paradigm shift to automated AI-powered tools that promise optimal organisation is obviously needed for media companies to face the challenges and adapt to new realities.
Yet, the path to automation is lined with challenges. Introducing such advanced tools demands hefty costs, a deep pool of AI expertise, and the crucial need to manage expectations. It’s vital for the non-technical staff in media companies to possess a grounded understanding of AI’s capabilities and limitations. Unrealistic expectations can hinder progress and inflate investment without yielding the desired results.
Unlocking Value from Existing Content
Amidst the clamor for the new, there’s a treasure trove lying in the old. Media entities sit on vast reserves of content that, with the right tools, can be repurposed, opening up new avenues of value and revenue. However, the real challenge lies in making this content easily discoverable.
Imagine a scenario where content that was created for one specific use-case can be easily accessed, modified, and reused for another. This ensures cost efficiency and maximizes the return on content investment. The magic key that unlocks this potential? Proper metadata and archiving practices that are bolstered by AI.
Looking Forward: Adapting to the New Era
Much like any other, the media industry isn’t isolated from disruption. With the exponential rise of user-generated content and citizen journalism, traditional revenue streams are being constantly challenged. This disruption has made it imperative for media companies to adapt and realign their strategies.
Media entities must rethink their content creation models in a world where every individual with a high-quality camera phone can be a content creator. This is where AI-enhanced tools step in, serving as the bridge to the future and helping media entities remain competitive, relevant, and efficient.
The Path Ahead: AI for Media
The marriage of AI and media promises a future brimming with potential. But to harness this potential to its fullest, there’s a need for a new, AI-based approach. Media entities must embrace technological upgrades, invest in staff training, and constantly re-evaluate and evolve their existing workflows.
As we stand on the cusp of this transformation, it’s essential for AI developers and media companies to foster a spirit of collaboration. This mutual partnership will ensure that technological capabilities align perfectly with the industry’s ever-evolving needs, ushering in a new era of efficient, innovative, and forward-thinking media.
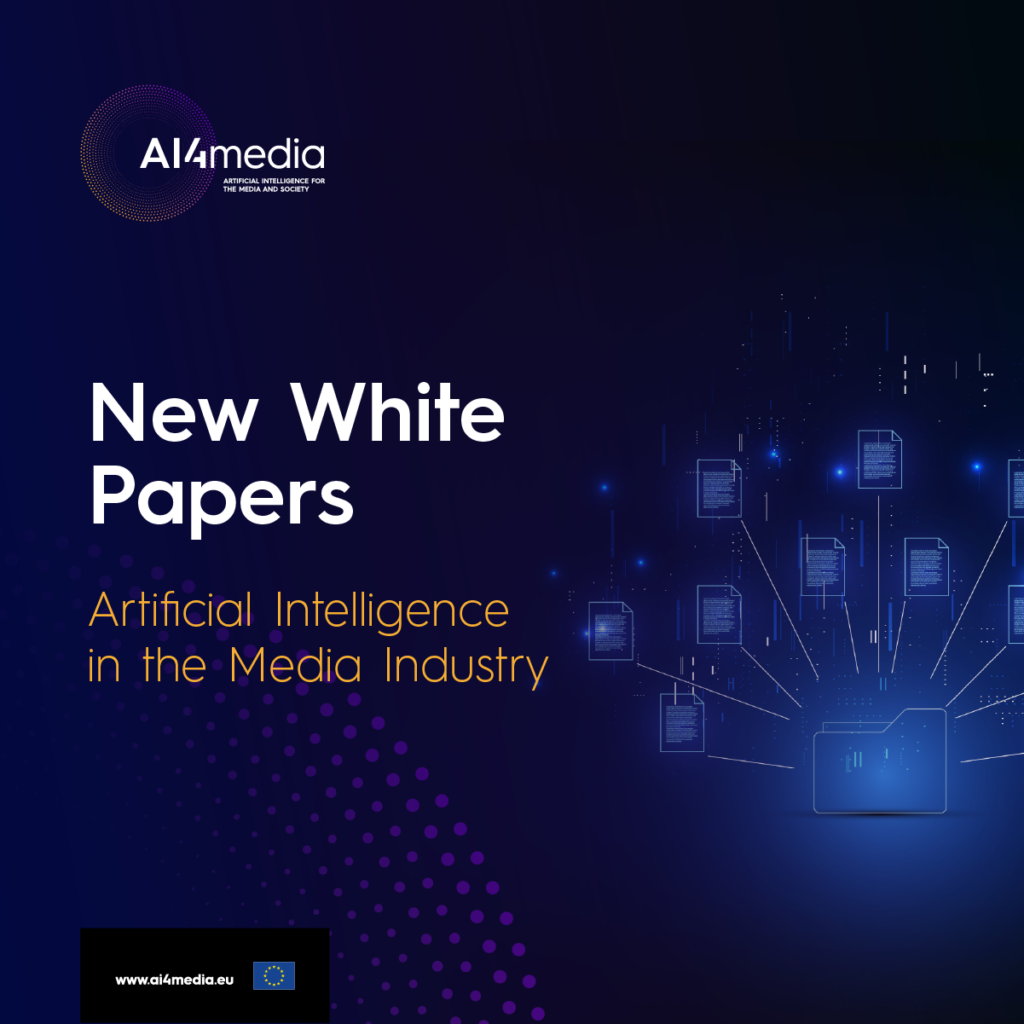
Download the latest Imagga White Paper on AI Technology for Image and Video Organization
Imagga’s white paper is sponsored by AI4Media, a Horizon 2020 project, building an AI network for organisations from academia and industry that embraces a vibrant ecosystem focused on AI for media and society and enables the quick market uptake of technologies and research/business collaboration opportunities. A series of white papers are published in an effort to align AI research with the industrial needs of media companies. These white papers describe the most critical challenges and requirements for AI uptake in different media sectors.
Imagga Attended CounteR’s Autumn Technical Meeting in Malta
Imagga took part in CounteR Project’s third technical meeting in Valetta/Malta on September 28-29, 2022. The meeting brings together representatives of the consortium partners of the CounteR Project.
Georgi Kostadinov, CTO of Imagga, together with representatives of ELTE University and Insikt Intelligence, presented the progress on WP4/Data Analytics for Detecting Radical Content‘s data understanding and preparation, the basic features and transfer learning regarding the NLP analysis, and image and social networks analyses.
The partners are reviewing the status of the work with a focus on current tasks. The participants are also planning ahead and discussing the upcoming project deliverables to be submitted by Month 18.
The event is a perfect opportunity for all consortium partners to meet, update each other on the project's progress, exchange ideas, get inspired, and create business opportunities.
The CounteR consortium brings together an illustrious group of international subject-matter experts in counterterrorism, radicalisation, and privacy law, six European law enforcement agencies with practical in-field knowledge, as well as a group of technical SMEs and academic partners.