The practical applications of Artificial Intelligence in our world today are ubiquitous, and image recognition in waste management is one of the curious cases in which new technology can bring immense positive changes.
Image recognition powered by AI is, in fact, already being explored as a tool for effective waste management. Many prototypes have been developed around the world, and some are gradually being deployed in practice. Waste detection and separation with the help of image recognition is still an activity driven by scientific researchers and remains yet to become a massive practice.
Let’s review how image recognition can assist in revolutionizing waste detection and sorting to achieve Zero Waste — helping humanity deal with the negative impacts of trash by turning it into usable resources that drive Industry 4.0.
Contents
The Global Issues in Waste Management Today
Exponentially increasing amounts of trash, pollution, risk of diseases, and economic losses — the aftereffects of improper waste management are numerous.
Despite technological advancements across the board, handling waste is one of the major issues in our modern world. According to the UNEP Global Waste Management Outlook 2024 report, municipal solid waste is bound to grow from 2.3 billion tonnes in 2023 to 3.8 billion tonnes by 2050. The global waste management cost in 2020 was $252 billion, but when taking into account the costs of pollution, poor health, and climate change, it’s estimated at $361 billion. The projected costs for 2050 are $640.3 billion unless waste management is rapidly revolutionized. All of this affects people on the whole planet, and as usual, its adverse effects are bigger in poorer areas.
Finding effective waste management solutions is important on a number of levels. Naturally, the issue of protecting people’s health is primary, as well as nature preservation — especially given the major problem with plastic waste that is cluttering our planet’s oceans. Beyond handling pollution and health issues, proper waste handling can also lead to adequate recycling and reusing of resources, as well as bring direct economic benefit to our societies. The UNEP Global Waste Management Outlook 2024 report points out that applying a circular economy model containing waste avoidance and sustainable business practices, as well as a complete waste management approach, can lead to a financial gain of USD 108.5 billion per year.
While there are advanced waste management systems around the world, a practical and affordable solution that can be applied in different locations across the globe has not yet been discovered. This is especially relevant for the major task of trash separation of biological, plastic, glass, metal, paper, and other materials for recycling, as well as for dangerous waste detection that safeguards people’s health. This is where image recognition powered by Artificial Intelligence can step in.
Image Recognition for Waste Detection and Sorting
The applications of Artificial Intelligence in waste management are growing, and the main approach that fuels this growth is the use of image recognition based on machine learning algorithms. With the help of convolutional neural networks (CNNs), waste management tools can find patterns in the analyzed visual data of trash, propelling the development of intelligent waste identification and recycling (IWIR) systems.
Use of Image Recognition in Waste Management
The main tasks of image recognition in waste management are identification of recyclable materials, waste classification, detection of toxic waste, and trash pollution detection. In addition, image recognition solutions for waste management have to offer a high degree of customization and flexibility to tailor to the difference between regions. For example, fine-tuning for different areas with specific trash images is essential. Training the algorithms with waste items particular to the specific demographic region is crucial for effective classification.
The goal of waste management systems powered by image recognition is to be able to identify and classify different types of trash. They are being trained with large image datasets, so that they can afterwards predict and spot hazardous trash and differentiate between different types of recyclable materials. In some cases, prototypes are made with robotic platforms that use sensors and microcontroller boards for accurate detection and processing, while in others, mobile phone terminals are being used.
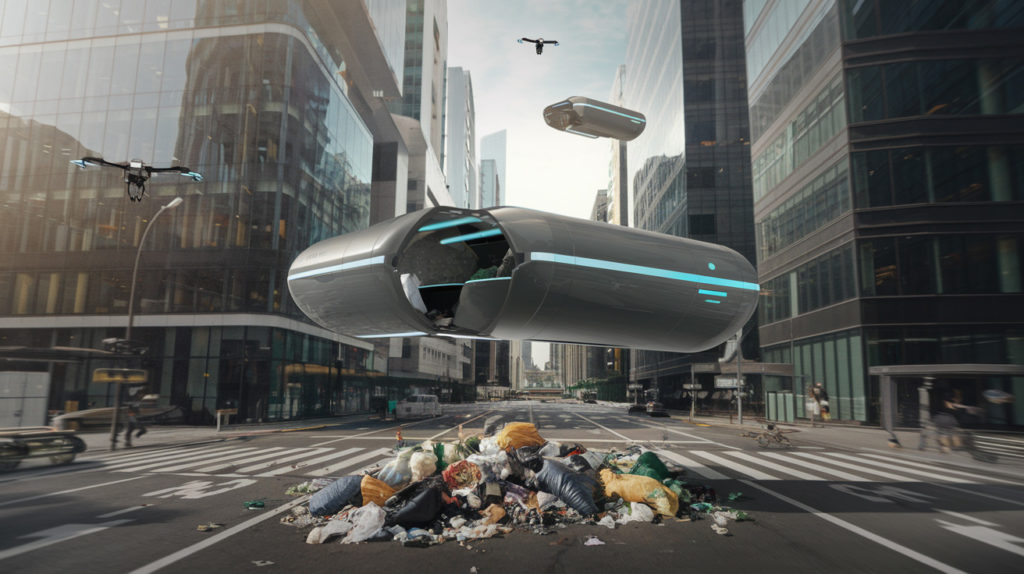
The Future Possibilities and Benefits
The use of image recognition in waste management, while developing rapidly, is yet to become widespread, but the possibilities and benefits it offers are highly promising.
The automatization of recyclable material sorting can have an immense impact on environmental sustainability, helping resource conservation and reduction of greenhouse gas emissions due to new production. It can increase the identification and use of valuable materials in trash, as well as reduce overall waste.
The shift in waste management with image recognition can lead to meaningful changes in waste management jobs. Image classification technology would save people from doing dangerous and dirty jobs, while ast the same time creating new opportunities in technology and data analysis.
Awareness about recycling and greater access to recycling services can grow as a result of image recognition-powered waste management systems. If recycling is more efficient and widespread, even communities with limited resources would get access to recycling options, as well as information about the benefits of recycling practices in daily life.
Use Case: Image Recognition in Waste Management
At Imagga, we do our best to push the boundaries of what’s possible with image recognition — and waste management is an area that we’ve already been exploring.
Already in 2016, we had a pilot trial with the Seoul National University, the most prestigious South Korean research university, on a project for waste management with image recognition capabilities. A team from a couple of departments at the University, led by Prof. Seong-Woo Kim and Dr. Daeun Kim, started the pilot project Fun with Trash to create a system that makes trash recycling easy and effective.
The prototype built by the team relied on a smart sensor system to identify the different types of recyclable trash that are being deposited in the different recycle bins (plastic, paper, glass, and metal). Once a person is about to throw a piece of trash, a camera sensor captures an image, and then the system recognizes the type of trash and compares it to a database of images. Then, the right bin is opened to deposit the trash.
Imagga jumped on board to help the SNU team with the image recognition technology to power up the recycling machine prototype. Our team trained the custom classifier for waste categorization and helped with improving its accuracy, reaching precision rates of 95%. Our experts also advised on the sensor positioning and illumination of the hardware setup.
Learn How Image Recognition Can Power Up Your Waste Management System
Imagga’s robust image recognition platform holds a huge potential for waste management systems due to our custom model training. In the context of waste management, custom model training is essential to provide the necessary flexibility to work in different demographic areas.
Our customizable tool allows you to define the categories in which you want to classify visuals so that the classification system is tailored to your particular needs. First, specific datasets are selected, and then we build a deep learning classification model trained with the particular categories and data. Afterward, our image categorization API does the rest of the job by fully automating the image categorization process.
Want to learn more about how image recognition can fuel the efficiency of waste management systems? Get in touch with us.