Generative AI Fashion Imagery: Transforming the Future of the Industry
Fashion is evolving, and the next revolution is happening digitally, aka Generative AI Fashion . Generative AI is now capable of producing photorealistic fashion imagery, rivaling traditional photography. But that’s just the beginning—AI is pushing the boundaries further with virtual human models and virtual try-ons, personalized for each shopper. In this post, we explore where fashion imagery stands today, between being fully created through traditional photography and a future where AI-generated visuals make up a significant portion of the content.
International Creative Director Federico Donelli writes in his article on the current state of AI in fashion imaging that the primary criterion for evaluating AI's ability to rival traditional fashion photography is photorealism. With the introduction of Midjourney V.6, he says, the line between AI-generated and traditional photography has become increasingly difficult to discern. By applying the same methodical approach he uses in directing photo shoots to craft prompts for AI-generated images, Donelli achieves impressive results. He notes that the distinction between AI and traditional photography is now remarkably subtle.
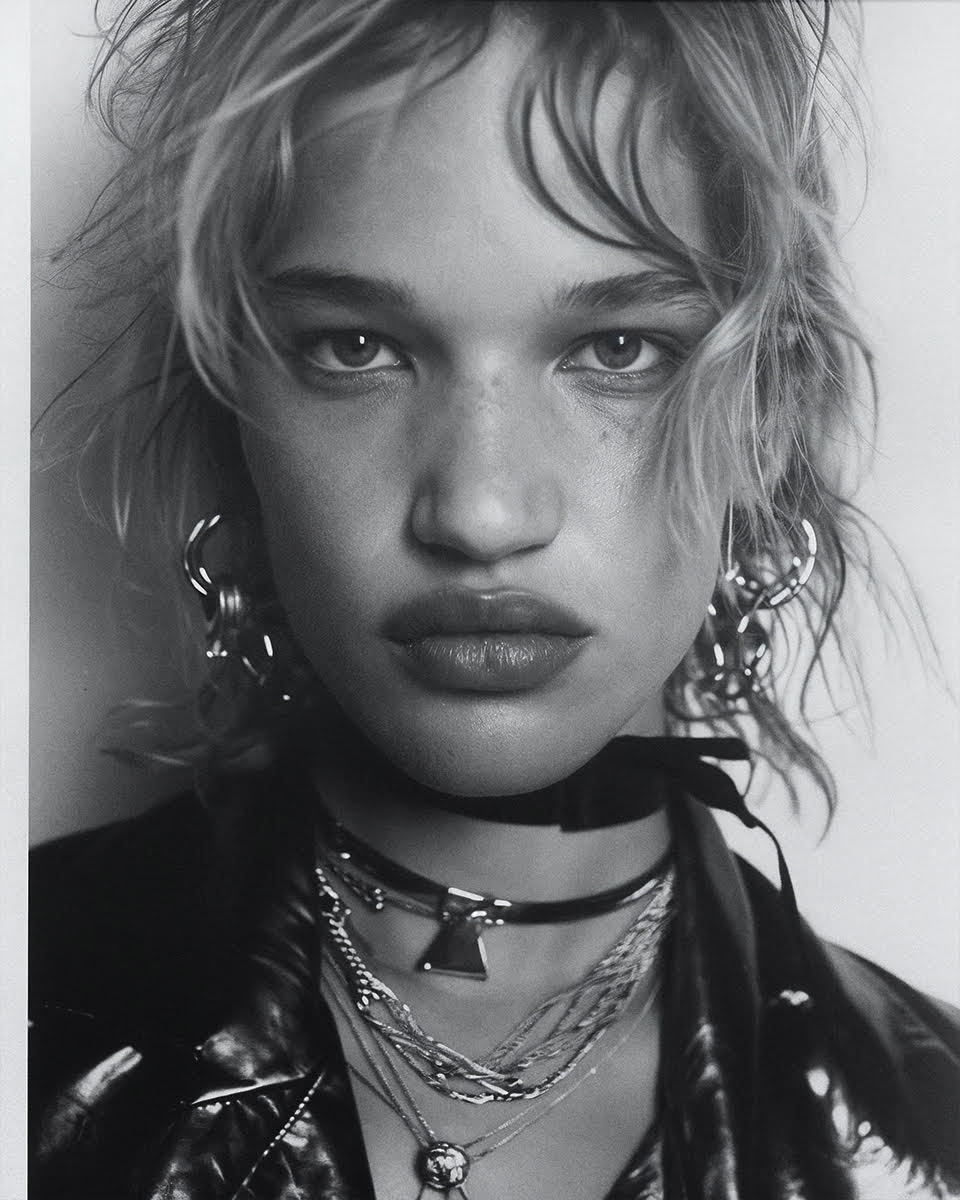
In his detailed analysis of other key aspects of fashion photography—such as framing, camera focus, angles, styling, lighting, hair and makeup, setting, and casting—Donelli concludes that the technology is now largely ready for commercial use, especially when paired with skilled post-production and retouching.
The Challenge: Garment Replication via Generative AI
One of the major challenges in applying AI to fashion imaging, as Donelli highlights, is garment replication—the ability to dress subjects in virtual garments that precisely replicate the look and feel of real clothing.
This is an area where our engineering team has recently made significant strides, achieving impressive results.
Generative AI Fashion Virtual Models
Imagga engineers have successfully transformed images of clothes on plastic mannequins into stunning visuals featuring AI-generated human models.
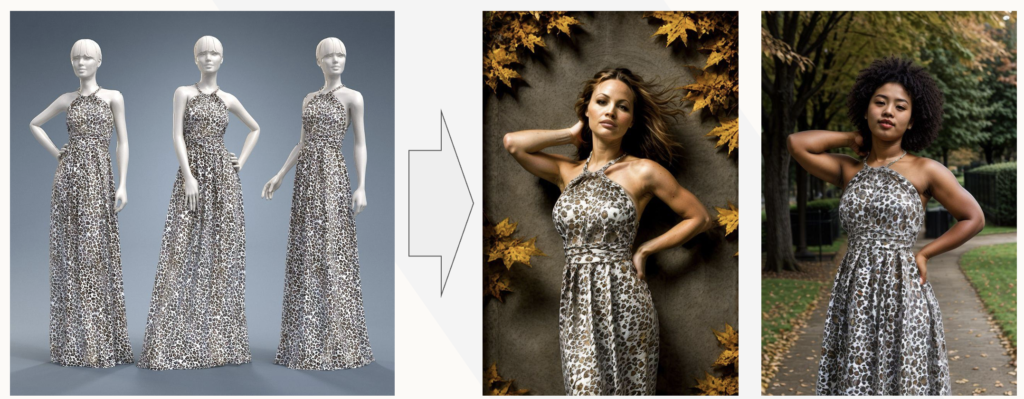
They’ve developed a model that not only showcases how a given dress looks on women of different body shapes and sizes but also how various design variations of the dress appear.
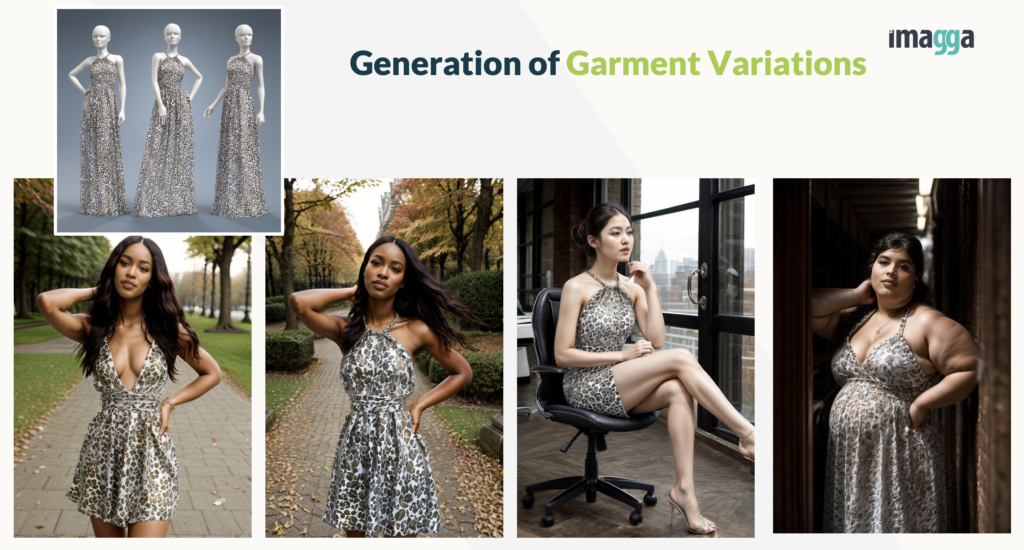
They have even demonstrated how the same dress looks on women of similar body shapes but from different races and age groups.
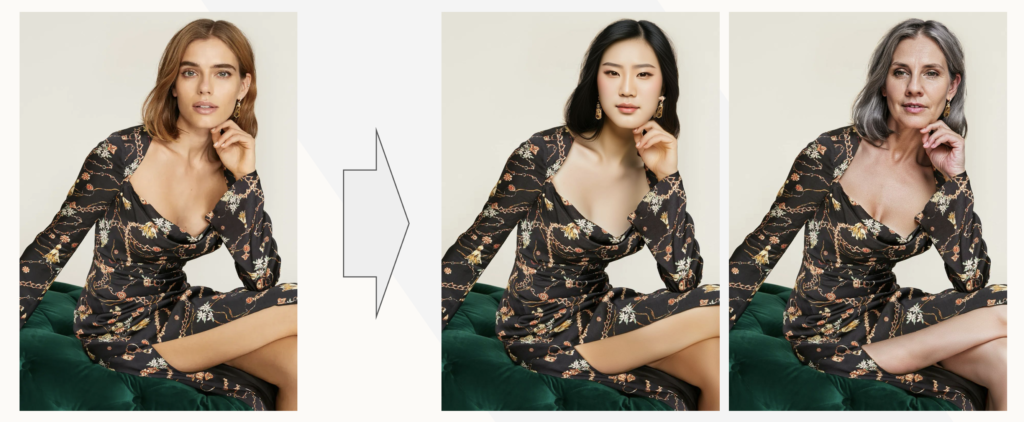
Virtual Try-On
A virtual try-on lets users visualize how clothing would look on them without trying it on physically. It is difficult to build because it requires accurate body simulation, accounting for diverse shapes, sizes, and fabric movement.
Our engineering team has made significant advances with our virtual try-on functionality, which will allow users to upload a photo and see the exact fit on their body, offering a highly personalized and delightful experience.
For online fashion retailers, where user experience is a key differentiator, our virtual try-on has the potential to significantly enhance engagement and conversion rates. By allowing customers to see exactly how clothes will fit using their own photos, it creates a more immersive and personalized shopping experience.
The virtual try-on functionality enhances confidence in online purchases by bridging the gap between virtual browsing and real-life fitting rooms.
![]() |
![]() |
![]() |
![]() |
The Technology Behind the Generative AI Fashion
Virtual human models are powered by Low-Rank Adaptation Models or LoRA models. These are relatively small machine learning models designed to efficiently adapt and learn from new data. Training LoRA models is the next step toward achieving precision in photorealistic fashion imagery. Image processing algorithms further enhance photo quality by correcting imperfections like glare and color discrepancies.
Implementing generative AI, image processing, and image recognition technologies is no easy task. Here are some of the key challenges:
Skin Tone Accuracy
Accurate representation of skin tone is critical to creating realistic virtual models. Generative AI must be trained on diverse datasets to capture a wide range of skin tones without introducing bias. This requires high-quality data and advanced color calibration techniques to ensure virtual models appear natural under various lighting conditions and on different displays.
Body Posture and Movement
Simulating realistic body postures and movements is particularly challenging. Virtual models must interact with clothing naturally, meaning the AI must understand and accurately replicate fabric dynamics and how they drape over different body shapes. This task demands complex algorithms that predict cloth movement, requiring extensive computational resources and advanced modeling techniques.
Finger and Hand Positioning
Modeling hands and fingers accurately is difficult due to their intricate movements and interactions with objects. Fingers require detailed articulation to avoid unnatural poses that can detract from the overall realism of the virtual model.
Seamless Interaction with Apparel
For a realistic appearance, clothing must conform naturally to the virtual model's body shape. This involves accurately simulating how fabric interacts with body contours, a particularly challenging task with complex garments, such as layered outfits or those made from multiple materials.
Environmental Factors
Achieving photorealism in Generative AI Fashion imagery requires accurate simulation of environmental factors, such as lighting and background elements. Whether it's ambient or direct lighting, these aspects play a crucial role in how clothing appears on virtual models. Properly replicating light sources ensures the images feel natural and realistic. Similarly, background objects need to be rendered in harmony with the scene to avoid distractions and enhance the overall authenticity of the visual.
Scalability and Speed
While creating a few high-quality virtual models is achievable, scaling the process to accommodate thousands of products across various poses and settings is daunting. Ensuring this process remains time- and cost-effective without sacrificing quality is a critical challenge for commercial applications.
The future of Generative AI Fashion
Generative AI is transforming fashion imagery by creating photorealistic images which are virtually indistinguishable from those captured through conventional photography, creating lifelike virtual models and making content production faster and easier. However, there are still big challenges related to garment replication, seamless interaction of models with garments, and scaling the technology for widespread use.
With our deep expertise in image recognition and custom visual model training, combined with ongoing R&D, we’ve developed solutions to help clients bring their ideas to life through advanced AI technologies.
If you’re exploring how AI can enhance your fashion imagery, reach out!
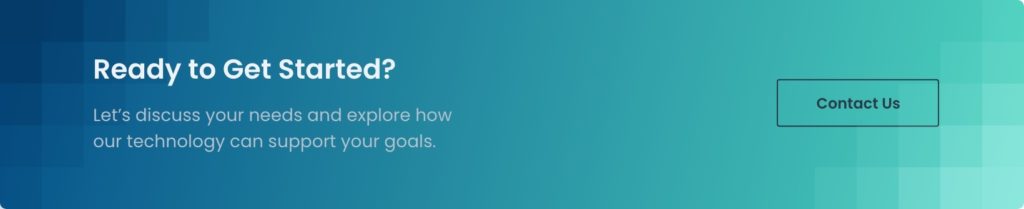
The Myth of Cheap Visual AI: Revealing the True Cost of Advanced Solutions
Artificial Intelligence often gets a reputation for being a cheap, magical solution. AI services like ChatGPT have revolutionized how people interact with technology. For a small subscription fee, anyone can access a powerful language model that can generate text, visual and code. This has led many to believe in the the myth of cheap visual AI - all AI applications share this affordability and ease of use.
General-purpose visual AI models are also budget-friendly and have fueled this belief. They efficiently organize and manage large volumes of images and videos. These models excel when your requirements closely align with their training data; however, their effectiveness diminishes with the specificity of the task such as detecting manufacturing defects or recognizing different types of waste.
The leap from general-purpose models to custom visual AI causes a significant increase in complexity and cost, a reality often overshadowed by the allure of inexpensive AI solutions. In this post, we explore the intricate world of custom visual models designed for complex use cases, shedding light on why they command a higher price tag.
Custom Visual Models: Precision Comes at a Cost
Consider an online fashion retailer that enables customers to upload images of garments they like and search for similar items in its inventory. This capability relies on a finely tuned object detection model and a visual search model, both specialized for this specific use case. Achieving this precision necessitates a comprehensive dataset, including a diverse array of images and detailed attributes like object size, lighting conditions, and background variations. Each nuance adds layers of complexity—and cost—to the models’ development.
The Expertise Required
Developing custom visual AI models is not just a technical challenge; it requires a blend of deep technical know-how and business acumen. The development process is intricate, demanding a multidisciplinary team proficient in computer vision and machine learning, augmented by domain-specific expertise. Moreover, ensuring the ethical use of AI, adherence to data protection laws such as GDPR, and the mitigation of biases is paramount, adding further to the complexity.
The Importance of Quality Data
Data is the cornerstone of any AI model. Custom visual AI models require large quantities of high-quality data that accurately represent the tasks they’re designed for. Often, clients don’t have the necessary data on hand. While this isn’t a dealbreaker for developing the model, it does contribute to rising costs. Data can be collected and annotated in several ways. For example, our partners at Humans in the Loop can assist in data collection and annotation. Additionally, existing datasets can be enriched with synthetic data generation, though this also increases costs.
Is a Custom Visual AI Model Worth the Investment?
Deciding whether to invest in a custom model should start with identifying specific business challenges that visual AI could address. Evaluate whether existing tools are sufficient or if a custom solution could provide a competitive edge. Consider the availability and quality of the data needed to train the model—robust, well-annotated datasets are crucial.
Investing in a custom visual AI solution can be transformative if it uniquely enhances your operations or solves a problem unaddressable by generic models. By understanding the complexities and costs involved, businesses can make informed decisions about harnessing the power of custom visual AI.
Conclusion: Beyond the Hype of Cheap AI
The narrative that AI is universally cheap and easy diminishes the true value and complexity of tailor-made solutions. Custom visual AI models are not just software upgrades—they are strategic investments that require careful consideration and substantial resources. By demystifying the costs and processes involved, we can appreciate the profound capabilities and potential of these advanced technological tools.
Content Moderation for UGC Platforms Guide – Solutions, Infographic, Statistics
What is Content Moderation?
Content moderation is the process of screening and filtering any type of user-generated content (UGC) uploaded and shared online to determine whether it’s appropriate for publication based on predefined rules. The monitored content can be images, videos, audio, text, and livestream. While inappropriate content categories can vary from platform to platform based on the targeted users, there is content that is undoubtedly harmful and illegal and should be blocked no matter the website hosting them.
Billions of people have come online in the past decade, gaining unprecedented access to information. The ability to share their ideas and opinions with a wide audience has grown businesses, raised money for charity, and helped bring about political change. At the same time, the UGC economy has also made it easier to find and spread content that could contribute to harm, like terror propaganda, abuse, child nudity exposure, and others. The free access to content sharing online creates tons of “digital garbage”. Billions of users share text, image, video and audio content online on a daily basis. Some of it is inappropriate, insulting, obscene, or outright illegal. If left unsupervised, user-generated content may cause major harm to brands and vulnerable groups.
“The free access to content sharing online creates tons of digital garbage.”
Companies of various industries and sizes need content moderation as a shield against online bullies. Content Moderation can be performed by people, by AI-powered visual recognition algorithms or by hybrid moderation solutions combining humans and AI.
Why is Content Moderation So Hard?
The complexity of content moderation lies in the enormous volume of UGC and its exponential growth as existing platforms scale and new ones appear. Companies lack the processes and tools to handle the relentless pace at which content circulates on the web. Unlike the content volumes which grow exponentially, the content moderation teams do so at a very slow linear pace. Additionally, the content moderation job is excruciating and has detrimental emotional and mental effects on employees, causing people to quit and content moderation companies to close business.
The alternative solution, AI-powered visual recognition algorithms, while capable to process and filter enormous amounts of content with high precision, cannot deliver where cultural or other context is needed.
Additionally, the margin for error is intolerable. Even if a given platform does a great job filtering 99.99% of the inappropriate content and misses just 0.01%, the missed content (false negative) can do significant damage traumatizing the audience and hurting the company reputation; while the incorrectly blocked unrelated content (false positives) raises issues for censorship.
User Generated Content Statistics
Billions of visual, text, and audio content pieces uploaded daily online need to be screened and filtered. Let’s take a look at the number of photos1, videos and tweets shared on some of the biggest social media platforms:
Facebook – 300 million photos uploaded daily
Instagram – 95 million posts daily
Twitter – 140 million tweets daily
Snapchat – 3 billion daily snaps
Youtube – 300 hours of video are uploaded every minute
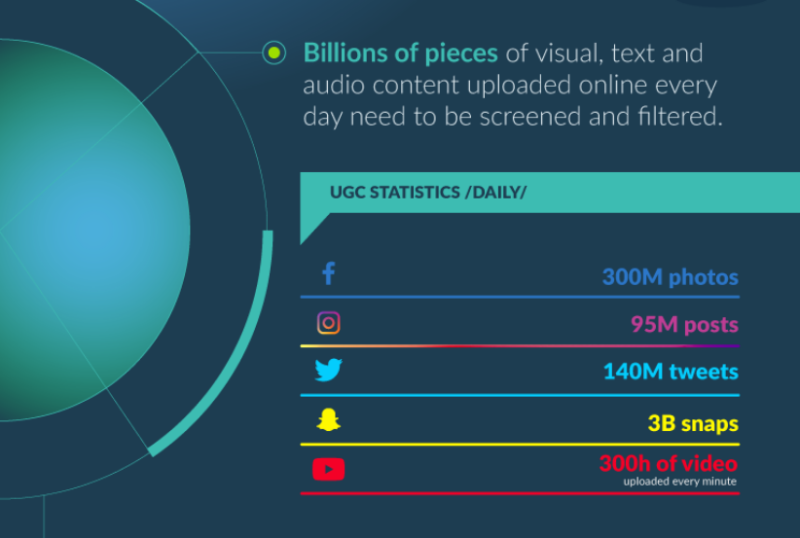
Who Needs a Content Moderation Solution?
The topic has become a top priority for the largest social platforms amid scandals related to human content moderators’ harsh work reality, escalating content-related crises and increased public and political concerns2.
Тhe reality is that any online platform that operates with UGC is facing the same problem. There will be closer scrutiny on how companies are moderating content as regulators around the globe are paying closer attention to the subject.
Here’s a list of online platform types that need a solution:
- Social media giants like Facebook, YouTube, LinkedIn, Instagram, Pinterest, Snapchat, Twitter
- Travel and accommodation platforms such as Airbnb and TripAdvisor
- Dating platforms such as Tinder and Grindr
- Entertainment social media such as 9Gag and 4chan
- Artwork communities like DeviantArt and Behance
- Mobile photos and video sharing platforms such as Flickr and 500px
- Stock photography websites such as Unsplash and Pixabay
- Crowdsourcing platforms such as KickStarter and Indiegogo
- Retailers and online marketplaces such as Ebay and Etsy
- Information sharing such as Reddit and Upworthy
- Blog hosting platforms such as Medium and Tumblr
- All blogs and websites that allow user comments in any form, text including
- Audio social networks such as SoundCloud
- Customer review websites such as Yelp
- All marketing campaigns relying on user-generated content
- All online communities and forum websites
Human Content Moderation
Because of the rise of social media, content moderation has become one of the largest and most secretive operational functions in the industry. An army of moderators all over the world screens through the Internet for violence, pornography, hate speech, and piles of inappropriate or illegal content.
There are two major problems with moderation done by people.
The first one is ethical. Evidence keeps piling up, showing that people exposed daily to disturbing and hurtful content undergo serious psychological harm3. Burnout and desensitization are only on the surface, followed by PTSD, social isolation, and depression, according to Sarah T. Roberts, author of Behind the Screen book4. The Verge published an investigation5 into the working conditions at Cognizant in Phoenix, a top content moderation company that later in the same year left that business6. Employees have been diagnosed with post-traumatic stress syndrome.
The other problem is related to productivity and scale but should not and cannot be examined in isolation of the ethical aspect.
- Manual moderation is too expensive and too delayed in time to support near-real-time and live streaming moderation.
- Content volumes grow exponentially while moderation teams do so at a very slow linear pace.
- It’s hard to train huge masses of people and change requirements dynamically, which makes it impossible to change policies or add overnight a new type of content that needs to be recognized
- People overlook things easier than A.I. If a specific piece of content has been flagged as inappropriate, the machine would never mistake it for appropriate again (unless explicitly corrected). This gives the opportunity to stop the spreading of inappropriate content - something that is a major problem for manual moderation
- There is a potential privacy issue when real people examine the content of other real people
“Unlike the content volumes which grow exponentially, the content moderation teams do so at a very slow linear pace.”
AI Content Moderation
AI–powered visual recognition algorithms, or AI content moderation, hold the promise of addressing both the ethical and economic side of the problem. The machine algorithm can do the heavy lifting, processing and categorizing a gigantic amount of data in real-time. It can be trained to recognize visuals in any harmful content category such as pornography, weapons & torture instruments, brawls & mass fights, infamous & vulgar symbols, horror & monstrous images, and many more and achieve an extremely high precision rate.
AI-powered visual recognition algorithms are exceptionally strong at spotting weapons in images and videos, detect nudity or partial nudity, detect infamous people, landmarks, and symbols.
One area where AI models fall short is the contextual understanding of the content and its distribution channels. A video showing an armed conflict between military and civilians might be broadcasted by a TV channel as an important piece of news. But the same video can be viewed as harmful and removed when shared by a user and accompanied by commentary applauding the violence outside of the context in which the violence is being used3.
A Hybrid Solution: Combining the Best of Artificial and Human Intelligence
Combining human judgment with artificial intelligence holds enormous potential for handling the tons of violent, pornographic, exploitative, and illegal content generated online daily. The algorithms, processing the majority of the content, and sending just a small fraction of it to human moderators, can significantly reduce the workload for hundreds of thousands of psychologically harming content moderator positions. Furthermore, it is vastly more productive, easier to scale, and less expensive for companies when most of the data is processed by AI.
As one of the pioneers in the image recognition space, the Imagga data science and engineering teams have deep expertise in developing AI solutions for visual analysis. Learn how Imagga technology is leveraging the best of artificial and human intelligence to handle every aspect of the moderation needs of companies of any size and industry.
Content Moderation Infographic

...
Sources:
1. Social Media Statistics 2020: Top Networks By the Numbers: https://dustinstout.com/social-media-statistics/
2. THE CONTENT MODERATION REPORT: Social platforms are facing a massive content crisis — here's why we think regulation is coming and what it will look like: https://www.businessinsider.com/content-moderation-report-2019-1
3. The Problem With AI-Powered Content Moderation Is Incentives Not Technology: https://www.forbes.com/sites/kalevleetaru/2019/03/19/the-problem-with-ai-powered-content-moderation-is-incentives-not-technology/#6caa805c55b7
4. Behind the Screens book: https://www.behindthescreen-book.com
5. The Trauma Floor - The Secret Lives of Facebook Moderators in America: https://www.theverge.com/2019/2/25/18229714/cognizant-facebook-content-moderator-interviews-trauma-working-conditions-arizona
6. Why a Top Content Moderation Company Quit the Business Instead of Fixing its Problems: https://www.theverge.com/interface/2019/11/1/20941952/cognizant-content-moderation-restructuring-facebook-twitter-google
Imagga Joins the Fight Against COVID-19—Develops Software for Early Diagnosis of Infectious Diseases
Kelvin is a platform for monitoring and early diagnosis of infectious diseases that have epidemic/pandemic character.
Our lives are a perpetual transfer of energy - our blood constantly bringing nutrition, protection, and heat to all our organs and body parts. In the intangible but ever-present world of mathematics every single thing in the universe can be described with a mathematical equation. With project Kelvin we unite experts in thermal cameras and optics, image recognition and bio-imaging, respiratory infections and diseases, Big Data and AI/ML analysis, mobile applications development, UI and UX on a mission to protect your and your family’s health by early diagnosis of highly-infectious diseases.
Kelvin works via directly attached thermal-imaging camera to a mobile device (iOS, Android) that feeds image data to a mobile application with an embedded image and medical analysis algorithms.
Our body typically exhibits certain normal temperature deviations in the different body zones. When there’s an inflammatory process, caused by a virus, COVID-19 including, the changes in the deviations can give early signals for a viral infection. The normal thermometers are not capable of detecting these subtle changes, but the thermal cameras are. The image recognition technology developed by Imagga’s team analyzes the personal thermal pictures and the periodic changes in it. Using a specialized algorithm to maximize the accurate assessment of the individual's current state and their respiratory system activity, Kelvin determines the likelihood of a viral infection.
Kelvin utilizes Imagga’s advanced AI technology to address the currently unfolding global crisis due to COVID-19. It can be used by individuals to efficiently monitor their health status (existence and development of infections) in the home environment. The platform can also be used by infected patients to control the inflammation progress, send/receive data to GPs and make timely decisions for hospitalization.
The desired outcomes include virus spread control, monitoring the progress of the disease - stages and complications, opportunity for daily check of the health status of non-hospitalized individuals.
The successful implementation of Kelvin will be of great help to the healthcare systems around the world in the current COVID-19 pandemic situation, allowing the proper prioritization of urgent measures to those who need it most and reducing the unneeded and risky exposure to medical institutions of those who do not.
Project Kelvin is in active development and we’ll provide updates on the progress we’re making.
Image Recognition Explained - Content Moderation
How Are Websites Affected by UK Government Internet Safety Regulations?
With the exponential rise of user-generated content shared online, the need for content screening moderation is increasing. And if until now it was up to the businesses to decide whether they screen content or not, soon they will be required to do so by official content moderation regulations.
The UK government recently announced that Ofcom, the country’s communications regulator, will be put in charge to oversee the internet for two specific areas covering illegal and harmful content. Ofcom will be watching that illegal content is immediately taken down with a particular focus on terrorism and child abuse images, but also prevent the posting of such content in the first place. The organization will also be empowered to fine platforms for user-generated content (UGC) such as social media for serving harmful content to its users.
The proposed legislation yet to be passed but it is clear that it will soon be a reality. With the UK leading the pack, other governments will follow and more companies globally will be affected.
There's a convenient and affordable solution. In this short video, our CEO Georgi Kadrev explains how your company can benefit from an AI-powered content moderation solution.
Any website which published UGC is affected by content moderation regulations
Virtually any website which operates with user-submitted content needs to screen it in order or reduce online harm. And there are many viable reasons for this with or without official legislation in place. The content referred to includes images, videos, and users' comments – thus affecting a large percentage of the online platforms and websites.
What are the options for content moderation?
Content moderation can be performed by humans, AI or both. Companies are still largely relying on human moderation, but this approach is expensive, difficult to scale and takes an emotional toll on the people performing the job. And while AI-powered content moderation addresses both the ethical and economic side of the problem, the algorithms are not yet sophisticated enough to fully take over. In most cases today, the best approach is using both human and AI content moderation – in the most practical and safe ways. Combining the power computer vision and human judgment holds huge potential for moderating a massive amount of violent, pornographic, exploitative and illegal content and protect the internet from online bullies and criminals.
Learn more about Imagga Content Moderation platform.
Image Recognition Explained – Image Tagging
This blog post series explains in simple words the technologies associated with image recognition.
Image tagging is possibly the most widely used technology, being related to the organization of visual content which every large visual database needs. It automatically assigns keywords and tags to images and videos and can be applied to millions of visuals, which alternatively would require days of tedious and repetitive manual work. For this to happen a computer algorithm is trained with a huge amount of visuals and it “learns” from them, starting to recognize the people, objects, places or other attributes it has been trained with.
In this short video, Imagga CEO, Georgi Kadrev talks about image tagging and its applications.
Image tagging is often times combined with a similar technology that puts the images into relevant categories, called image categorization. This solves a major pain for companies operating with massive image databases – it provides fast and consistent image keywording, which is critical to image searchability.
Using our own Image Recognition API we created a free plugin for Adobe Lightroom to make it accessible to photographers who need to have control over their photo collections. Wordroom automatically offers diverse keywords with high accuracy and users can add them to the image’s metadata with a single click. Learn more about how to use Wordroom here.
Industries that use image tagging extensively include stock photography and photo sharing, DAM, advertising, commerce and retail, travel and booking platforms, real estate and more. Picture tagging has application in virtually any platform or system that operates with a large image or video database and wants to have control over their visual content.
Coming up in the next episode is Content Moderation.
Image Recognition Explained - Part 1
Image recognition and the technologies behind it are quickly finding application in industries spanning from media, retail and telecoms to automotive, healthcare, security and surveillance. Still fairly new as a concept and turned into a hype word, image recognition often lies within the blind-spot of many businesses that can benefit significantly from putting it to use. Talking to prospects we find out that a huge part of the potential users of the technology don’t realize that they have a use case for the technology. And that it is affordable too.
In the Image Recognition Explained series we’ll cover the major technologies related to it and their applications to help business owners understand if they have a use case for image recognition or not.
What is image recognition?
Image recognition is the ability of computer software to recognize objects, places, people, faces, logos, and even emotions in digital images and videos. A computer algorithm is being trained with a large amount of visual data using machine learning and as a result the algorithm starts recognizing the type of visual content it has been trained with. Unlike the human brain, artificial intelligence sees the images as complex mathematical matrices, and can recognize people and objects within very high confidence levels.
Which are the typical applications of image recognition in different industries?
Automatic classification and categorization – technologies such as image tagging and categorization allow automation of processes that alternatively involve gigantic manual effort and are often times impossible to tackle. Automatic tagging and classification of huge visual databases are used for organization and discovery of media content by DAM systems, stock photography platforms, hotel and travel booking systems, and can be used by virtually any platform hosting visual content in the range of hundreds of thousands to billions of images.
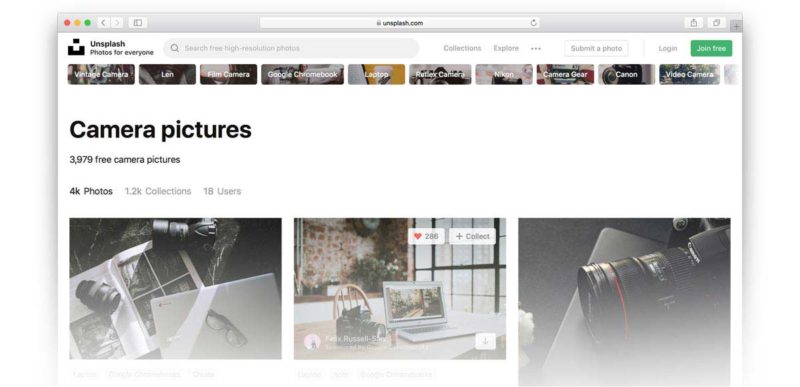
Read how Unsplash improved their image search and user experience.
Photo and video organization – technologies such as image tagging, facial recognition and Not Safe for Work (NSFW) models are used to enable image organization and discovery for Telecom clouds, Dropbox and Google Photos-like apps. Beside securely storing user media content, companies need to provide value by offering better search and discovery capabilities on a level equal or superior to the widely used iCloud Photos and Google Photos.
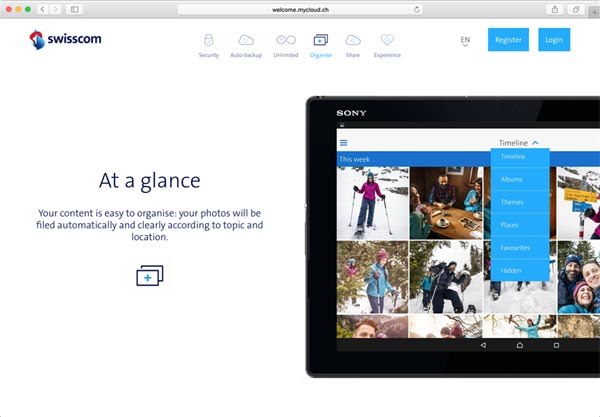
Read how Imagga Image Recognition technology helped Swisscom to provide better visual content organization for individuals and businesses.
Users profiling & insight – image recognition can be used for matching interests & behavior with visual content. Used by advertisement platforms and social media analytics systems image recognition technology can provide valuable insights on users’ preferences and sentiment for a product or brand based on analyzing the content of the pictures she shares online.
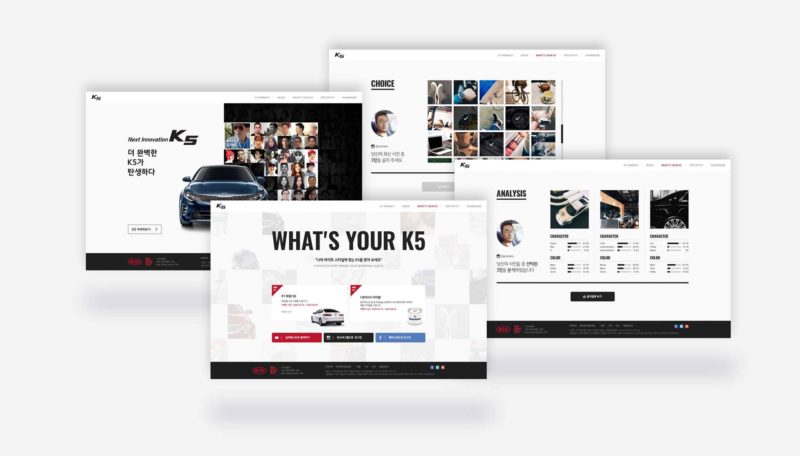
Easier product discovery – fashion retail, e-commerce and home goods leaders are building visual search within their websites and mobile apps to make product discovery easier, offer product recommendations and alternatives to products out of stock.
Facial detection and recognition – companies such as telecoms use face recognition technology allow their users to organize their personal photos by the people in the images. Typical applications of face recognition are in video surveillance and analysis and access control.
Visual and text content moderation – virtually any platform that operates with user generated content (UGC) needs to monitor it and remove illegal and abusive images, videos and text. Learn how Imagga Content Moderation solution can help you address the screening of massive content in order to avoid hurting vulnerable groups and legal issues.
What’s next?
Check out the video for Image Tagging
Check out the video for Content Moderation
Stay tuned for the technologies behind image recognition explained…
How Imagga Facial Recognition Works?
We’re excited to share that we’ve just added another powerful image recognition capability to our offering – Facial Recognition API for face detection and recognition of faces in still images and live stream videos.
Using the API, companies can implement face recognition functionality in their web, desktop, and mobile applications and systems.
How the facial recognition algorithm sees a face?
The face recognition algorithm “sees” a face as a complex numerical code containing hundreds of facial measurements. Examples of facial measurements include: the distance between the eyes, the width of the nose, length of the jawline, depth of the eye sockets, shape of your cheekbones, and more. These specific facial measurements are called nodal points, and each face has 500 such points. The unique combination of all these measurements creates an anonymous numerical code, which represents the face in the database.
How facial recognition works?
- Face detection
First, the face is extracted from the rest of the image using a technology called object localization. - Face analysis
Often the head is tilted, which can distort the data, so the technology locates the face attributes like eyes, nose and ears to correct the face rotation or tilt. Then it takes the facial measurements of the reference image and creates the unique numerical code we mentioned above. - Comparison
The last step is looking for the exact match or one with a high level of confidence in a pre-defined or custom, still or dynamic database.
Is face verification possible with Imagga Facial Recognition API?
Face verification compares a reference image of a face to another already present in the database and checks if there is a match. Our API now supports this functionality out-of-the-box and you can implement it directly in your application. Read how.
Facial recognition use cases
The facial recognition market is expected to grow at a fast pace due to increased adoption in both law enforcement and non-law enforcement sectors.
Source: Markets and Markets
Editorial Management Systems use facial recognition to identify and recognize celebrities, politicians, and other public figures — for efficient organization and search in large media archives.
It can be used for personal photo organizations by companies such as telecoms allowing their users to organize their personal photos by the people in the images.
In the video surveillance and analysis sector, facial recognition can be used for the analysis of video footage to identify key people and their attributes like age, gender and ethnicity.
Another typical application of facial recognition is access control. The technology can be used to ensure that only authorized individuals enter facilities like bank vaults, labs, and other sensitive locations, as well as for the time-tracking of employees.
Read more about the image recognition trends in 2020.
You have a case for image recognition? Let’s talk
ViewBug Uses Imagga AI Content Moderation to Filter Through Millions of Images
ViewBug is a web and mobile platform for visual creators with more than 2 million members and tens of thousands of image uploads every day. Like a Behance for the enthusiast photographer it gives photographers opportunities to be discovered and improve their skills offering a full suite of tools that help them learn, work better, and make more money.
As many user generated content platforms, ViewBug needs a safety shield against offensive content that could harm the brand’s reputation, disturb vulnerable groups and cause compliance and legal issues. Filtering out adult images, which are not allowed in their platform, posed a challenge for the ViewBug team given the large scale volume of visual content generated by their users every day. They needed an automated content moderation software that was reliable, precise, accessible and easy to implement.
After looking at a few solutions, they chose Imagga Not Safe for Work (NSFW) classifier - adult image content moderation categorizer trained on state of the art image recognition technology. It was integrated in the code, verifying every single image upload in real-time to make sure that it is not explicit content.
The NSFW classifier is part of Imagga AI-powered Content Moderation solution. It’s designed to help visual content platforms of any size keep their reputation and content safe in a cost-efficient and scalable way. It will offer automated detection of diverse and customizable categories of inappropriate content and can be deployed in the cloud and on-premise.
You have a similar need and you’re not sure what’s the best solution for you? Get in touch with us to discuss your case.
Image Recognition Trends in 2020
The image recognition market is estimated to reach USD 38.9 billion by 2021, compared to USD 15.9 billion in 2016 — more than 100% increase for the projected period, according to data by Markets and Markets research. Companies in e-commerce, automotive, content sharing, healthcare and security are rapidly implementing image recognition and driving the adoption of the technology. Other contributing factors are the sophistication of facial recognition, content moderation and other algorithms, the rise in popularity of media cloud services, the surge in mobile devices equipped with cameras and hardware technological advancements.
What is Image Recognition?
Image recognition refers to technologies that can detect and identify people, places, objects, logos, emotions and other variables in still images and videos. Image recognition is a subset of computer vision and is based on AI and machine learning.
What is image recognition used for?
One of the most widely used applications of image recognition is the automatic detection and tagging of people, objects and places in large visual databases. Other technologies with increasing popularity are facial recognition used in security, biometrics and personal photos platforms, and content moderation for filtering offensive and illegal visual content.
Which Image Recognition Technologies Will be on the Rise in 2020?
Content Moderation
Billions of users share content online and tons of it falls within a range from inappropriate to outright illegal. Platforms operating with user-generated content (UGC) face a significant challenge — how to efficiently and effectively monitor UGC and block inappropriate and offensive images, videos and text. Content moderation done poorly can disturb vulnerable groups, hurt the brands reputation and cause compliance and legal issues.
Who takes care of the “toxic digital garbage”?
Content moderation can be performed by humans, AI or both. More than 100,000 people worldwide monitor the most violent, pornographic, exploitative and illegal content to protect internet users from online bullies and criminals. The job is tough and can take its emotional and mental toll.
Cognizant, one of the top content moderation companies, just recently announced its decision to exit the business and laid off 6,000 people, performing content moderation for clients such as Facebook among others. A memo from the CEO of the company to its employees stated that while thousands of jobs will be eliminated, Cognizant will contribute to the development of machine-learning systems that can replace the human moderators job.
AI-powered or automated content moderation addresses both the ethical and economic side of the problem. The algorithms, processing the majority of the content and sending just a small fraction of it to human moderators can significantly mitigate the detrimental effect this job has on people. Furthermore it is vastly more productive, easier to scale and ultimately less expensive than human moderation.
Automated content moderation explained
Image Tagging
Image tagging is one of the earliest applications of image recognition in businesses, however its adoption will continue to grow as more companies embrace AI to offer better user experience or improve internal workflows.
What is image tagging?
Image tagging is the automatic assignment of relevant tags or keywords to vast collections of images and videos. In order for this to happen a deep learning model is trained to analyze the pixel content of visuals, to extract their features and detect objects of interest. It is a cost-effective and time-saving solution for companies operating with massive amounts of image content often coming from different sources.
This technology, often times combined with complementing capabilities such as image categorization solves a common pain of platforms operating with large image databases — inadequate image descriptions making the images hard to find, while searchability is critical for these platforms. Often times the images are uploaded by different users, vendors or employees which results in incoherent meta-data describing the images or even lack of any description. This is where image tagging comes in — it can tag coherently a multi million image database in a matter of hours.
Image tagging is widely used in stock photography and photo sharing. Read why Unsplash chose to incorporate Imagga image recognition API in their tagging system.
Advertisers, publishers and ad agencies also use the image tagging technology to get better at contextual advertising. SEEDPOST used Imagga Image Tagging and Color Extraction APIs to analyze image content form user’s social accounts and created, based on image understanding, 36 different lifestyles that match the highly customizable experience of KIA’s new K5 (Optima) model. Read the case study.
Visual Search
Visual search means searching for an image with a similar image. The technology allows users to search and find products similar to the ones they shot with their camera or downloaded from the web. In contrast, the search for images as we know it today relies on the accuracy of the images text description. Visual search delivers, where text search fails.
Apparel, home decor brands and retailers are among the early adopters of the technology, as it allows for easier product discovery and shortens the path to conversion.
Another benefit of the technology lies in the ability to provide product recommendations based on true similarity rather than other users’ preferences. Visual search can power similar products suggestions to offer a bigger choice or to provide alternatives to products out of stock.
Read more about how retailers use visual search for a competitive advantage
Facial Recognition
Facial recognition is widely used in government applications and law enforcement, and is gaining more popularity in commercial applications for use cases such as contactless payments, access control through facial biometrics and more. The technology is expected to witness the highest growth rate in the upcoming years due to increased adoption in both law enforcement and non-law enforcement sectors.
Image source: https://www.alliedmarketresearch.com/image-recognition-market
Facial recognition can be used as a key component in every photo organization application or service — allowing its users to search and filter photos by people in the shot.
Editorial Management Systems use facial recognition to identify and recognize celebrities, politicians, and other public figures — for efficient organization and search in large media archives.
In the security and law enforcement sectors, facial recognition is used for detection and prevention of crimes. An example of such application is an app that helps police officers identify individuals on the field giving them data about these individuals, so they know who they are dealing with from a safe distance.
Another typical application of facial recognition is access control. The technology can be used to ensure that only authorized individuals enter facilities like bank vaults, labs and other sensitive locations, as well as for time-tracking of employees.
Are you future proof?
If you are operating with large visual databases on the front-end and/or back-end of your business applications you most probably have a case for visual AI. The wide array of technologies commonly known as image recognition can help you achieve results which would be impossible without AI, or deliver a better user experience to your end users. Our 10+ years of expertise in delivering image recognition solutions to companies across diverse industries, large and small, has shown us that most cases require a custom approach and a combination of 2 or more technologies. We’ve helped companies coming to us with a vague definition of a need or a straightforward list of requirements.
You have a case for image recognition? Let’s talk!