Top 5 Adult Content Detectors You Need to Know About
Keeping online content safe isn’t just a priority - it’s a necessity. For businesses, AI-driven content moderation tools have become critical in protecting users and ensuring compliance with ever-evolving regulations. One of the most vital tasks in this space? Filtering adult content. Today’s AI-powered tools offer real-time protection, helping platforms stay safe and user-friendly.
From social media and e-commerce to educational websites, dating platforms and digital communities, adult content detection serves two primary goals: meeting legal obligations and safeguarding user trust. Whether it's about protecting young users or maintaining a brand’s image, the stakes are higher than ever. Below, we explore why adult content filtering matters, which businesses need it most, and five leading AI tools to help you stay compliant and safe.
Why Adult Content Detection Is Essential
As regulatory frameworks tighten - EU’s Digital Services Act, Section 230 of the Communications Decency Act in the U.S., and the Online Safety Bill in the UK - platforms are legally bound to protect their users. Beyond compliance, adult content detection is crucial for fostering a safe and welcoming online experience.
This process involves removing explicit user-generated content from platforms where it doesn’t belong. AI-driven algorithms excel here, scanning images, videos, and live streams in real-time to catch explicit material with impressive accuracy.
Businesses like social media platforms, e-commerce marketplaces, dating apps, and educational websites face unique challenges. For platforms catering to minors or educational spaces, the stakes are even higher. Balancing between user safety and freedom of expression is no easy task, but modern AI models are stepping up to solve it.
Who Needs Adult Content Detection?
Adult content detection isn’t just for social media giants. Any platform that allows user-generated content needs a strategy for keeping things clean. Here’s a quick look at who benefits most:
- Dating platforms - ensuring shared photos and messages remain appropriate
- E-commerce sites - keeping user-uploaded product photos and reviews family-friendly.
- Online communities - gaming forums, interest groups, and other communities with visual content sharing
- Educational platforms - protecting minors and maintaining professional environments
- Social media and messaging apps - where user-generated content flows constantly
Top 5 Tools for Adult Content Detection
1. Imagga Adult Content Detection
The Imagga Adult Content Detection model has been around for a while, but was previously known as NSFW detection. After significant improvements, it has now achieved a whooping 98% in recall for explicit content detection and 92.5% overall model accuracy. The new model has been trained on an expanded and diversified training dataset consisting of millions of images of different types of adult content.
The model is able to differentiate content in three categories with high precision: explicit, suggestive, and safe. It thus provides reliable detection for both suggestive visuals containing underwear or lingerie and for explicit nudes and sexual content. The adult content detection operates in real time, preventing inappropriate content from going live unnoticed. Imagga offers both a Cloud API and on-premise deployment.
With a stellar recall rate of 98% for explicit content and 92.5% accuracy overall, Imagga Adult Content Detection sets new standards in content moderation.
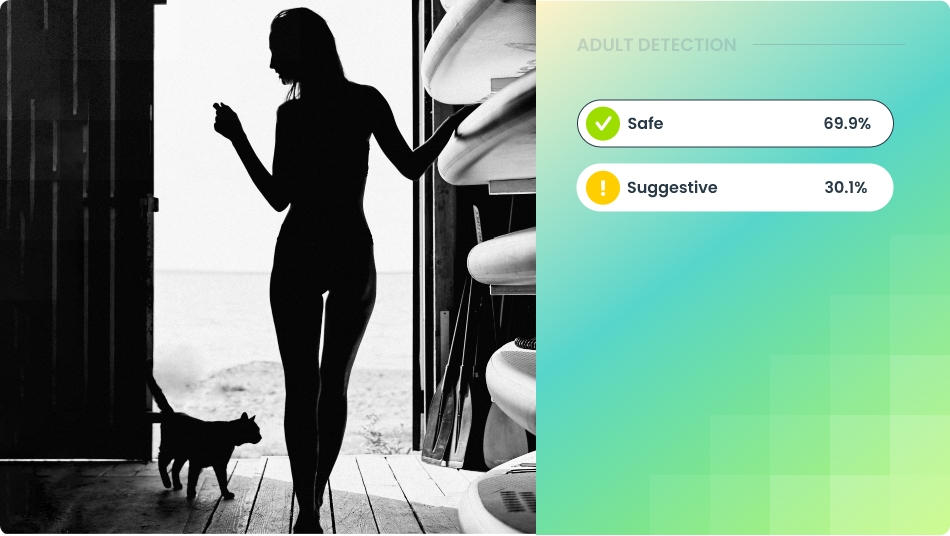
2. Google Cloud Vision AI
The Cloud Vision API was launched by Google in 2015 and is based on advanced computer vision models. At first, it was targeting mainly image analysis like object and optical character recognition. Today it also boasts a special tool for detecting explicit content called SafeSearch. It works with both local and remote image files.
The Cloud Vision API is powered by the wide resource network of Google, which fuels its accuracy in adult content detection too. SafeSearch detects inappropriate content in five categories — adult, spoof, medical, violence, and racy. The API is highly scalable and can process significant amounts of detection requests, which makes it attractive for platforms with large-scale user-generated content.
The Google Cloud Vision AI has a readily available API (REST and RPC) and can be easily integrated with other Google Cloud services.
3. Amazon Rekognition
Amazon Web Services (AWS) introduced Amazon Rekognition in 2016. It provides computer vision tools for the cloud, including content moderation. Amazon Rekognition can detect and remove inappropriate, unwanted, or offensive, which spans adult content.
Powered by a deep learning model, Amazon Recognition can identify unsafe or inappropriate content in both images and videos with high precision and speed. The identification can be based on general standards, or can be business-specific. In particular, businesses can use the Custom Moderation option to adapt the model to their particular needs. They can upload and annotate images to train a custom moderation adapter.
The enterprise-grade scalable model can be integrated hassle-free with a Cloud API, as well as with other AWS services.
4. Sightengine
Sightengine is a platform solely focused on content moderation and image analysis. It boasts 110 moderation classes for filtering out inappropriate or harmful content, including nudity. Sightengine works with images, videos, and text. It also has AI-image and deepfake detection tools, as well as a feature for validating profile photos.
Specifically for NSFW content, Sightengine has a Nudity Detection API that can classify content according to different levels of explicitness or suggestiveness. It can filter explicit and partial nudity based on an analysis of 30 different concepts, including pose, clothing, context, framing, and more. The filtering can be additionally improved by adding extra classes and contextual data. The model has eight pre-set severity levels, so that businesses can set the right limits on explicit content for their purposes.
Sightengine is easy to integrate through its API and is fully privacy compliant, since there are no human moderators involved and images are not shared with any third parties.
5. Clarifai
Clarifai offers a full-stack AI lifecycle platform, and one of its solutions is the content moderation platform. It employs deep learning AI models to provide moderation and filtering of images, videos, text, and audio. Among its features is the NSFW filtering, which can identify whether a visual contains NSFW or not.
When it comes to adult content detection, Clarifai can classify content in three categories: either safe, suggestive, or explicit. It uses pre-trained models built with popular taxonomies like GARM. Clarifai allows for creating custom-trained models that are particularly tailored for the needs of a specific brand. Clarifai is easily deployable and scalable.
Stay Compliant and Protect your Users
Adult content detection has become the norm for digital platforms in different business fields, helping companies stay compliant with legal regulations regarding explicit content, as well as protect users from being exposed to unwanted and inappropriate content.
At Imagga, we build state-of-the-art technology in all of our image recognition tools - and this is the case with our Adult Content Detection Model too. If you want to learn how it can help your business, get in touch or check out how to get started with the model in no time.
Automated Content Moderation | What Is It? Benefits, tools and more
Automated content moderation plays a key role in keeping digital platforms safe and compliant as user-generated content grows rapidly. From social media posts to video-sharing platforms, content moderation help detect harmful content quickly and consistently, handling far more material than human moderators alone could manage. The benefits are clear - faster moderation, cost savings, and real-time filtering - but challenges remain. Automated systems can struggle with context, show bias, and raise privacy concerns, making accuracy and fairness ongoing issues.
As platforms work to protect users while respecting free speech, automated moderation continues to evolve. This blog will break down how these systems work, their strengths and weaknesses, key trends shaping the future, and the ethical considerations needed to create safer, fairer online spaces.
Automated content moderation uses AI-powered tools and algorithms to review, filter, and manage user-generated content across digital platforms. It automatically assesses text, images, videos, livestreams, and audio for compliance with predefined standards. The goal is to prevent the spread of harmful content, misinformation, copyright violations, or inappropriate material. This process helps maintain a safe, respectful, and legally compliant online environment by scaling content oversight far beyond human capacity, often combining machine learning models with customizable rule sets for accuracy and efficiency.
Benefits of Automated Content Moderation
The advantages of using automated moderation are numerous. The new technology has practically revolutionized how platforms based on user-generated content are handling these processes.
Scalability
Automated content moderation can process vast amounts of user-generated content in real-time, making it ideal for platforms with high traffic and frequent uploads. Unlike human moderation, which is limited by time and workforce capacity, automated content moderation can handle millions of visuals, posts, comments, and media files simultaneously, ensuring faster content review. This level of scalability is essential for global platforms seeking to maintain quality standards across diverse audiences.
Cost-Effectiveness
By reducing the need for large teams of manual moderators, automated content moderation significantly lowers operational costs for digital platforms. AI-driven tools can manage most routine content review tasks, allowing human moderators to focus on complex or ambiguous cases. This balance minimizes expenses while maintaining content quality and compliance, especially for platforms dealing with massive amounts of user-generated content daily.
Protection of Human Moderators
Automated content moderation can shield human moderators from exposure to harmful and disturbing content by filtering out explicit material before human review is required. This helps protect moderators' mental health by reducing their direct exposure to traumatic material. It also allows human reviewers to focus on nuanced decisions rather than routine filtering tasks.
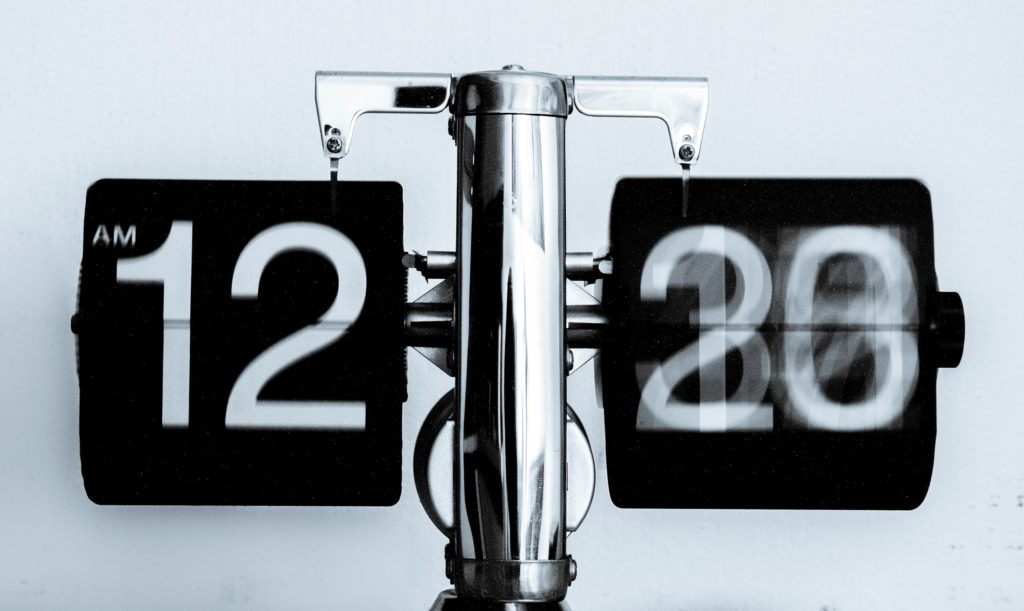
Real-Time Moderation
Automated systems can instantly detect and act on harmful content as it is uploaded, preventing it from reaching audiences in the first place. This real-time intervention is especially critical in fast-paced environments like live streaming or comment sections, where harmful content can spread rapidly if not addressed immediately.
24/7 Operation
Automated moderation tools work around the clock without breaks, ensuring continuous content monitoring across all time zones. This persistent oversight is crucial for global platforms where content activity never stops, offering a consistent safeguard against harmful material at all times.
Customizability
AI-powered moderation systems can be tailored to match the unique content guidelines, brand values, and legal requirements of each platform. Custom rule sets allow for a more precise application of standards, making it possible to address platform-specific needs, cultural sensitivities, and industry regulations with greater accuracy.
Legal Compliance
Automated moderation assists platforms in meeting legal obligations by enforcing content policies in line with regional laws and industry standards. By proactively identifying and filtering out illegal content, such as hate speech, harassment, or copyrighted material, these tools help reduce liability and ensure regulatory compliance across multiple jurisdictions. Check out this comprehensive guide to content moderation regulations.
Limitations of Automated Content Moderation
While automated content moderation offers significant benefits, it also comes with several limitations that can impact its effectiveness and fairness. One of the primary challenges is contextual misunderstanding. AI systems often struggle to interpret the nuances of language, humor, sarcasm, and cultural differences, which can lead to the misclassification of harmless content as harmful or vice versa. For example, a sarcastic comment criticizing hate speech could be mistakenly flagged as offensive, while subtle forms of harassment might go undetected due to a lack of contextual awareness.
Bias and fairness are also critical concerns. Automated moderation systems are trained on datasets that may reflect existing societal biases, leading to unfair treatment of certain groups or perspectives. This can result in the disproportionate removal of content from marginalized communities or the failure to flag harmful material affecting those groups. Ensuring fairness requires ongoing adjustments and diverse, representative training data, which can be complex and resource-intensive to maintain.
Over-blocking and under-blocking are common issues as well. Automated systems may excessively filter benign content like artistic expressions or educational discussions while failing to detect evolving harmful content designed to bypass filters. Dependence on predefined rules and data further limits adaptability, as AI models can struggle to keep up with shifting cultural norms, slang, and emerging threats without frequent updates.
While these challenges can limit the effectiveness of automated moderation, many can be mitigated with expert training and advanced AI models designed for greater nuance. For example, Image Adult Content Detection demonstrates how specialized training can significantly improve moderation accuracy and fairness, enhancing its ability to provide nuanced content classification.
How Does Automated Content Moderation Work?
Automated content moderation can be used in different ways, depending on the needs of your platform:
- Pre-moderation: algorithms screen all content before it goes live
- Post-moderation: content is screened shortly after it’s gone live; this is the most popular method
- Reactive moderation: users report posts for inappropriateness after they have been published
Whichever method you choose, your first step will be to set your moderation policy. You’ll need to define the rules and types of content that have to be removed, depending on the overall strategy of your platform. Thresholds also have to be set, so that the moderation tool has clear demarcation when content violates your standards.
In the most common case of post-moderation, all user-generated content is processed by the moderation platform. On the basis of the set rules and thresholds, clearly inappropriate content is immediately removed. Due to the automation, this can happen quite soon after it’s been published. Some items are considered trickier by the algorithm, and they are fed for human review. Content moderators access the questionable items through the moderation interface. Then they make the final decision to keep or remove the content.
Whenever content is forwarded for manual moderation, the training data from the human moderators’ actions feeds back into the automated moderation platform. In this way, the AI learns from the subtleties in human decisions to remove or keep certain content. With time, the new learnings enrich the algorithms and make the automatic process more and more accurate.
What Type of Content Can You Moderate Automatically?
You can use automated content moderation with all types of content — visual, textual, and even with moving images.
1. Visuals
With the help of computer vision, automated platforms can identify inappropriate content in images through object detection mechanisms. They use algorithms to recognize unwanted elements and their position for an understanding of the whole scene. Offensive text can also be spotted, even if it is contained in an image.
The types of inappropriate visuals you can catch with fully automated content moderation include:
- Nudity and pornography
- Self-harm and gore
- Alcohol, drugs, and forbidden substances
- Weapons and torture instruments
- Verbal abuse, harsh language, and racism
- Obscene gestures
- Graffiti and demolished sights
- Physical abuse and slavery
- Mass fights
- Propaganda and terrorism
- Infamous or vulgar symbols
- Infamous landmarks
- Infamous people
- Horror and monstrous images
- Culturally-defined inappropriateness
2. Text
Natural language processing (NLP) algorithms can recognize the main meaning of a text and its emotional charge. Automated moderation can identify the tone of the text and then categorize it thanks to sentiment analysis. It can also search for certain keywords within textual content. Additionally, built-in knowledge databases can be used to predict the compliance of texts with moderation policies.
Algorithms can screen for:
- Bullying and harassment
- Hate Speech
- Trolling
- Copyrighted text
- Spam and scam
- Fraudulent text
- Pornographic text
3. Video
AI moderation for videos involves frame-by-frame analysis to detect explicit scenes, violence, or sensitive content. It can also involve audio transcription to screen spoken language within videos for harmful speech or misinformation.
4. Audio
Content moderation tools can process audio files and transcripts to detect offensive language, hate speech, and violations of content guidelines. Speech recognition technology allows for the automatic flagging of harmful content in podcasts, voice messages, and livestream audio.
Emerging Trends in Automated Content Moderation
As digital platforms evolve, so do the technologies driving automated content moderation. Recent advancements are making AI tools more sophisticated, accurate, and adaptable to the dynamic nature of online content. Key emerging trends include:
Multi-Modal AI
Multi-modal AI combines the analysis of multiple content types, such as text, images, audio, and video, within a single moderation system. By examining content holistically, these models can better understand context and reduce false positives or missed violations. For example, a video can be analyzed not just for visuals but also for spoken language and captions simultaneously, providing a more comprehensive moderation process.
Real-Time Moderation
With the rise of livestreaming and interactive content, real-time moderation has become essential. AI systems are increasingly capable of scanning content instantly, flagging or removing harmful material before it reaches audiences. This trend is particularly impactful in social media, gaming, and e-commerce platforms where content can go viral within minutes.
User-Customizable Filters
Customization is becoming a focal point, allowing platforms and even end users to set their own content preferences. User-customizable filters enable tailored moderation, where individuals or brands can adjust sensitivity levels for different types of content, ensuring a balance between content freedom and community safety. This personalization makes moderation more adaptable across various platforms and audience types.
Sentiment Analysis
Beyond detecting harmful content, sentiment analysis tools are being integrated into moderation systems to better understand the emotional tone behind posts and comments. By assessing whether content is aggressive, sarcastic, or supportive, these tools help platforms moderate in a more context-aware manner. Sentiment analysis can also aid in preventing online harassment and fostering healthier digital interactions.
These trends are shaping a future where automated content moderation is not only more effective but also more adaptable to the diverse needs of digital platforms and their audiences.
Automated Content Moderation Solutions
Imagga’s content moderation platform provides you with all the tools you need to automate your moderation process. It’s a powerful and easy-to-use solution that you can integrate in your operations — and prepare your platform for scaling.
Imagga’s AI-powered pre-trained systems analyze all content on the basis of the moderation guidelines that you have set. Our API learns on the go too, so it improves with every project it processes.
In the Admin Dashboard, you can create different categories of inappropriate content to look for and define the parameters for each. You can set priority levels for projects, as well as thresholds for flagging and forwarding content for human moderation. You can also control data retention length.
The Moderation Interface is crafted to make your human moderators’ job easier. They get automatically prescreened content that they can review faster and with reduced risks because the most horrible content has already been removed. Moderators can use handy hotkeys and organize their work effectively in the interface.
With Imagga’s content moderation platform, you can effectively ensure the protection of your users, your brand reputation, and your human moderators. You can use our tools in the cloud or on premise — and you can easily plug them in your current processes, whether you have an in-house or an outsourced moderation team.
Automated Content Moderation Case Studies
1. Live Streaming Use Case
- Simultaneous moderation of live video streams is needed
- Can’t be done via manual moderation due to privacy concerns
- Automated moderation guarantees the privacy
- Done in short interval of time, and if a problematic stream is detected, escalated to the website admins to follow nsfw policies - sending warning or/and terminating the stream, etc
2. Dating website Use Case
- similar to above but images uploaded for profile, videos and live stream chat if supported
- Different levels of moderation regarding country of operation and type of dating website.
- Automated CM removes the privacy concerns as it might be very sensitive when it comes to dating websites
Read how Imagga Adult Content Detection helped a leading dating platform transform their content moderation.
3. Travel web sites Use Case
- Moderation of both images and texts - travel sights are better thanks to the reviews visitors leave - moderation of the text and images/videos is needed
- Automated CM makes possible real time publishing of reviews when they pass the auto CM filter
Ethical Considerations in Automated Content Moderation
As automated content moderation becomes more widespread, ensuring its ethical implementation is critical to maintaining fairness, accuracy, and respect for user rights. Several key considerations must be addressed:
Safety and Free Speech Balance
Striking the right balance between user safety and freedom of expression is a core challenge in content moderation. While automated systems can effectively filter harmful content, they may also suppress legitimate discussions on sensitive topics. Ethical moderation requires safeguards to avoid unnecessary censorship while still protecting users from harm.
Bias Reduction
Automated moderation systems can unintentionally reflect biases present in their training data, leading to unfair treatment of certain groups or viewpoints. This can result in the disproportionate removal of content from marginalized communities or the failure to identify subtle forms of harm. Ethical content moderation involves diverse, representative datasets and continuous auditing to minimize bias.
Cultural Sensitivity
Content that is offensive in one cultural context may be acceptable in another, making it challenging for AI systems to apply uniform standards globally. Ethical moderation practices must consider cultural differences and avoid enforcing a one-size-fits-all approach. This may include localized content guidelines and region-specific moderation settings.
Transparency
Users deserve to understand how and why their content is being moderated. Ethical content moderation requires clear communication of policies, the use of AI tools, and decision-making processes. Providing users with detailed explanations and appeals processes can help build trust and accountability in automated systems.
Privacy Compliance
Automated moderation often involves scanning user-generated content, raising privacy concerns. Ethical implementation requires strict adherence to data protection laws like GDPR and CCPA, ensuring that user data is handled securely and only to the extent necessary for moderation purposes.
Frequently Asked Questions
Automated content moderation is highly effective for clear-cut violations like explicit imagery, hate speech, and spam. Advanced AI models can detect harmful content with minimal human intervention but may struggle with nuanced cases such as sarcasm or culturally sensitive material. Accuracy improves with expert training, diverse datasets, and human oversight, helping reduce both harmful content exposure and unnecessary censorship.
Platforms balance automated moderation and user privacy by limiting data collection to what’s necessary for content analysis while ensuring compliance with privacy laws like GDPR and CCPA. Techniques such as data anonymization and encryption help protect personal information during moderation processes. Clear policies on data use, along with transparency about how content is reviewed, further safeguard user privacy while maintaining platform safety.
Automated moderation systems handle live content by using AI tools capable of real-time analysis, scanning visuals, audio, and text as content is broadcasted. These tools can instantly flag or block harmful material, such as offensive language, explicit imagery, or harassment, to prevent policy violations before reaching the audience. However, live moderation often requires a combination of automated filters and human oversight to address complex cases and reduce false positives.
Automated moderation systems often face challenges such as contextual misunderstanding, where sarcasm, satire, or cultural nuances can lead to incorrect content flagging. Bias can also arise from imbalanced training data, causing unfair treatment of certain groups. Additionally, over-blocking and under-blocking may occur, where harmless content is removed or harmful material goes undetected. Keeping up with evolving language and trends can also be difficult, requiring regular updates to maintain accuracy and fairness.
What Is Content Moderation? | Types of Content Moderation, Tools, and more
The volume of content generated online every second is staggering. Platforms built around user-generated content face constant challenges in managing inappropriate or illegal text, images, videos, and live streams.
Content moderation remains the most effective way to safeguard users, protect brand integrity, and ensure compliance with both national and international regulations.
Keep reading to discover what content moderation is and how to leverage it effectively for your business.
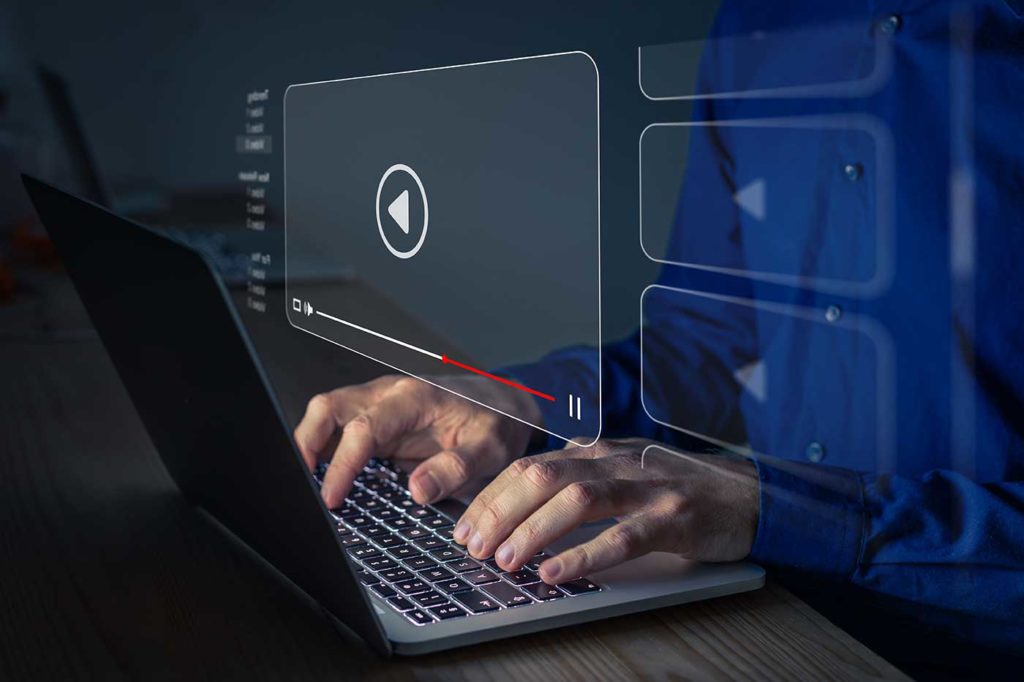
What Is Content Moderation?
Content moderation is the process of reviewing and managing user-generated content to ensure it aligns with a platform's guidelines and community standards. It involves screening posts, comments, images, videos, and other submissions for violations such as violence, explicit content, hate speech, extremism, harassment, and copyright infringement. Content that fails to meet these standards can be flagged, restricted, or removed.
The primary purpose of content moderation is to create a safer, more respectful online environment while protecting a platform’s reputation and supporting its Trust and Safety initiatives. It plays a critical role in social media, dating apps, e-commerce sites, online gaming platforms, marketplaces, forums, and media outlets. Beyond public platforms, it’s also essential for corporate compliance, ensuring internal communications and public-facing content remain professional and within legal boundaries.
Why Is Content Moderation Important?
Because of the sheer amount of content that’s being created every second, platforms based on user-generated content are struggling to stay on top of inappropriate and offensive text, images, and videos.
Content moderation is the only way to keep your brand’s website in line with your standards — and to protect your clients and your reputation. With its help, you can ensure your platform serves the purpose that you’ve designed it for, rather than giving space for spam, violence and explicit content.
Types of Content Moderation
Many factors come into play when deciding what’s the best way to handle content moderation for your platform — such as your business focus, the types of user-generated content, and the specificities of your user base.
Here are the main types of content moderation processes that you can choose from for your brand.
1. Automated Moderation
Moderation today relies heavily on technology to make the process quicker, easier and safer. These advancements have been made possible by the evolution in image recognition accuracy and AI as a whole.
AI-powered content moderation allows for effective analysis of text, visuals and audio in a fraction of the time that people need to do that, and most of all — they don’t suffer psychological traumas from processing inappropriate content. Among its business benefits are also ensuring legal compliance, supporting multilingual moderation, and overall flexibility and adaptability of the process.
When it comes to text, automated moderation screens for keywords that are deemed as problematic. More advanced systems can spot conversational patterns and relationship analysis too.
As for visuals, image recognition powered by AI tools like Imagga offer a highly viable option for monitoring images, videos and live streams. Such solutions identify inappropriate imagery and have various options for controlling threshold levels and types of sensitive visuals.
Human review may still be necessary in more complex and nuanced situations, so automated moderation usually implies a mixture between technology and human moderation.
2. Pre-Moderation
This is the most elaborate way to approach content moderation. It entails that every piece of content is reviewed before it gets published on your platform. When a user posts some text or a visual, the item is sent to the review queue. It goes live only after a content moderator has explicitly approved it.
While this is the safest way to block harmful content, this process is rather slow and not applicable for the fast-paced online world. However, platforms that require a high level of security still employ this moderation method. A common example are platforms for children where security of the users comes first.
3. Post-Moderation
Post-moderation is the most typical way to go about content screening. Users are allowed to post their content whenever they wish to, but all items are queued for moderation. If an item is flagged, it gets removed to protect the rest of the users.
Platforms strive to shorten review times, so that inappropriate content doesn’t stay online for too long. While post-moderation is not as secure as pre-moderation, it is still the preferred method for many digital businesses today.
4. Reactive Moderation
Reactive moderation entails relying on users to mark content that they find inappropriate or that goes against your platform’s rules. It can be an effective solution in some cases.
Reactive moderation can be used as a standalone method, or combined with post-moderation for optimal results. In the latter case, users can flag content even after it has passed your moderation processes, so you get a double safety net.
If you opt to use reactive moderation only, there are some risks you’d want to consider. A self-regulating platform sounds great, but it may lead to inappropriate content remaining online for far too long. This may cause long-term reputational damage to your brand.
5. Distributed Moderation
This type of moderation relies fully on the online community to review content and remove it as necessary. Users employ a rating system to mark whether a piece of content matches the platform’s guidelines.
This method is seldom used because it poses significant challenges for brands in terms of reputation and legal compliance.
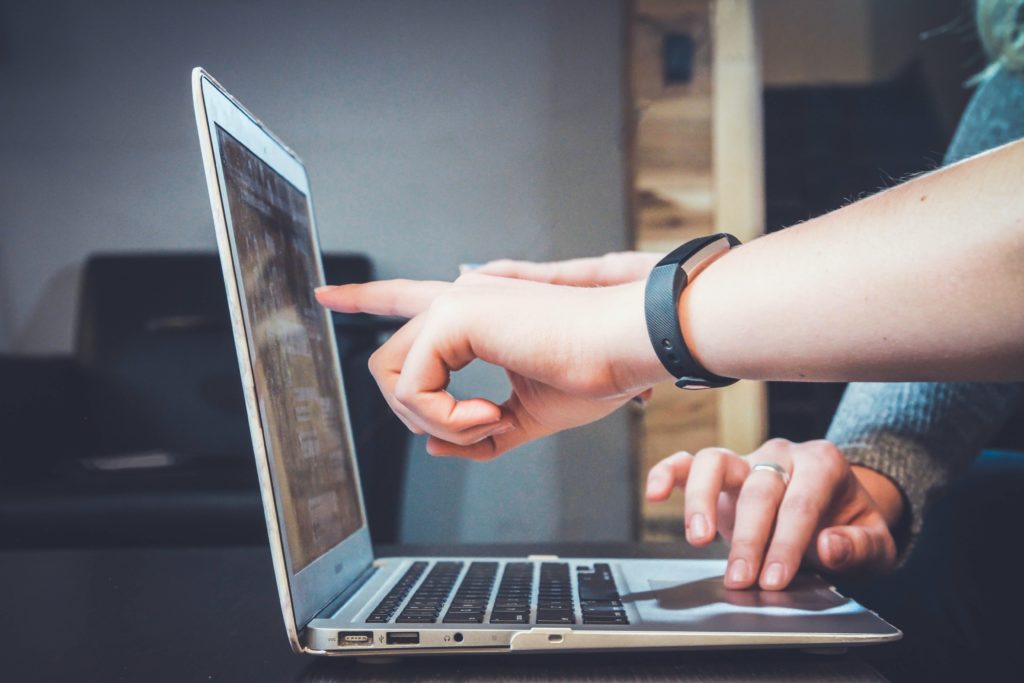
Content Moderation Process
The first step for an effective moderation process, including automated content moderation, is setting clear guidelines about what constitutes inappropriate content. This is how the content moderation system and the people who will be doing the job — content moderators — will know what to flag and remove. Typically, creating the guidelines is tightly connected with setting your overall Trust & Safety program.
Besides types of content that have to be reviewed, flagged and removed, you have to define the thresholds for moderation. This refers to the sensitivity level when reviewing content. Thresholds are usually set in consideration of users’ expectations and their demographics, as well as the type of business. They would be different for a social media platform that operates across countries and for an ecommerce website that serves only a national market.
What Types of Content Can You Moderate?
Moderation can be applied to all kinds of content, depending on your platform’s focus - text, images, video, and even live streaming.
1. Text
Text posts are everywhere — and can accompany all types of visual content too. That’s why moderating text is one of the prerogatives for all types of platforms with user-generated content.
Just think of the variety of texts that are published all time, such as:
- Articles
- Social media discussions
- Comments
- Job board postings
- Forum posts
In fact, moderating text can be quite a feat. Catching offensive keywords is often not enough because inappropriate text can be made up of a sequence of perfectly appropriate words. There are nuances and cultural specificities to take into account as well.
2. Images
Moderating visual content is considered a bit more straightforward, yet having clear guidelines and thresholds is essential. Cultural sensitivities and differences may come into play as well, so it’s important to know in-depth the specificities of your user bases in different geographical locations.
Reviewing large amounts of images can be quite a challenge, which is a hot topic for visual-based platforms like Pinterest, Instagram, and the like. Content moderators can get exposed to deeply disturbing visuals, which is a huge risk of the job.
3. Video
Video has become one of the most ubiquitous types of content these days. Moderating it, however, is not an easy job. The whole video file has to be screened because it may contain only a single disturbing scene, but that would still be enough to remove the whole of it.
Another major challenge in moderating video content is that it often contains different types of text too, such as subtitles and titles. They also have to be reviewed before the video is approved.
4. Live Streaming
Last but not least, there’s live streaming too, which is a whole different beast. Not only that it means moderating video and text, but it has to occur simultaneously with the actual streaming of the content.
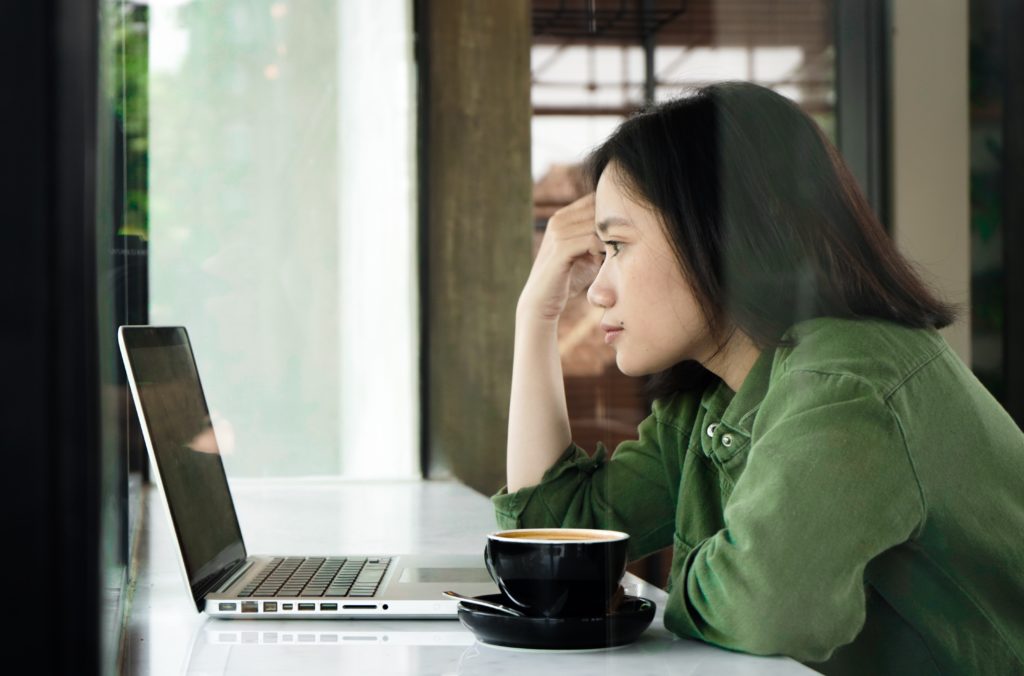
The Job of the Content Moderator
In essence, the content moderator is in charge of reviewing batches of content — whether textual or visual — and marking items that don’t meet a platform’s pre-set guidelines. This means that a person has to manually go through each item, assessing its appropriateness while reviewing it fully. This is often rather slow — and dangerous — if the moderator is not assisted by an automatic pre-screening.
It’s no secret today that manual content moderation takes its toll on people. It holds numerous risks for moderators’ psychological state and well-being. They may get exposed to the most disturbing, violent, explicit and downright horrible content out there.
That’s why various content moderation solutions have been created in recent years — that take over the most difficult part of the job.
Content Moderation Use Cases
Content moderation has use cases and applications in many different types of digital businesses and platforms. Besides social media where it’s being used massively, it’s also crucial for protecting users of online forums, online gaming and virtual worlds, and education and training platforms. In all these cases, moderation provides an effective method to keep platforms safe and relevant for the users.
In particular, content moderation is essential for protecting dating platform users. It helps remove inappropriate content, prevent scam and fraud, and safeguard minors. Moderation for dating platforms is also central to ensuring a safe and comfortable environment where users can make real connections.
E-commerce businesses and classified ad platforms are also applying content moderation to keep their digital space safe from scam and fraud, as well as to ensure the most up-to-date product listings.
Content moderation has its application in news and media outlets too. It helps filter out fake news, doctored content, misinformation, hate speech and spam in user-generated content, as well as in original pieces.
Content Moderation Solutions
Technology offers effective and safe ways to speed up content moderation and to make it safer for moderators. Hybrid models offer unseen scalability and efficiency for the moderation process.
Tools powered by Artificial Intelligence, such as Imagga Adult Content Detection hold immense potential for businesses that rely on large volumes of user generated content. Our platform offers automatic filtering of unsafe content — whether it’s in images, videos, or live streaming.
You can easily integrate Imagga in your workflow, empowering your human moderation team with the feature-packed automatic solution that improves their work. The AI-powered algorithms learn on the go, so the more you use the platform, the better it will get at spotting the most common types of problematic content you’re struggling with.
Frequently Asked Questions
Here are the hottest topics you’ll want to ask about content moderation.
Content moderation is the process of screening for inappropriate content that users post on a platform. The meaning of content moderation is to safeguard the users from any content that might be unsafe or illegal and might ruin the reputation of the platform it has been published on.
Content moderation can be done manually by human moderators that have been instructed what content must be discarded as unsuitable, or automatically using AI platforms for precise content moderation. Today, a combination of automated content moderation and human review for specific cases is used for faster and better results.
While AI tools can filter vast amounts of content quickly, human moderators remain essential for context-driven decisions. Sarcasm, cultural nuances, and evolving slang often require human judgment to avoid mistakes. The most effective systems combine AI efficiency with human empathy and critical thinking.
Platforms operating globally face the challenge of aligning with diverse legal frameworks and cultural expectations. In such cases, content moderation often adapts with region-specific guidelines, balancing global standards with local laws and values—though this can create tensions when rules seem inconsistent.
Absolutely. A gaming platform might prioritize moderation for harassment and cheating, while a dating app focuses more on preventing fake profiles and explicit content. Each platform requires a tailored moderation approach based on its unique risks and user interactions.
Content moderation isn't about silencing voices - it's about creating a space where conversations can happen without harm. While platforms encourage diverse opinions, they draw the line at content that incites harm, spreads misinformation, or violates established guidelines. The goal is to promote respectful discourse, not censorship.
Image Recognition Trends for 2025 and Beyond
What lies ahead in 2025 for image recognition and its wide application in content moderation?
In this blog post, we delve into the most prominent trends that will shape the development of AI-driven image recognition and its use in content moderation in the coming years, both in terms of technological advancements and responding to perceived challenges.
Image recognition is at the heart of effective content moderation, and is also the basis of key other technological applications such as self-driving vehicles and facial recognition for social media and for security and safety. It’s no wonder that the global image recognition market is growing exponentially. In 2024 it was valued at $46.7 billion and is projected to grow to $98.6 billion by 2029.
As a myriad of practical uses of AI across the board explode, including AI video generation, deepfake, and synthetic data model training, content moderation will become more challenging — and will have to rely on the evolution of image recognition algorithms. At the same time, the plethora of issues around AI ethics and biases, paired with the more stringent content moderation regulations on national and international level, will drive a significant growth in image recognition’s power and scope.
Without further ado, here are the nine trends we believe will shape the path of image recognition and content moderation in 2025.
AI Video Generation Goes Wild
AI-powered video generation started going public in 2022, with a bunch of AI video generators now available to end users. Platforms like Runway, Synthesia, Pika Labs, Descript and more are already being used by millions of people to create generative videos, turn scripts into videos, and edit and polish video material. It’s safe to say AI video generation is becoming mainstream.
In 2025, we can expect this trend to set off massive changes in how videos are being created, consumed, and moderated. As generative AI tools make giant leaps, AI video generators will become even more abundant and more precise in creating synthetic videos that mimic life. Their use will permeate all digital channels that we frequent and will become more visible in commercials and art forms like films.
At the same time, the implications of these changes for the film industry and for visual and cinema artists will continue to raise questions about ownership and copyright infringements, as well as about the value of human creativity vs. AI generation.
Real-Time Moderation for Videos and Live Streaming
AI-powered image recognition is already at the heart of real-time moderation of complex content like videos and especially live streaming. It allows platforms to ensure a safe environment for their users, whether it’s social media, dating apps, or e-commerce websites.
As we move forward into 2025, we can expect real-time moderation to become ever more sophisticated to match the growing complexity and diversity of live video streaming. AI moderation systems will grow their capabilities in terms of contextual analysis needed to grasp nuances and tone, as well as in terms of multimodal moderation of video, audio and text within livestreams. They will also get better at predicting problematic content in streams.
Moderation is also likely to follow the pattern of personalization that’s abundant in social media and digital communications. Platforms may start allowing users to choose their own moderation settings, such as levels for profanity and the like.
Multi-Modal Foundational AI Models
Multi-modality is becoming a necessity in understanding different data types at the same time, including visual and textual. They combine visual, audio, text and sensor data to grasp the full spectrum and context of content. Multi-modal foundational AI models are advanced systems that are able to put together different data inputs into one in order to bring out deeper levels of meaning and help decision-making processes in various settings.
In 2025, multi-modal AI models will grow, integrating many different types of data for all-round understanding. Cross-modality understanding will thus gradually become the norm for AI interactions that will grow to resemble human ones.
Multi-modal AI models will bring about new applications in robotics, Virtual Reality and Augmented Reality, accessibility tools, search engines, and even virtual assistants. They will enable more comprehensive interactions with AI that are closer to human experience.
Deepfake and AI-generated Content Detection
The spread of deepfake and AI-generated content is no longer a prediction for the future. Deepfakes are all around us and while they might be entertaining to watch when it comes to celebrities and favorite films, their misuse in representing politicians, for example, is especially threatening. Discerning between authentic and AI-generated content is becoming more and more complex, since AI becomes better at it.
As people find it harder to tell the truth from fiction, detection of AI-generated content and deepfakes will evolve to meet the demand — the AI vs. AI battle. AI-powered detection and verification tools will become more sophisticated and will provide real-time identification, helping people differentiate content on the web in order to prevent fraud, scams, and misuse.
We can expect to see the development of universal standards for detection and marking of AI-generated content and media, as well as databases with deepfakes that will help identification of fraudulent content. As the issue circulates in the public's attention, people will also get better at identifying fake materials — and will have the right tools to do so.
AI-Driven Contextual Understanding
Comprehensive understanding of content, including context, tone, and intent, is still challenging for AI, but that is bound to change. We expect AI to get even better at discerning the purpose and tone of content — grasping nuances, humor, and satire, as well as harmful intentions.
Contextual understanding will evolve to improve the accuracy of AI systems, as well as our trust in them. We’ll see more and more multi-modal context integration that enables all-round understanding, including intent and nuances detection. Cultural specificities will also come into play, as AI models are already embedding cultural contexts and differences.
In 2025, we can also expect AI to develop further in spotting emotions and impact in content, getting closer to our human perception of data. Developments in contextual understanding will be driven by AI learning on-the-go from our changing trends in language and social norms.
Use of Generative AI and Synthetic Data for Models’ Training
AI models’ training relies on large amounts of data — which are often scarce or difficult to obtain for a variety of reasons, including copyright and ethical concerns.
Generative AI and synthetic data are already fueling models’ training, and we can expect to see more of that in the coming years. For example, synthetic data for content moderation is already in use, bringing about benefits like augmentation and de-biasing of datasets. However, it still requires in-depth supervision and input from real data.
Synthetic data will advance, creating accurate and hyper-realistic datasets for training. They will continue to help reduce bias in real-world datasets, plus will offer a way to scale and develop models that are working in more specific contexts.
AI-Human Hybrid Content Moderation
Content moderation is the norm in various digital settings, from social media and dating apps to e-commerce and gaming. Its development has been fueled by the application of AI-driven image recognition across the board — and this trend will continue, combining multi-modality and context understanding advancements.
At the same time, the AI-human hybrid content moderation is likely to stay around, especially when it comes to moderation of sensitive and culturally complex content. The speed and scale that AI offers in content moderation, particularly in live streaming and video, will continue to be paired with human review for accuracy and nuance detection.
Betting on the hybrid approach will make content moderation achieve even greater efficiency and balancing, accounting for ethical and moral considerations as they appear. As AI trains on human decisions, its ability to discern complex dilemmas will also evolve.
More Stringent Content Moderation Regulations
Legal requirements for content moderation are tightening, making digital platforms more accountable for the content they circulate and allow users to publish. As technology evolves, various threats evolve too — and national and international regulatory bodies are trying to catch up by adopting new legislation and enforcement methods.
In particular, the most important recent content moderation regulations bringing about higher transparency and accountability requirements include the EU’s Digital Services Act (DSA), fully enforced in February 2024, and the General Data Protection Regulation (GDPR) which is in action from 2018. In the USA, this is Section 230 of the Communications Decency Act, while in the UK — Online Safety Bill that was enforced at the end of 2023. The EU’s AI Act will come into effect in 2025, and in full force — in 2026.
We can expect the stricter legal requirements trend to continue in the coming years, as countries struggle to create an intentional framework for handling harmful content. AI accountability, fairness, transparency, and moral and ethical oversight will be certainly on the list of legislators’ demands.
Ethics and Bias Mitigation
As stricter regulations come into force in 2025, like the EU’s AI Act, people will demand more comprehensive ethical and bias mitigation. This is becoming especially important in the context of security surveillance based on facial recognition, as well as in biases in AI generative models.
Along with legal requirements, we’ll see new ethical frameworks for AI and image recognition in particular being developed and applied, which might even evolve into ethical certifications. Besides fair use, inclusivity and diversity will also become focal points that will be included in ethical guidelines. All of this will require the efforts of experts from the AI, law, and ethics fields.
Bias mitigation will rely on diversifying datasets for model training, be it real-life or synthetic. The goal will be to decrease biases based on gender, race, or cultural differences, which can also be helped by automated bias auditing systems and by real-time bias detection.
Discover the Power of Image Recognition for Your Business
Imagga has been a trailblazer in the image recognition field for more than a decade. We have become the preferred tech partner of companies across industries, empowering businesses to make the most of cutting-edge AI technology in image recognition.
Get in touch to find out how our image recognition tools — including image tagging, facial recognition, visual search, and adult content detection — can help your business evolve, stay on top of image recognition trends, and meet industry standards.
A Comprehensive Guide to Content Moderation Regulations
The digital world is expanding and transforming at an unprecedented pace. Social media platforms now serve billions, and new channels for user-generated content emerge constantly, from live-streaming services to collaborative workspaces and niche forums. This rapid growth has also made it easier for harmful, illegal, or misleading content to spread widely and quickly, with real-world consequences for individuals, businesses, and society at large.
In response, governments and regulatory bodies worldwide are stepping up their scrutiny and tightening requirements around content moderation. New laws and stricter enforcement aim to address issues like hate speech, misinformation, online harassment, and child protection. The goal is to create safer digital spaces, but the impact on platform operators is profound - those who fail to implement effective moderation strategies risk heavy fines and serious reputational damage.
If your business hosts any form of user-generated content – whether posts, images, videos, or posts – you can’t afford to ignore these developments. This article will guide you through the major content moderation regulations shaping today’s digital environment. Knowing these regulations is essential to protect both your platform and its users in a world where the expectations and penalties for content moderation have never been higher.
Content Moderation and The Need for Regulations
In essence, content moderation entails the monitoring of user-generated content, so that hate speech, misinformation, harmful and explicit content can be effectively tackled before reaching users. Today the process is typically executed as a mix between automated content moderation powered by AI and input from human moderators on sensitive and contentious issues.
While effective content moderation depends on tech companies, different regulatory bodies across the world have taken on to create legal frameworks. They set the standards and guide the moderation measures that social media and digital platforms have to apply. Major cases of harmful content mishandling have led to these regulatory actions by national and multinational authorities.
However, getting the rules right is not an easy feat. Regulations have to balance between protecting society from dangerous and illegal content while preserving the basic right of free expression and allowing online communities to thrive.
The Major Content Moderation Regulations Around the World
It’s challenging to impose content moderation rules worldwide, so a number of national and international bodies have created regulations that apply to their territories.
European Union: DSA and GDPR
The European Union has introduced two main digital regulation acts: the Digital Services Act (DSA) that came in full force in February 2024 and the General Data Protection Regulation (GDPR) applied since 2018.
The purpose of the DSA is to provide a comprehensive framework for compliance that digital platforms have to adhere to. It requires online platforms and businesses to provide a sufficient level of transparency and accountability to their users and to legislators, as well as to protect users from harmful content specifically.
Some specific examples include requirements for algorithm transparency, content reporting options for users, statements of reasons for content removal, options to challenge content moderation decisions, and more. In case of non-compliance with the DSA, companies can face penalties of up to 6% of their global annual turnover.
The GDPR, on the other hand, targets data protection and privacy. Its goal is to regulate the way companies deal with user data in general, and in particular during the process of content moderation.
USA: Section 230 of the Communications Decency Act
The USA has its own piece of legislation that addresses the challenges in content moderation. This is Section 230 of the Communications Decency Act. It makes it possible for online platforms to moderate content, as well as taking away from them the liability for user-generated content.
At the same time, Section 230 is currently under fire for not being able to address the recent developments in the digital world, and in particular generative AI. There are worries that it would be used by companies developing generative AI to escape legal responsibilities for potential harmful effects of their products.
UK: Online Safety Bill
At the end of 2023, the Online Safety Bill came into force in the United Kingdom after years in the making. Its main goal is to protect minors from harmful and explicit content and to make technological companies take charge of content on their platforms.
In particular, the Bill requires social media and online platforms to be responsible for removing content containing child abuse, sexual violence, self-harm, illegal drugs, weapons, cyber-flashing, and more. They should also require age verification where applicable.
A number of other countries have enforced national rules for content moderation that apply to online businesses, including European countries like Germany but also others like Australia, Canada, and India.
Challenges of Content Moderation Regulations
The difficulties of creating and enforcing content moderation regulations are manyfold.
The major challenge is the intricate balancing act between protecting people from harmful content and at the same time, upholding freedom of speech and expression and people’s privacy. When rules become too strict, they threaten to suffocate public and private communication online — and to overly monitor and regulate it. On the other hand, without clear content moderation regulations, it’s up to companies and their internal policies to provide a sufficient level of protection for their users.
This makes the process of drafting content moderation regulations complex and time consuming. Regulatory bodies have to pass the legal texts through numerous stages of consultations with different actors, including consumer representative groups, digital businesses, and various other stakeholders. Finding a solution that is fair to parties with divergent interests is challenging, and more often than not, lawmakers end up in situations of compliance rejection and lawsuits.
A clear example of this conundrum is the recently enacted Online Safety Bill in the UK. It aims to make tech companies more responsible for the content that gets published on their digital channels and platforms. Even though its purpose is clear and commendable — to protect children online — the bill is still contentious.
One of the Online Safety Bill requirements is especially controversial, as it requires messaging platforms to screen user communication for child abuse material. WhatsApp, Signal, and iMessage have declared that they cannot comply with that rule without hurting the end-to-end encryption of user communication, which is meant to protect privacy. The privacy-focused email platform Proton has expressed a strong opinion against this rule, too, since it allows government interference in and screening of private communication.
The UK’s Online Safety Bill is just one example of many content moderation regulations that have come under fire from different interest groups. In general, laws in this area are complex to draft and enforce — especially given the constantly evolving technology and the respective concerns that come up.
The Responsibility of Tech Companies
Technology businesses have a lot on their plate in relation to content moderation. There are both legal and ethical considerations, having in mind that a number of online platforms cater mostly to minors and young people, who are the most vulnerable.
The main compliance requirements for digital companies vary across different territories, which makes it difficult for international businesses to navigate them. In the general case, they include child protection, harmful and illegal content removal, and disinformation and fraud protection, but every country or territory can and does enforce additional requirements. For example, the EU also includes content moderation transparency, content removal reports, and moderation decisions.
Major social media platforms have had difficult moments in handling content moderation in recent years. One example is the reaction to the COVID-19 misinformation during the pandemic. YouTube enforced a strict strategy for removing videos but was accused of censorship. In other cases, like the Rohingya genocide in Myanmar, Facebook was accused of not taking enough steps to moderate content in conflict zones, thus contributing to violence.
There have been other long-standing disagreements about social media moderation, and nudity is a particularly difficult topic. Instagram and Facebook have been attacked for censorship of artistic female nudity and of breastfeeding. At the same time, Snapchat has been accused of its AI bot, which is producing adult-containing text in its communication with minors. It’s more than obvious that striking the right balance is tough for tech companies, as it is for regulators.
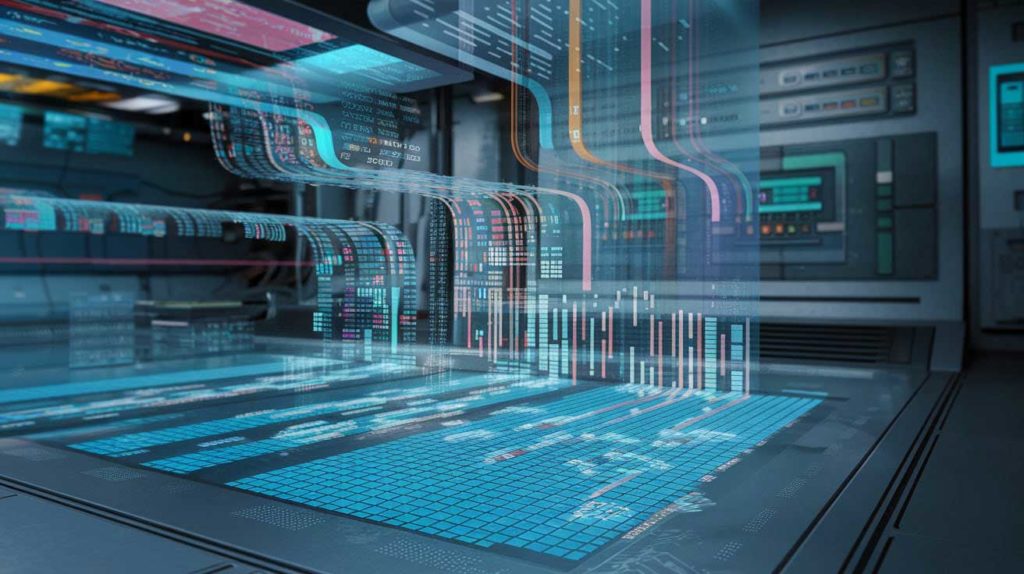
Emerging Trends in Content Moderation Regulations
Content regulation laws and policies need to evolve constantly to address the changing technological landscape. A clear illustration of this is the rise of generative AI and the lack of a legal framework to handle it. While the EU’s Digital Services Act came into force recently, it was drafted before generative AI exploded — so it doesn’t particularly address its new challenges. The new AI Act will partially come into force in 2025 and fully in 2026, but the tech advancements by then might already make it insufficient.
The clear trend in content moderation laws is towards increased monitoring and accountability of technological companies with the goal of user protection, especially of vulnerable groups like children and minorities. New regulations focus on the accountability and transparency of digital platforms to their users and to the regulating bodies. This is attuned to societal expectations for national and international regulators to step into the game — and exercise control over tech companies. At the same time, court rulings and precedents will also shape the regulatory landscape, as business and consumer groups challenge in court certain aspects of the new rules.
In the race to catch up with new technology and its implications, global synchronisation of content moderation standards will likely be a solution that regulators will seek. It would require international cooperation and cross-border enforcement. This may be good news for digital companies because they would have to deal with unified rules, rather than having to adhere to different regulations in different territories.
It is likely that these trends will continue while seeking a balance with censorship and privacy concerns. For digital companies, the regulatory shifts will certainly have an impact on operations. While it’s difficult to know what potential laws and policies might be, the direction towards user protection is clear. The wisest course of action is thus to focus on creating safe and fair digital spaces while keeping up-to-date with developments in the regulatory framework locally and internationally.
Best Practices for Regulatory Compliance
The complex reality of content moderation and its regulations today is that it requires digital companies to pay special attention and dedicate resources.
In particular, it’s a good idea for businesses to:
- Use the most advanced content moderation tools to ensure user protection
- Create and enforce community guidelines, as well as Trust and Safety protocols
- Stay informed about developments in the content moderation regulations landscape
- Be transparent and clear with users regarding your content moderation policies
- Have special training and information sessions for their teams on the regulatory framework and its application in internal rules
- Collaborate with other companies, regulators, and interest groups in the devising of new regulations
- Have legal expertise internally or as an external service
All in all, putting user safety and privacy first has become a priority for digital businesses in their efforts to meet ethical and legal requirements worldwide.
Image Recognition in Waste Management: How AI Can Make Our World Cleaner
The practical applications of Artificial Intelligence in our world today are ubiquitous, and image recognition in waste management is one of the curious cases in which new technology can bring immense positive changes.
Image recognition powered by AI is, in fact, already being explored as a tool for effective waste management. Many prototypes have been developed around the world, and some are gradually being deployed in practice. Waste detection and separation with the help of image recognition is still an activity driven by scientific researchers and remains yet to become a massive practice.
Let’s review how image recognition can assist in revolutionizing waste detection and sorting to achieve Zero Waste — helping humanity deal with the negative impacts of trash by turning it into usable resources that drive Industry 4.0.
The Global Issues in Waste Management Today
Exponentially increasing amounts of trash, pollution, risk of diseases, and economic losses — the aftereffects of improper waste management are numerous.
Despite technological advancements across the board, handling waste is one of the major issues in our modern world. According to the UNEP Global Waste Management Outlook 2024 report, municipal solid waste is bound to grow from 2.3 billion tonnes in 2023 to 3.8 billion tonnes by 2050. The global waste management cost in 2020 was $252 billion, but when taking into account the costs of pollution, poor health, and climate change, it’s estimated at $361 billion. The projected costs for 2050 are $640.3 billion unless waste management is rapidly revolutionized. All of this affects people on the whole planet, and as usual, its adverse effects are bigger in poorer areas.
Finding effective waste management solutions is important on a number of levels. Naturally, the issue of protecting people’s health is primary, as well as nature preservation — especially given the major problem with plastic waste that is cluttering our planet’s oceans. Beyond handling pollution and health issues, proper waste handling can also lead to adequate recycling and reusing of resources, as well as bring direct economic benefit to our societies. The UNEP Global Waste Management Outlook 2024 report points out that applying a circular economy model containing waste avoidance and sustainable business practices, as well as a complete waste management approach, can lead to a financial gain of USD 108.5 billion per year.
While there are advanced waste management systems around the world, a practical and affordable solution that can be applied in different locations across the globe has not yet been discovered. This is especially relevant for the major task of trash separation of biological, plastic, glass, metal, paper, and other materials for recycling, as well as for dangerous waste detection that safeguards people’s health. This is where image recognition powered by Artificial Intelligence can step in.
Image Recognition for Waste Detection and Sorting
The applications of Artificial Intelligence in waste management are growing, and the main approach that fuels this growth is the use of image recognition based on machine learning algorithms. With the help of convolutional neural networks (CNNs), waste management tools can find patterns in the analyzed visual data of trash, propelling the development of intelligent waste identification and recycling (IWIR) systems.
Use of Image Recognition in Waste Management
The main tasks of image recognition in waste management are identification of recyclable materials, waste classification, detection of toxic waste, and trash pollution detection. In addition, image recognition solutions for waste management have to offer a high degree of customization and flexibility to tailor to the difference between regions. For example, fine-tuning for different areas with specific trash images is essential. Training the algorithms with waste items particular to the specific demographic region is crucial for effective classification.
The goal of waste management systems powered by image recognition is to be able to identify and classify different types of trash. They are being trained with large image datasets, so that they can afterwards predict and spot hazardous trash and differentiate between different types of recyclable materials. In some cases, prototypes are made with robotic platforms that use sensors and microcontroller boards for accurate detection and processing, while in others, mobile phone terminals are being used.
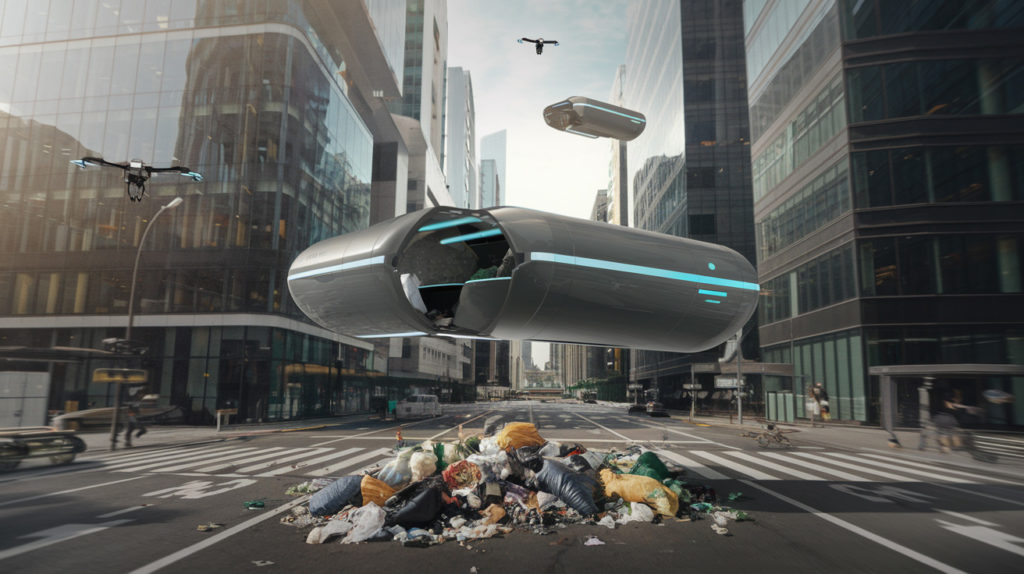
The Future Possibilities and Benefits
The use of image recognition in waste management, while developing rapidly, is yet to become widespread, but the possibilities and benefits it offers are highly promising.
The automatization of recyclable material sorting can have an immense impact on environmental sustainability, helping resource conservation and reduction of greenhouse gas emissions due to new production. It can increase the identification and use of valuable materials in trash, as well as reduce overall waste.
The shift in waste management with image recognition can lead to meaningful changes in waste management jobs. Image classification technology would save people from doing dangerous and dirty jobs, while ast the same time creating new opportunities in technology and data analysis.
Awareness about recycling and greater access to recycling services can grow as a result of image recognition-powered waste management systems. If recycling is more efficient and widespread, even communities with limited resources would get access to recycling options, as well as information about the benefits of recycling practices in daily life.
Use Case: Image Recognition in Waste Management
At Imagga, we do our best to push the boundaries of what’s possible with image recognition — and waste management is an area that we’ve already been exploring.
Already in 2016, we had a pilot trial with the Seoul National University, the most prestigious South Korean research university, on a project for waste management with image recognition capabilities. A team from a couple of departments at the University, led by Prof. Seong-Woo Kim and Dr. Daeun Kim, started the pilot project Fun with Trash to create a system that makes trash recycling easy and effective.
The prototype built by the team relied on a smart sensor system to identify the different types of recyclable trash that are being deposited in the different recycle bins (plastic, paper, glass, and metal). Once a person is about to throw a piece of trash, a camera sensor captures an image, and then the system recognizes the type of trash and compares it to a database of images. Then, the right bin is opened to deposit the trash.
Imagga jumped on board to help the SNU team with the image recognition technology to power up the recycling machine prototype. Our team trained the custom classifier for waste categorization and helped with improving its accuracy, reaching precision rates of 95%. Our experts also advised on the sensor positioning and illumination of the hardware setup.
Learn How Image Recognition Can Power Up Your Waste Management System
Imagga’s robust image recognition platform holds a huge potential for waste management systems due to our custom model training. In the context of waste management, custom model training is essential to provide the necessary flexibility to work in different demographic areas.
Our customizable tool allows you to define the categories in which you want to classify visuals so that the classification system is tailored to your particular needs. First, specific datasets are selected, and then we build a deep learning classification model trained with the particular categories and data. Afterward, our image categorization API does the rest of the job by fully automating the image categorization process.
Want to learn more about how image recognition can fuel the efficiency of waste management systems? Get in touch with us.
A Detailed Guide on Content Moderation for Trust & Safety
Ensuring a safe digital environment has become a top priority for forward-looking companies in the dynamically changing online landscape.
Trust and Safety (T&S) programs are the essential building blocks of these efforts — both in order to deliver the necessary protection for users and to comply with local and global safety rules and regulations.
Content moderation is one of the main and most powerful methods within Trust and Safety company policies. It ensures that all user-generated content published and distributed on a digital platform or app has passed a check for its appropriateness and safety. Moderation has become an indispensable tool for businesses of all venues — from social media and gaming to dating and media.
But content moderation doesn’t come without its challenges, including large volumes of content to be reviewed, balancing between moderation and free expression, and misuse of AI technologies, among others.
Different moderation methods offer different advantages and disadvantages, and below we take a look at how the various approaches can be used and combined — to achieve a company’s Trust & Safety objectives in the most effective way.
The Content Moderation Challenges that Trust and Safety Teams Face
Trust and Safety teams, whether in-house or external, are entrusted with a challenging task. They have to make the digital channels and platforms of a business safe and trustworthy for its customers by establishing and running highly effective T&S processes — while at the same time delivering on ROI expectations.
No pressure at all!
T&S teams have to shape and run a T&S program that identifies and manages risks that can negatively impact users and their experience with a brand. The programs have to be quite comprehensive so that they can ensure a safe and comfortable environment where customers can achieve their goals and feel at ease. This is how people’s trust in the brand can be enhanced — setting the ground for long-lasting relationships with customers.
Most importantly, T&S policies have to protect users from any kind of abuse while also adhering to safety and privacy rules applicable at local and international levels. And content moderation is the key to achieving both.
All of this sounds straightforward, but it is certainly not an easy feat. The challenges of getting content moderation right are numerous — and have different contexts and specifics.
Volume
First, there’s the volume. The amount of user-generated content that has to be sifted through is enormous — and it’s not only text and static images, but also includes more and more videos and live streams.
Striking a balance between moderation and censorship
Then there’s the delicate balance between removing harmful content, protecting free speech and expression, and avoiding bias while ensuring a great user experience. This complex balancing act involves both ethical and practical considerations that account for legal requirements, cultural specificities, and company goals — all at the same time.
Regulations
Naturally, legal compliance is a challenge on its own. Safety rules and regulations keep evolving along with new technology, and the EU’s Digital Services Act (DSA), the UK Online Safety Act, and Australia’s Online Safety Act are some of the prominent examples in this respect. Content moderation efforts have to be fully in tune with the latest regulatory activity — to ensure full protection for users and no liability for companies.
Generative AI content
Last but not least, there’s generative AI. While AI is powering content moderation, on the other side are deepfake, misinformation and fraud. Voice cloning and deepfake videos are a major threat to a safe online environment, and they create a pervasive sense that nothing can be trusted anymore. As it becomes more and more difficult to spot what’s genuine and what’s fabricated content, content moderation efforts have to keep up.
The Pros and Cons of the Different Content Moderation Approaches
While the present and future of content moderation are tightly linked to technology and automation, there are different approaches — and each of them has its benefits.
Currently, the most employed approach is hybrid, as it combines the best of manual human moderation and full automation. But let’s go briefly through each of the approaches.
Manual Moderation
In the first days of content moderation, it was all up to human moderators to clean up harmful and illegal content. This seems like madness from today’s point of view because people who did the job were exposed to the most horrific content. The growing amounts of user-generated content were unmanageable. The process was harmful, slow, and ineffective.
Luckily, these days are gone — but human input remains important for the nuanced and balanced content moderation of many online platforms.
Automated Moderation
The development of AI created the possibility of automating content moderation, and this has certainly proved to be a big breakthrough in the field. Automation allows for the processing of huge amounts of text and visual data, as well as the real-time moderation of complex content like live streams. Automated moderation is very good at identifying and removing content that is clearly illegal, explicit, or spam.
Naturally, automation has its downfalls. While precision has dramatically improved since the early days of AI content moderation, social and cultural nuances and contexts can still be challenging.
Hybrid Moderation
The hybrid approach puts together the best of both worlds — reaping the power of AI automation that provides scale and efficiency, while adding the precision and subtlety that human moderation allows for.
The combination provides for a constant balance between the productivity of technology and the social veracity and accuracy that only people can provide. The moderation tools mark content that is not straightforwardly inacceptable — and then it undergoes human review.
With continuous use, machine learning algorithms get better and better. The input from human moderators helps the AI platform develop a better understanding of more delicate elements in content, as well as their cultural meanings. The amount of content that gets processed also helps the platform learn and improve.
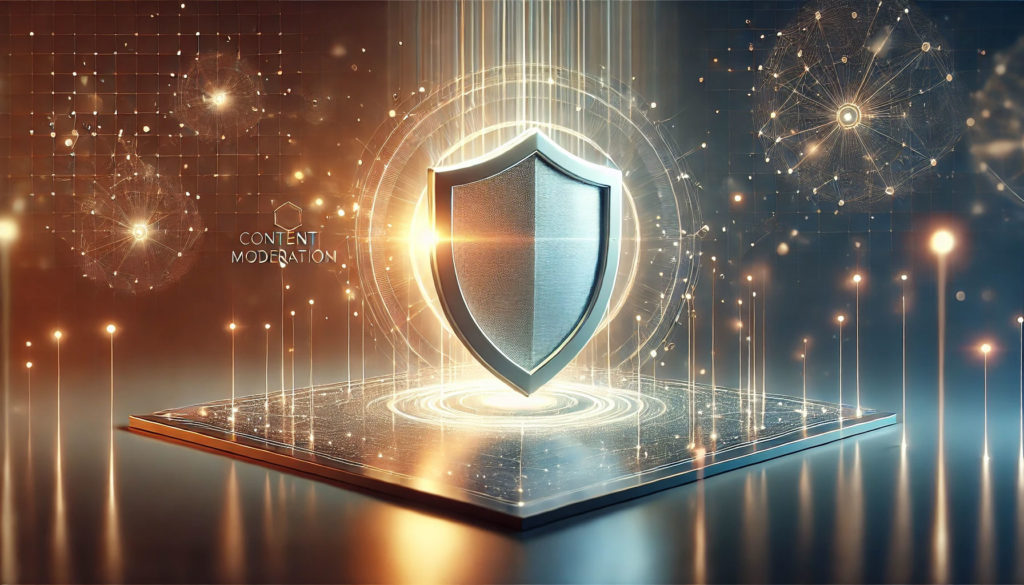
Buy vs. Build а Content Moderation Solution
Besides the different content moderation approaches, Trust & Safety teams have two main options for AI content moderation that they can choose from. They may decide to develop in-house content moderation tools or to use third-party vendors — also known as the build-or-buy dilemma.
Each option has its benefits and challenges — and the choice should be tailored to the particular needs of the company and its Trust & Safety team.
In-House
The path of creating in-house content moderation solutions is seen as giving the highest level of ownership over the tool and ability to craft it according to the specific business needs. However, it is certainly the most labor-intensive one and requires significant internal expertise in the field.
More specifically, companies have to add to their teams experts in advanced machine learning and AI, AI model training and optimization, and image and video processing. They also have to ensure the necessary infrastructure and resources, which entails computational power and data management. Last but not least, a major factor are the high development costs involved in creating an in-house moderation platform, as well as the lengthy time-to-market of the solution.
While building an in-house content moderation system might seem like the only way to maintain control and customization within the company, this path poses substantial challenges, especially for companies lacking expertise in image recognition and AI model training.
The in-house option usually makes the most sense for companies that are involved in digital security, Trust and Safety, and similar fields.
Third-Party Providers
With the growth and development of content moderation platforms, the option to use third-party vendors has become popular for many companies of all sizes.
Content moderation platform providers are top specialists in the field, employing the most cutting-edge AI content moderation tools. Since their focus is on building the best possible moderation platforms, they have the know-how and bandwidth to keep up with technological advancements, legal requirements, and usability expectations.
Using third-party content moderation providers ensures a high level of expertise and efficiency in the moderation process, as well as a guarantee for staying on top of digital and legal threats, but ownership of the moderation tool is not with the business. However, vendors provide solid options for data protection and privacy, as well as a high level of flexibility in terms of customization and features.
Introducing Imagga’s Robust Content Moderation Solution
Imagga has been developing AI-powered content moderation tools for more than a decade — and the results are impressive.
Our state-of-the-art platform identifies and automatically removes illegal and harmful content in images, videos, or live streams — including adult content, violence, drugs, hate, and weapons, among others. It boasts eight classification and detection models that target different types of unwanted content. The tool is also equipped to detect AI-generated visuals so that users can be warned about fabricated or fake content and protected from fraud and hate speech.
Packed with all these capabilities, Imagga’s content moderation platform provides a robust tool for Trust and Safety teams to get their job done in an easier and faster way.
Rolling out Imagga in your systems is a straightforward process. You can easily deploy the content moderation API and start using it in no time.
In case you want to make a hybrid mix between automatic AI content moderation and human input for subtleties, you can use our AI Mode hybrid visual content moderation platform. It allows for the seamless coordination and connection between automation that allows large-scale processing and human moderation for precision and nuances.
Get Started with AI Content Moderation Today
Ready to explore how AI content moderation can boost your Trust and Safety program? Get in touch today to learn how you can seamlessly integrate Imagga’s content moderation solution in your workflow.
The Power of Content Moderation in Protecting Dating Platform Users
Online dating platforms have to be a safe harbor for people looking for romance and honest human connections — or else they cannot serve their purpose. But the dangers posed by various malicious actors like scammers and catfishers are numerous.
Protection has thus become a high priority for dating sites and apps in their efforts to ensure a safe environment for their users, to shield their brand reputation, and to increase the satisfaction with their products.
Content moderation based on AI is a powerful ally of dating platforms in their battle with harmful content, scams, and fraud. Besides user privacy and safety, content moderation is the key to scalable and immediate filtering, enforcing age restrictions, and protecting human moderators from exposure to harmful content.
In the visual age of our digital world today, especially important is the robust AI-based visual content moderation. It is the only way to perform powerful and accurate real-time monitoring of images, videos and live streams.
Below you can find the seven top ways in which automated AI content moderation helps dating platforms protect their users and create thriving and safe online communities.
1. Dealing with Inappropriate Content
Harmful, illegal, and offensive content is ubiquitous online — and dating platforms are a primary target for malicious actors that want to distribute such content. It is often included in user profiles, and of course, in private messaging between users.
Having to constantly deal with irrelevant, abusive or explicit content is not optimal for people who turn to a platform to seek companionship and romance. In fact, it is a primary factor in dropping out of an online community — which is exactly the opposite of what dating platforms aim for. Inclusivity and safety are thus of primary concern.
Content moderation solutions based on AI tackle the big issue of inappropriate and harmful content with growing efficiency, accuracy and speed. Powered by constantly evolving machine learning algorithms, these solutions automatically filter out the content that is categorically not fit for the platform. They also flag content that is dubious — which then gets reviewed by human moderators to balance out protection and freedom of expression.
2. Scam and Fraud Prevention
Looking for love — and getting scammed instead. This is a classic scenario that many people either experience or are afraid of, and for a good reason. Dating platform users need, more than ever, reassurance that they are communicating online with real people with honest intentions.
With the help of AI content moderation, platforms are now able to provide this reassurance and protection. Checking the authenticity of user profiles can be automated with scanning of profile photos and analysis of profile information and patterns of behavior that point to scam and fraud.
Content moderation also provides effective monitoring for catfishing and bot activities. It allows platforms to minimize unwanted promotions, spam messaging, ads, money requests, and harmful links sharing, among other inappropriate content.
The AI-powered verification of profile authenticity has become an essential way to provide a safe and trustworthy environment where people can relax and focus on meaningful exchanges with potential soulmates.
3. User Privacy and Safety First
Ensuring a protected online environment is, clearly, of paramount importance for dating platforms — and user privacy and safety are central. In their private communications, people may be tempted to or lured into sharing sensitive private data, such as addresses, phone numbers, bank details, and more.
In such cases, dating platforms can use content moderation to identify and block the sharing of private data in order to shield users from harm and make sure their privacy and personal information is protected.
Other ways to ensure user safety is monitoring for stalking and abusive activities by other users. People can report such behavior, but with content moderation, dating platforms can also be a step ahead and have an overview on user activity that might be harmful for the rest of the online community. In addition, content moderation can provide personalized options, so users can set stricter filters for protection if they want to.
4. Safeguarding Minors
Enforcing age restrictions is a must for dating platforms, since they are targeted at individuals that are not minors. But checking the age of each and every user — especially when minors may try to conceal their age — can be a daunting task.
Content moderation comes in handy in this situation as well. Visual moderation helps analyze the images shared by users to assess their actual age and crosscheck it with the information they are sharing. In case the visual materials show a minor, the platform will be able to apply the age restrictions effectively.
The protection of minors is also active in in-app communication, where content moderation provides monitoring for harmful content sharing, abuse, and other potential dangers for younger users who have illegally gained access to the platform.
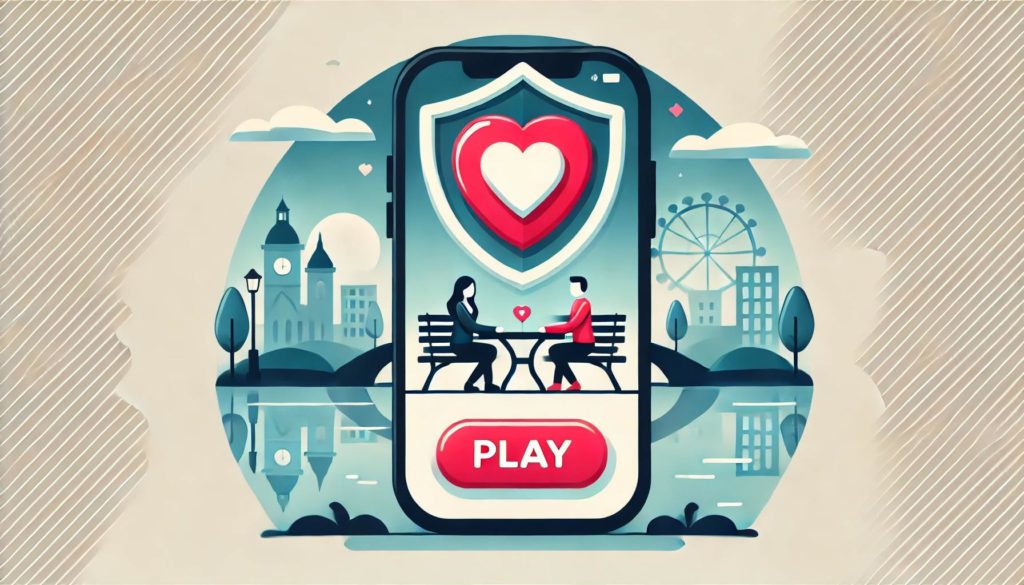
5. Protecting Human Moderators
With the focus on protecting users through content moderation, it’s important not to lose sight of other actors that need protection — in this case, human moderators. Most digital platforms still employ people to check content, especially in more tricky situations when automated content moderation needs human input for precision.
But AI-powered content moderation, nonetheless, has taken an immense burden off of human moderators. In the early days of moderation, it was all up to the people who had to manually go through every piece of user-generated content. The psychological harm that this can lead to is massive, including desensitizing, depression, and more.
Automated content moderation thus is central to protecting human moderators who, at the end of the day, have to make some tough decisions. However, they are no longer exposed to the most horrific content — because it is automatically removed by effective machine-learning algorithms.
Plus, when AI content moderation gets the heavy lifting done, people on the job can focus on tackling the truly complicated cases where human judgment is needed, rather than sifting through unimaginable amounts of harmful and explicit content.
6. Scalable Real-Time Monitoring
Scale and speed of content moderation might seem like factors that only concern the efficiency that businesses seek. But in this case, they are essential for dating platform users too — because they need real-time protection mechanisms that can handle huge amounts of data.
AI-powered content moderation solutions have developed significantly in the last decade. They are now able to process immensely large volumes of textual and visual information and to identify and remove unwanted and illegal content.
What’s more, only automated content moderation can provide the necessary speed of action to prevent the spreading of inappropriate content and dating users’ exposure to it — and 24/7 availability of protection. Visual content moderation powered by AI can analyze and filter out harmful content in real time, at any time, from images, videos, and, most impressively, live streams.
With the growing use of content moderation, machine learning algorithms get better and better. Their accuracy and ability to recognize nuances and different contexts improve — making it an indispensable element in the protection of dating platforms.
7. Positive User Experience
What makes for a positive user experience on a dating platform? It’s the feeling of being in a safe space, backed up by solid community standards and Trust and Safety protocols. It also entails that the dating app or site is consistent and predictable in its policies and actions.
The factors that contribute to people enjoying a dating platform are numerous, and of course, they include how much luck they have in striking meaningful conversations with matching partners. But besides the streak of luck, the focus is on this feeling of safety and of being cared for.
Protection from harmful content, fraud, spam, stalking, predatory behavior, minor abuse, as well as ensuring inclusivity, respect and safeguarding of dignity — all of these factors contribute to the positive experience of a person who has become a member of a dating platform in order to seek love and companionship.
This, in turn, helps solidify the brand reputation of a dating site or app, making it more popular and preferred by people — which in turn, grows the pool of potential candidates for matchmaking.
Imagga’s State-of-the-Art Content Moderation Solution
Imagga is a trusted partner for a wide array of digital businesses that want to provide a high level of protection for their online users through cutting-edge content moderation.
Our solution helps dating platforms, among others, follow their Trust and Safety regulations with state-of-the-art content moderation. Imagga’s platform monitors and automatically gets rid of harmful, illegal, and fraudulent content that can affect dating users — including images, videos, and live streams. It prevents exposure to content containing violence, drugs, hate crimes, not safe for work, weapons, and more.
Imagga has a dedicated adult image content moderation solution that comes in especially handy for dating platforms. The NSFW categorizer ranks content in three categories: NSFW, underware, and safe. We also boast custom model training that allows for tailoring models to your specific needs.
Integrating Imagga’s content moderation tools in your systems is seamless and quick. The deployment of our API is a clear process that allows you to provide effective user protection.
Try Out AI Content Moderation Today
Get started with AI content moderation and discover its numerous benefits for ensuring safety and privacy on your dating platform.
Get in touch with us to find out how you can easily integrate Imagga’s content moderation platform and offer safe dating experiences to users.
The Intersection of Visual Search and Social Media: New Avenues for User Engagement
Visual search is among the most popular uses of tech novelties that consumers can see and experience firsthand. It’s changing the ways in which we search for information and products online, make purchasing decisions, and share digital assets with peers.
Technology is often ruling the headlines these days, with new frontiers constantly being surpassed, especially in the field of Artificial Intelligence.
In a nutshell, visual search technology allows people to look for information online not on the basis of text queries, but by providing an image of the item they’re searching for. This is a tremendous change, as it shifts the focus from keyword-based queries and makes the process of searching online much more vigorous and flexible.
A major application of visual search that we can already see in motion is in the field of e-commerce businesses. With its help, users can easily upload a photo of the item they’re looking for — and get purchasing suggestions. This has been a major attracting force for younger customers — Gen Z and millennials — because of the convenience, ease of product discovery, and high level of personalization.
But AI visual search is being employed in other cases too: such as in search engines like Google, in websites, and more.
Then social media comes in the mix: how do visual search and social media join forces to boost user engagement?
Let’s get started with the basics first and go over the way visual search functions and where it’s already being used. Then we can dive into its intersection with social media and the exciting opportunities that it presents to both businesses and individuals.
What Is Visual Search?
Visual search entails performing an online search by using a visual query instead of a text-based one.
By employing AI, search engines are able to ‘understand’ the images and videos that users provide for their queries. Computer vision allows search engines to grasp the content, as well as the context, of the visuals in the blink of an eye — and then provide relevant visual and text content to the user.
The implications of visual search in terms of user experience are immense: it makes online searching a completely different and improved process. It brings a high level of flexibility and convenience to users, especially in cases when people can’t remember or define their query in text form.
Visual search has proven quite attractive for younger people who are highly tech-savvy and are used to operating with visuals in their everyday digital use. At the same time, the new technology is becoming prevalent and more and more people are discovering its perks — such as the speed of searching and ability to get much more robust results from visual queries.
But how exactly does visual search work? It’s all about AI, obviously, or more precisely — image recognition and computer vision. By using machine-learning algorithms, visual search engines are able to recognize and understand what an image contains.
Image recognition is, in fact, the powerhouse behind this capability. It allows the identification and categorization of objects, backgrounds, and various details about an image. The most interesting aspect of image recognition, being based on AI, is that it improves with use due to the use of machine-learning algorithms. The more images it processes, the better it becomes — and its accuracy and speed increase.
Let’s consider an example. When an image recognition platform processes images of clothes, for example, it examines each pixel and compares the new data with its previous knowledge gathered from identifying images of clothes. With each new image, it improves its ability to recognize colors, size, styling, and other details about clothes. Thus, through image recognition, the search engine is able to provide search results of similar clothes to the user’s query from its database.
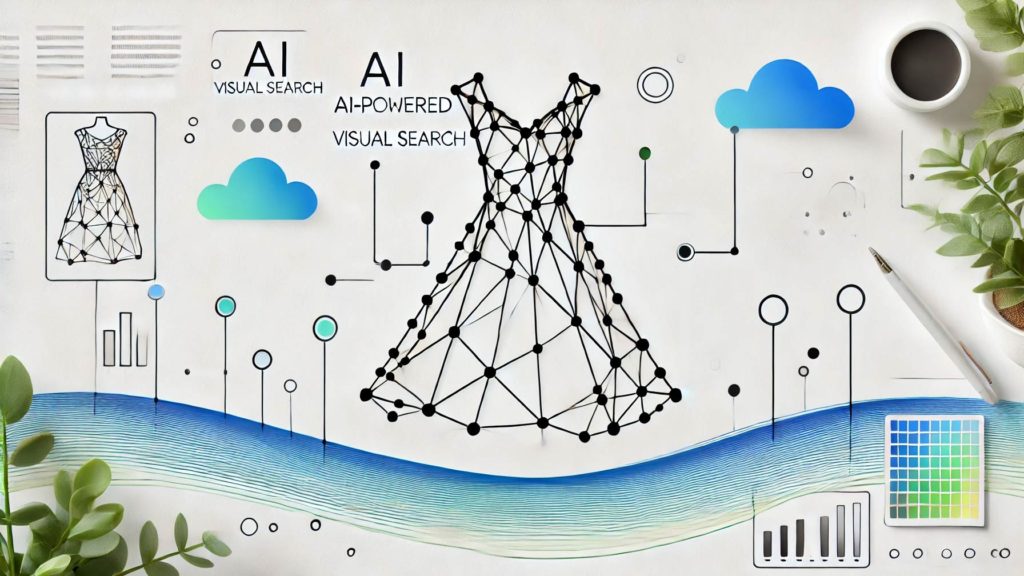
The Practical Uses of Visual Search Today
Visual search is already abundantly used online — from Google search, Amazon and Microsoft through a myriad of e-commerce websites to image-based social media platforms like Pinterest and Instagram.
The visual search functionality in Google is available through Google Lens. Users can select an image or a segment of a website to start their visual query. The search engine will yield results connected to the visual query in the form of relevant websites, images, products, and text content.
E-commerce companies are already using the opportunities of visual search extensively. Some of the fields in which it has become quite prominent include fashion and home decoration, among many others.
For example, users can provide a screenshot of a sofa they like — and the search engine of the e-commerce platform would provide them with similar products from its database. Or, users can upload an image of a fashion outfit they want to have, and they get matching suggestions for products they can buy immediately. Thus consumers can easily make their purchasing decision without a lengthy search process.
Visual Search and Social Media
At the intersection between social media and visual search, Pinterest has been a clear trailblazer. Being one of most popular visual social media, it has long been experimenting with the power of visual search — and has seen high levels of user engagement because of this.
Pinterest has been employing visual search capabilities since 2017, when it released
three visual search tools. With Pinterest Lens, users can take a photo with their phone’s camera and get related pins on the platform. Instant Ideas allows users to control the relevance of their Pinterest feed by selecting their most preferred pins and thus getting more accurate results in their feed. Last but not least, with the Shop the Look capability, Pinterest users can select items inside a pinned visual and search product listings to find that item for purchase.
While Instagram does not support reverse image search, when combined with Google Lens, it does provide a powerful visual search combo. On Instagram, you can perform a visual search on a computer with a right-click, which makes a Google search on the basis of the selected image.
In this respect, it’s worth mentioning that Google Lens has brought an immense advancement in visual search overall, particularly in terms of how social media and visual search interact. While Pinterest was the main force with its three visual search tools, Google Lens has been key in making visual search popular in a general sense. Beyond e-commerce and shopping, with Google Lens users also get quick and easy access to QR code identification, item recognition (like identifying plants and animals), location identification to provide business spots, reviews and more, and even translation of street signs and restaurant menus on the basis of photos made on the go.
Bing Visual Search, Snapchat Camera Search, and Amazon StyleSnap are just a few other examples of prominent uses of visual search at the cross-section of social media, search engines, and e-commerce.
Boosting User Engagement by Searching Visually
Visual search has clearly revolutionized the way people are interacting with social media platforms — and both brands and digital marketers are trying to catch up with the technological advancements.
It’s apparent that the only way forward is through visuals — and companies from all industries are aiming to adapt to this new digital reality. In order to stay ahead of the competition, brands can tailor to the specificities of visual search so they can provide seamless, intuitive, and personalized experiences to online users.
The main goal for businesses that want to grow their user base and engage with existing and future customers is proper optimization of content for visual search — both on social media and beyond.
In the case of visual social media platforms like Instagram and Pinterest, the optimization process for brands entails inclusion of appropriate hashtags and using the features for visual search to expand the company’s presence. Products can be included in single social media posts and promoted for better visibility — and the images used can then be included in various visual search queries.
But there’s much more that can be done to unleash the potential of visual search. For example, brands can put effort in creating high-quality visual content that is both visually pleasing and informative — including infographics, product visuals, videos, and much more. These visual assets can then be shared on social media where they can reach a wider audience through the visual search capabilities and thus engage more users. Visual search also opens up numerous new possibilities for influencer marketing, especially for fashion and home decoration brands.
Brand discovery can also be boosted through visual search optimization of the company website. More specifically, brands can bet on good images in optimal size and format, accurate image titles, adequately written alt texts, and informative descriptions and captions for CMS platforms. Using geotagging and long-tail keywords for images are also recommended techniques.
All of these aspects would allow for better search engine indexation. In turn, the more a brand’s visual content is understood by visual search engines, the higher would the discoverability of its products and engagement with end users.
Use the Power of Image Recognition with Imagga
At Imagga, we are helping businesses in various industries reap the benefits of AI-powered image recognition. Our solutions offer unprecedented accuracy and flexibility in image categorization, tagging, and visual search, among others.
Get in touch today to explore Imagga’s robust image recognition solutions and see how we can help your business grow.
The Image Recognition Market is Growing
Self-driving cars, face detection, social media content moderation — it’s already difficult to imagine our world without image recognition powered by Artificial Intelligence. Digital image processing has become an essential element of the operations and progress for companies across industries, locations, and sizes — and for each of us as individuals, too. The Image recognition market is rapidly growing!
In the last decade, the AI image recognition market, based on ever-evolving machine learning algorithms with growing accuracy, is making impressive steps. In 2023, the global market was evaluated at $53.29 billion. The projections are that it will reach 12.5% compound annual growth rate until 2030 — to hit the impressive $128.28 billion.
But what is driving this massive development?
It’s the perfect storm of diverse factors: major technological advancements, availability of funding, the ubiquity of mobile devices and cameras, and last but not least, shifts in consumer expectations about interactive visual user experience.
The result is that numerous big industries have already implemented image recognition in various contexts — from retail, ecommerce, media and entertainment to automobile, transportation, manufacturing and security. The prospects are that adoption of image recognition will continue on a massive scale across verticals.
In the sections below, we go over the basics about image recognition, the growth statistics and projections, and the industries driving the change, as well as Imagga’s pioneering role in the field.
The Basics About Image Recognition and Computer Vision
Before we delve into the numbers about the image recognition market growth, let’s get some definitions about the technology — and the nuances involved in image recognition and computer vision and their place within the broader fields of Artificial Intelligence and machine learning.
While the two terms are very close and sometimes even used interchangeably, there are some differences to note.
Image recognition entails the identification and categorization of visual content, including objects, text, people, actions, colors and other attributes within an image or photo. The typical actions that can be executed with the help of image recognition tools include image tagging, image classification, detection and localization, as well as feature identification and extraction. They’re often powered by technology called convolutional neural networks (CNNs).
Computer vision, on the other hand, is a broader term. It includes not only image recognition, but also the process of grasping context and gathering data from visuals. The main techniques in AI computer vision include object detection, image classification, and image segmentation, as well as progressive new ones like generation of visuals, restoration, and 3D vision.
The Numbers Behind the AI Image Recognition Market Growth
What stands behind the $53.29 billion global market value of image recognition? Let’s delve into the findings of Maximize Market Research’s AI Image Recognition Market- Forecast and Analysis (2023-2029) and of Grand View Research’s Image Recognition Market Size, Share & Trends Analysis Report covering the period 2017-2030.
Naturally, the biggest share is North America, which made up 34.8% of the market in 2022 because of cloud-based streaming services’ expansion in the U.S. It also has the highest growth momentum at the moment, compared to Europe, Asia Pacific, Latin America, and the Middle East and Africa.
The most quickly growing market is Asia Pacific, with a compound annual growth rate of 16.7% until 2030. The driving forces there include increased use of mobile devices and online streaming, as well as security cloud solutions and ecommerce. As for Europe, the projections until 2030 are promising major growth too, fueled by technological advancements in obstacle-detection for automobiles.
The regional market penetration is the highest in North America, followed by Europe and Asia Pacific.
In terms of field of application, the five main areas where image recognition has a major role include marketing and advertising (biggest share), followed by scanning and imaging, security, visual search, and augmented reality (with the smallest share).
As for segments, the facial recognition one was the most prominent in 2023, driven by the increased security needs in the banking, retail and government sectors. In the next few years, the sectors projected to be the main driving forces for the image recognition market growth are marketing and advertising, as well as the service segment. In marketing and advertising, the need for improved user experience and better ad placement are key. As for the service segment, customization of image recognition tools for specific business needs is central.
The main techniques in wide use include recognition of faces, objects, barcode and QR, patterns, and optical characters. In 2022, facial recognition accounted for 22.1% of the image recognition market, driven by the growing requirements for safety and security. Pattern recognition is expected to grow significantly, with its use in areas like detection of anomalies, recommendation systems, and more.
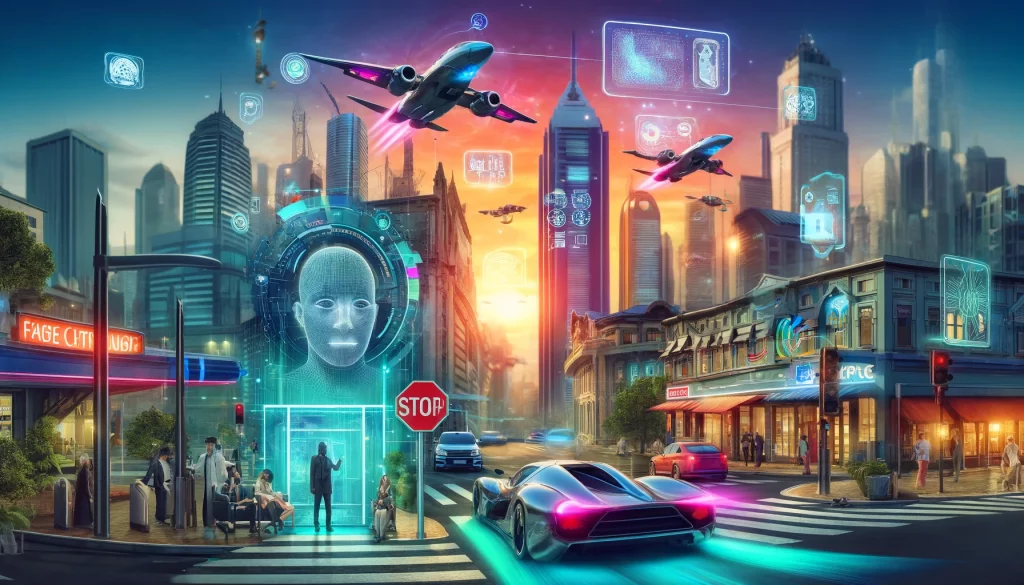
Industries and Verticals Driving the Expansion
The expansion of the image recognition market is driven by the technological adoption in a couple of key industries and verticals, as observed in the Image Recognition Market Size, Share & Trends Analysis Report by Grand View Research and the AI Image Recognition Market- Forecast and Analysis (2023-2029) by Maximize Market Research.
The major ones include media and entertainment, retail and ecommerce, banking, financial services and insurance (BFSI), automobile and transportation, telecom & IT, government, and healthcare, as well as education, gaming, and aerospace and security.
In the last few years, the retail and ecommerce segment had the biggest market share of more than 22%. This was largely due to the use of image recognition in online shopping, through which customers can search for items by making a photo of their desired object, shop the look, and similar functionalities. They have found wide adoption in fashion, furniture and other ecommerce sections.
The other major segments were media and entertainment, followed by banking, financial services and insurance where image recognition is widely used for ensuring security and identification, and automobile and transportation, driven by the use of image recognition in self-driving vehicles.
In 2023, the revenue share was the biggest in retail and ecommerce, while the lowest was in healthcare. As for the forecast for the compound annual growth rate until 2030, the banking, financial services and insurance segment has the lowest projection, while healthcare has the highest.
Imagga: A Pioneer and Major Player on the Image Recognition Arena
Imagga has been working on cutting edge AI technology in the field of image recognition for more than 14 years now. We’re proud to be one of the pioneers and to continue our inventive approach to automating visual tasks for businesses across industries. We’re also happy to be on the list of key players in the field in the AI Image Recognition Market- Forecast and Analysis report by Maximize Market Research, alongside IBM, Amazon, Google, Microsoft, and more.
Our product portfolio is constantly expanding and currently includes technology that powers up image and video understanding, content moderation, and pre-trained computer vision machine learning models.
Imagga’s image recognition technology is packaged in easy-to-integrate APIs. They can be easily rolled out in the cloud or on premise, depending on the customer’s needs. We also offer custom model training which is essential for building custom models tailored to the specificities of the client’s data.
Imagga’s mission is to provide companies with the most powerful tools to get the greatest value from their visual content. We help businesses improve their workflows, employ automation of visual tasks, and gain deeper insights into their visual data.
The Exponential Development of Content Moderation
Image recognition is at the core of AI content moderation — a particular use of visual processing and identification that has gained significant traction in recent years.
With the unseen growth of social platforms and websites, as well as ecommerce and retail digital channels, the amount of user generated content posted online every second is simply unimaginable. But not all content is appropriate, legal, or safe from harm. That’s why many different types of online businesses have turned to content moderation — so they can provide user-friendly and safe platforms for shopping and socializing to their customers.
Automated content moderation has brought a major change in the field. It has a high rate of efficiency and protects human content moderators from being exposed to horrific visual content on a daily basis. In a nutshell, AI content moderation offers visual content screening and filtering at scale, full legal compliance, protection of content moderators, multilingual moderation, and an unseen level of productivity.
While the most known field where content moderation is king is social media, its uses are numerous. Dating platforms, travel booking websites, gaming platforms, all types of online marketplaces and ecommerce, fashion apps and websites, video platforms, forums and communities, educational platforms, networking websites, and messaging apps — this is just a short list of the applications that content moderation has today.
Unleash the Power of Image Recognition for Your Business
With its solid pioneering role in image recognition, Imagga has been the trusted partner of all types of companies in integrating the capabilities of machine learning algorithms in their workflows.
Want to learn how you can boost your business performance and tools with image recognition? Get in touch with us to explore our ready and tailor-made solutions for image tagging, categorization, visual search, face recognition, and more.