Image Recognition Trends for 2025 and Beyond
What lies ahead in 2025 for image recognition and its wide application in content moderation?
In this blog post, we delve into the most prominent trends that will shape the development of AI-driven image recognition and its use in content moderation in the coming years, both in terms of technological advancements and responding to perceived challenges.
Image recognition is at the heart of effective content moderation, and is also the basis of key other technological applications such as self-driving vehicles and facial recognition for social media and for security and safety. It’s no wonder that the global image recognition market is growing exponentially. In 2024 it was valued at $46.7 billion and is projected to grow to $98.6 billion by 2029.
As a myriad of practical uses of AI across the board explode, including AI video generation, deepfake, and synthetic data model training, content moderation will become more challenging — and will have to rely on the evolution of image recognition algorithms. At the same time, the plethora of issues around AI ethics and biases, paired with the more stringent content moderation regulations on national and international level, will drive a significant growth in image recognition’s power and scope.
Without further ado, here are the nine trends we believe will shape the path of image recognition and content moderation in 2025.
AI Video Generation Goes Wild
AI-powered video generation started going public in 2022, with a bunch of AI video generators now available to end users. Platforms like Runway, Synthesia, Pika Labs, Descript and more are already being used by millions of people to create generative videos, turn scripts into videos, and edit and polish video material. It’s safe to say AI video generation is becoming mainstream.
In 2025, we can expect this trend to set off massive changes in how videos are being created, consumed, and moderated. As generative AI tools make giant leaps, AI video generators will become even more abundant and more precise in creating synthetic videos that mimic life. Their use will permeate all digital channels that we frequent and will become more visible in commercials and art forms like films.
At the same time, the implications of these changes for the film industry and for visual and cinema artists will continue to raise questions about ownership and copyright infringements, as well as about the value of human creativity vs. AI generation.
Real-Time Moderation for Videos and Live Streaming
AI-powered image recognition is already at the heart of real-time moderation of complex content like videos and especially live streaming. It allows platforms to ensure a safe environment for their users, whether it’s social media, dating apps, or e-commerce websites.
As we move forward into 2025, we can expect real-time moderation to become ever more sophisticated to match the growing complexity and diversity of live video streaming. AI moderation systems will grow their capabilities in terms of contextual analysis needed to grasp nuances and tone, as well as in terms of multimodal moderation of video, audio and text within livestreams. They will also get better at predicting problematic content in streams.
Moderation is also likely to follow the pattern of personalization that’s abundant in social media and digital communications. Platforms may start allowing users to choose their own moderation settings, such as levels for profanity and the like.
Multi-Modal Foundational AI Models
Multi-modality is becoming a necessity in understanding different data types at the same time, including visual and textual. They combine visual, audio, text and sensor data to grasp the full spectrum and context of content. Multi-modal foundational AI models are advanced systems that are able to put together different data inputs into one in order to bring out deeper levels of meaning and help decision-making processes in various settings.
In 2025, multi-modal AI models will grow, integrating many different types of data for all-round understanding. Cross-modality understanding will thus gradually become the norm for AI interactions that will grow to resemble human ones.
Multi-modal AI models will bring about new applications in robotics, Virtual Reality and Augmented Reality, accessibility tools, search engines, and even virtual assistants. They will enable more comprehensive interactions with AI that are closer to human experience.
Deepfake and AI-generated Content Detection
The spread of deepfake and AI-generated content is no longer a prediction for the future. Deepfakes are all around us and while they might be entertaining to watch when it comes to celebrities and favorite films, their misuse in representing politicians, for example, is especially threatening. Discerning between authentic and AI-generated content is becoming more and more complex, since AI becomes better at it.
As people find it harder to tell the truth from fiction, detection of AI-generated content and deepfakes will evolve to meet the demand — the AI vs. AI battle. AI-powered detection and verification tools will become more sophisticated and will provide real-time identification, helping people differentiate content on the web in order to prevent fraud, scams, and misuse.
We can expect to see the development of universal standards for detection and marking of AI-generated content and media, as well as databases with deepfakes that will help identification of fraudulent content. As the issue circulates in the public's attention, people will also get better at identifying fake materials — and will have the right tools to do so.
AI-Driven Contextual Understanding
Comprehensive understanding of content, including context, tone, and intent, is still challenging for AI, but that is bound to change. We expect AI to get even better at discerning the purpose and tone of content — grasping nuances, humor, and satire, as well as harmful intentions.
Contextual understanding will evolve to improve the accuracy of AI systems, as well as our trust in them. We’ll see more and more multi-modal context integration that enables all-round understanding, including intent and nuances detection. Cultural specificities will also come into play, as AI models are already embedding cultural contexts and differences.
In 2025, we can also expect AI to develop further in spotting emotions and impact in content, getting closer to our human perception of data. Developments in contextual understanding will be driven by AI learning on-the-go from our changing trends in language and social norms.
Use of Generative AI and Synthetic Data for Models’ Training
AI models’ training relies on large amounts of data — which are often scarce or difficult to obtain for a variety of reasons, including copyright and ethical concerns.
Generative AI and synthetic data are already fueling models’ training, and we can expect to see more of that in the coming years. For example, synthetic data for content moderation is already in use, bringing about benefits like augmentation and de-biasing of datasets. However, it still requires in-depth supervision and input from real data.
Synthetic data will advance, creating accurate and hyper-realistic datasets for training. They will continue to help reduce bias in real-world datasets, plus will offer a way to scale and develop models that are working in more specific contexts.
AI-Human Hybrid Content Moderation
Content moderation is the norm in various digital settings, from social media and dating apps to e-commerce and gaming. Its development has been fueled by the application of AI-driven image recognition across the board — and this trend will continue, combining multi-modality and context understanding advancements.
At the same time, the AI-human hybrid content moderation is likely to stay around, especially when it comes to moderation of sensitive and culturally complex content. The speed and scale that AI offers in content moderation, particularly in live streaming and video, will continue to be paired with human review for accuracy and nuance detection.
Betting on the hybrid approach will make content moderation achieve even greater efficiency and balancing, accounting for ethical and moral considerations as they appear. As AI trains on human decisions, its ability to discern complex dilemmas will also evolve.
More Stringent Content Moderation Regulations
Legal requirements for content moderation are tightening, making digital platforms more accountable for the content they circulate and allow users to publish. As technology evolves, various threats evolve too — and national and international regulatory bodies are trying to catch up by adopting new legislation and enforcement methods.
In particular, the most important recent content moderation regulations bringing about higher transparency and accountability requirements include the EU’s Digital Services Act (DSA), fully enforced in February 2024, and the General Data Protection Regulation (GDPR) which is in action from 2018. In the USA, this is Section 230 of the Communications Decency Act, while in the UK — Online Safety Bill that was enforced at the end of 2023. The EU’s AI Act will come into effect in 2025, and in full force — in 2026.
We can expect the stricter legal requirements trend to continue in the coming years, as countries struggle to create an intentional framework for handling harmful content. AI accountability, fairness, transparency, and moral and ethical oversight will be certainly on the list of legislators’ demands.
Ethics and Bias Mitigation
As stricter regulations come into force in 2025, like the EU’s AI Act, people will demand more comprehensive ethical and bias mitigation. This is becoming especially important in the context of security surveillance based on facial recognition, as well as in biases in AI generative models.
Along with legal requirements, we’ll see new ethical frameworks for AI and image recognition in particular being developed and applied, which might even evolve into ethical certifications. Besides fair use, inclusivity and diversity will also become focal points that will be included in ethical guidelines. All of this will require the efforts of experts from the AI, law, and ethics fields.
Bias mitigation will rely on diversifying datasets for model training, be it real-life or synthetic. The goal will be to decrease biases based on gender, race, or cultural differences, which can also be helped by automated bias auditing systems and by real-time bias detection.
Discover the Power of Image Recognition for Your Business
Imagga has been a trailblazer in the image recognition field for more than a decade. We have become the preferred tech partner of companies across industries, empowering businesses to make the most of cutting-edge AI technology in image recognition.
Get in touch to find out how our image recognition tools — including image tagging, facial recognition, visual search, and adult content detection — can help your business evolve, stay on top of image recognition trends, and meet industry standards.
A Comprehensive Guide to Content Moderation Regulations
The digital world is expanding and transforming at an unprecedented pace. Social media platforms now serve billions, and new channels for user-generated content emerge constantly, from live-streaming services to collaborative workspaces and niche forums. This rapid growth has also made it easier for harmful, illegal, or misleading content to spread widely and quickly, with real-world consequences for individuals, businesses, and society at large.
In response, governments and regulatory bodies worldwide are stepping up their scrutiny and tightening requirements around content moderation. New laws and stricter enforcement aim to address issues like hate speech, misinformation, online harassment, and child protection. The goal is to create safer digital spaces, but the impact on platform operators is profound - those who fail to implement effective moderation strategies risk heavy fines and serious reputational damage.
If your business hosts any form of user-generated content – whether posts, images, videos, or posts – you can’t afford to ignore these developments. This article will guide you through the major content moderation regulations shaping today’s digital environment. Knowing these regulations is essential to protect both your platform and its users in a world where the expectations and penalties for content moderation have never been higher.
Content Moderation and The Need for Regulations
In essence, content moderation entails the monitoring of user-generated content, so that hate speech, misinformation, harmful and explicit content can be effectively tackled before reaching users. Today the process is typically executed as a mix between automated content moderation powered by AI and input from human moderators on sensitive and contentious issues.
While effective content moderation depends on tech companies, different regulatory bodies across the world have taken on to create legal frameworks. They set the standards and guide the moderation measures that social media and digital platforms have to apply. Major cases of harmful content mishandling have led to these regulatory actions by national and multinational authorities.
However, getting the rules right is not an easy feat. Regulations have to balance between protecting society from dangerous and illegal content while preserving the basic right of free expression and allowing online communities to thrive.
The Major Content Moderation Regulations Around the World
It’s challenging to impose content moderation rules worldwide, so a number of national and international bodies have created regulations that apply to their territories.
European Union: DSA and GDPR
The European Union has introduced two main digital regulation acts: the Digital Services Act (DSA) that came in full force in February 2024 and the General Data Protection Regulation (GDPR) applied since 2018.
The purpose of the DSA is to provide a comprehensive framework for compliance that digital platforms have to adhere to. It requires online platforms and businesses to provide a sufficient level of transparency and accountability to their users and to legislators, as well as to protect users from harmful content specifically.
Some specific examples include requirements for algorithm transparency, content reporting options for users, statements of reasons for content removal, options to challenge content moderation decisions, and more. In case of non-compliance with the DSA, companies can face penalties of up to 6% of their global annual turnover.
The GDPR, on the other hand, targets data protection and privacy. Its goal is to regulate the way companies deal with user data in general, and in particular during the process of content moderation.
USA: Section 230 of the Communications Decency Act
The USA has its own piece of legislation that addresses the challenges in content moderation. This is Section 230 of the Communications Decency Act. It makes it possible for online platforms to moderate content, as well as taking away from them the liability for user-generated content.
At the same time, Section 230 is currently under fire for not being able to address the recent developments in the digital world, and in particular generative AI. There are worries that it would be used by companies developing generative AI to escape legal responsibilities for potential harmful effects of their products.
UK: Online Safety Bill
At the end of 2023, the Online Safety Bill came into force in the United Kingdom after years in the making. Its main goal is to protect minors from harmful and explicit content and to make technological companies take charge of content on their platforms.
In particular, the Bill requires social media and online platforms to be responsible for removing content containing child abuse, sexual violence, self-harm, illegal drugs, weapons, cyber-flashing, and more. They should also require age verification where applicable.
A number of other countries have enforced national rules for content moderation that apply to online businesses, including European countries like Germany but also others like Australia, Canada, and India.
Challenges of Content Moderation Regulations
The difficulties of creating and enforcing content moderation regulations are manyfold.
The major challenge is the intricate balancing act between protecting people from harmful content and at the same time, upholding freedom of speech and expression and people’s privacy. When rules become too strict, they threaten to suffocate public and private communication online — and to overly monitor and regulate it. On the other hand, without clear content moderation regulations, it’s up to companies and their internal policies to provide a sufficient level of protection for their users.
This makes the process of drafting content moderation regulations complex and time consuming. Regulatory bodies have to pass the legal texts through numerous stages of consultations with different actors, including consumer representative groups, digital businesses, and various other stakeholders. Finding a solution that is fair to parties with divergent interests is challenging, and more often than not, lawmakers end up in situations of compliance rejection and lawsuits.
A clear example of this conundrum is the recently enacted Online Safety Bill in the UK. It aims to make tech companies more responsible for the content that gets published on their digital channels and platforms. Even though its purpose is clear and commendable — to protect children online — the bill is still contentious.
One of the Online Safety Bill requirements is especially controversial, as it requires messaging platforms to screen user communication for child abuse material. WhatsApp, Signal, and iMessage have declared that they cannot comply with that rule without hurting the end-to-end encryption of user communication, which is meant to protect privacy. The privacy-focused email platform Proton has expressed a strong opinion against this rule, too, since it allows government interference in and screening of private communication.
The UK’s Online Safety Bill is just one example of many content moderation regulations that have come under fire from different interest groups. In general, laws in this area are complex to draft and enforce — especially given the constantly evolving technology and the respective concerns that come up.
The Responsibility of Tech Companies
Technology businesses have a lot on their plate in relation to content moderation. There are both legal and ethical considerations, having in mind that a number of online platforms cater mostly to minors and young people, who are the most vulnerable.
The main compliance requirements for digital companies vary across different territories, which makes it difficult for international businesses to navigate them. In the general case, they include child protection, harmful and illegal content removal, and disinformation and fraud protection, but every country or territory can and does enforce additional requirements. For example, the EU also includes content moderation transparency, content removal reports, and moderation decisions.
Major social media platforms have had difficult moments in handling content moderation in recent years. One example is the reaction to the COVID-19 misinformation during the pandemic. YouTube enforced a strict strategy for removing videos but was accused of censorship. In other cases, like the Rohingya genocide in Myanmar, Facebook was accused of not taking enough steps to moderate content in conflict zones, thus contributing to violence.
There have been other long-standing disagreements about social media moderation, and nudity is a particularly difficult topic. Instagram and Facebook have been attacked for censorship of artistic female nudity and of breastfeeding. At the same time, Snapchat has been accused of its AI bot, which is producing adult-containing text in its communication with minors. It’s more than obvious that striking the right balance is tough for tech companies, as it is for regulators.
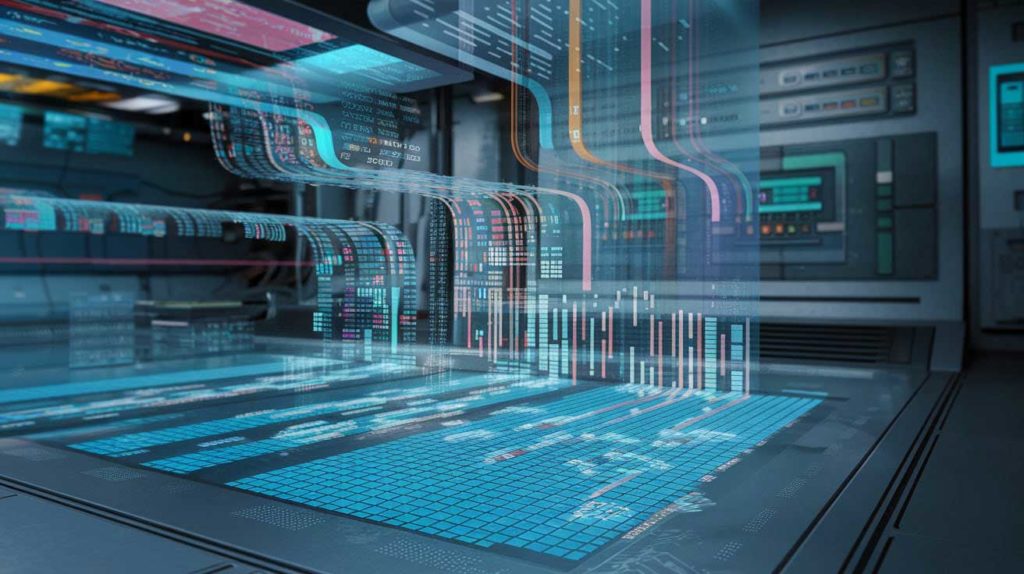
Emerging Trends in Content Moderation Regulations
Content regulation laws and policies need to evolve constantly to address the changing technological landscape. A clear illustration of this is the rise of generative AI and the lack of a legal framework to handle it. While the EU’s Digital Services Act came into force recently, it was drafted before generative AI exploded — so it doesn’t particularly address its new challenges. The new AI Act will partially come into force in 2025 and fully in 2026, but the tech advancements by then might already make it insufficient.
The clear trend in content moderation laws is towards increased monitoring and accountability of technological companies with the goal of user protection, especially of vulnerable groups like children and minorities. New regulations focus on the accountability and transparency of digital platforms to their users and to the regulating bodies. This is attuned to societal expectations for national and international regulators to step into the game — and exercise control over tech companies. At the same time, court rulings and precedents will also shape the regulatory landscape, as business and consumer groups challenge in court certain aspects of the new rules.
In the race to catch up with new technology and its implications, global synchronisation of content moderation standards will likely be a solution that regulators will seek. It would require international cooperation and cross-border enforcement. This may be good news for digital companies because they would have to deal with unified rules, rather than having to adhere to different regulations in different territories.
It is likely that these trends will continue while seeking a balance with censorship and privacy concerns. For digital companies, the regulatory shifts will certainly have an impact on operations. While it’s difficult to know what potential laws and policies might be, the direction towards user protection is clear. The wisest course of action is thus to focus on creating safe and fair digital spaces while keeping up-to-date with developments in the regulatory framework locally and internationally.
Best Practices for Regulatory Compliance
The complex reality of content moderation and its regulations today is that it requires digital companies to pay special attention and dedicate resources.
In particular, it’s a good idea for businesses to:
- Use the most advanced content moderation tools to ensure user protection
- Create and enforce community guidelines, as well as Trust and Safety protocols
- Stay informed about developments in the content moderation regulations landscape
- Be transparent and clear with users regarding your content moderation policies
- Have special training and information sessions for their teams on the regulatory framework and its application in internal rules
- Collaborate with other companies, regulators, and interest groups in the devising of new regulations
- Have legal expertise internally or as an external service
All in all, putting user safety and privacy first has become a priority for digital businesses in their efforts to meet ethical and legal requirements worldwide.
AI in Waste Management: How Image Recognition Can Help Make Our World Cleaner
The practical applications of AI in waste management is one of the surprising use cases. Image recognition powered by AI is, in fact, already being explored as a tool for effective waste management. Many prototypes have been developed around the world, and some are gradually being deployed in practice. Waste detection and separation with the help of image recognition is still an activity driven by scientific researchers and remains yet to become a massive practice.
Let’s review how image recognition can assist in revolutionizing waste detection and sorting to achieve Zero Waste — helping humanity deal with the negative impacts of trash by turning it into usable resources that drive Industry 4.0.
The Global Issues in Waste Management Today
Exponentially increasing amounts of trash, pollution, risk of diseases, and economic losses — the aftereffects of improper waste management are numerous.
Despite technological advancements across the board, handling waste is one of the major issues in our modern world. According to the UNEP Global Waste Management Outlook 2024 report, municipal solid waste is bound to grow from 2.3 billion tonnes in 2023 to 3.8 billion tonnes by 2050. The global waste management cost in 2020 was $252 billion, but when taking into account the costs of pollution, poor health, and climate change, it’s estimated at $361 billion. The projected costs for 2050 are $640.3 billion unless waste management is rapidly revolutionized. All of this affects people on the whole planet, and as usual, its adverse effects are bigger in poorer areas.
Finding effective waste management solutions is important on a number of levels. Naturally, the issue of protecting people’s health is primary, as well as nature preservation — especially given the major problem with plastic waste that is cluttering our planet’s oceans. Beyond handling pollution and health issues, proper waste handling can also lead to adequate recycling and reusing of resources, as well as bring direct economic benefit to our societies. The UNEP Global Waste Management Outlook 2024 report points out that applying a circular economy model containing waste avoidance and sustainable business practices, as well as a complete waste management approach, can lead to a financial gain of USD 108.5 billion per year.
While there are advanced waste management systems around the world, a practical and affordable solution that can be applied in different locations across the globe has not yet been discovered. This is especially relevant for the major task of trash separation of biological, plastic, glass, metal, paper, and other materials for recycling, as well as for dangerous waste detection that safeguards people’s health. This is where image recognition powered by Artificial Intelligence can step in.
Image Recognition for Waste Detection and Sorting
The applications of AI in waste management are growing, and the main approach that fuels this growth is the use of image recognition based on machine learning algorithms. With the help of convolutional neural networks (CNNs), waste management tools can find patterns in the analyzed visual data of trash, propelling the development of intelligent waste identification and recycling (IWIR) systems.
Use of Image Recognition in Waste Management
The main tasks of image recognition in waste management are identification of recyclable materials, waste classification, detection of toxic waste, and trash pollution detection. In addition, image recognition solutions for waste management have to offer a high degree of customization and flexibility to tailor to the difference between regions. For example, fine-tuning for different areas with specific trash images is essential. Training the algorithms with waste items particular to the specific demographic region is crucial for effective classification.
The goal of waste management systems powered by image recognition is to be able to identify and classify different types of trash. They are being trained with large image datasets, so that they can afterwards predict and spot hazardous trash and differentiate between different types of recyclable materials. In some cases, prototypes are made with robotic platforms that use sensors and microcontroller boards for accurate detection and processing, while in others, mobile phone terminals are being used.
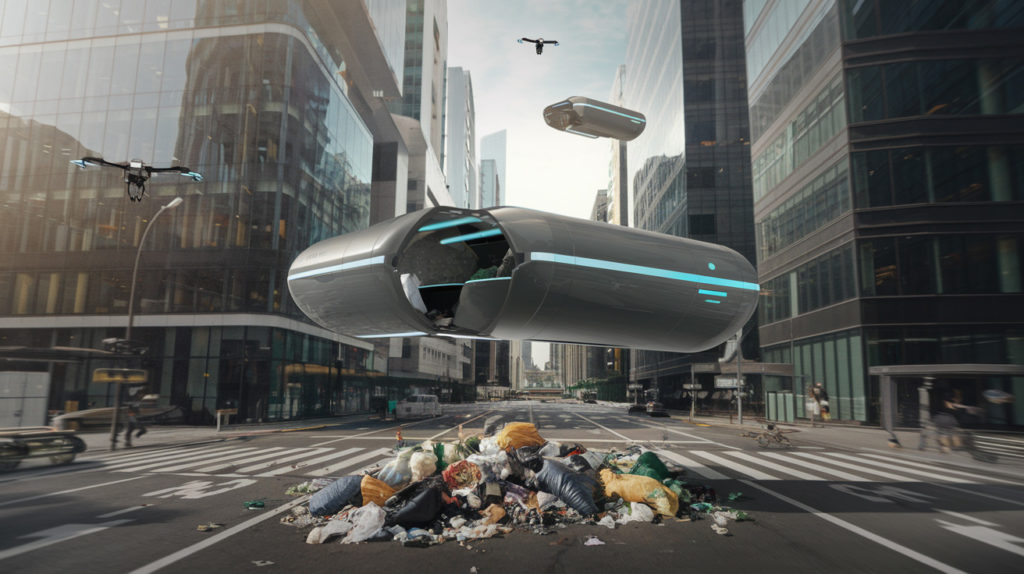
The Future Possibilities and Benefits
The use of image recognition in waste management, while developing rapidly, is yet to become widespread, but the possibilities and benefits it offers are highly promising.
The automatization of recyclable material sorting can have an immense impact on environmental sustainability, helping resource conservation and reduction of greenhouse gas emissions due to new production. It can increase the identification and use of valuable materials in trash, as well as reduce overall waste.
The shift in waste management with image recognition can lead to meaningful changes in waste management jobs. Image classification technology would save people from doing dangerous and dirty jobs, while ast the same time creating new opportunities in technology and data analysis.
Awareness about recycling and greater access to recycling services can grow as a result of image recognition-powered waste management systems. If recycling is more efficient and widespread, even communities with limited resources would get access to recycling options, as well as information about the benefits of recycling practices in daily life.
Use Case: Image Recognition in Waste Management
At Imagga, we do our best to push the boundaries of what’s possible with image recognition — and waste management is an area that we’ve already been exploring.
Already in 2016, we had a pilot trial with the Seoul National University, the most prestigious South Korean research university, on a project for waste management with image recognition capabilities. A team from a couple of departments at the University, led by Prof. Seong-Woo Kim and Dr. Daeun Kim, started the pilot project Fun with Trash to create a system that makes trash recycling easy and effective.
The prototype built by the team relied on a smart sensor system to identify the different types of recyclable trash that are being deposited in the different recycle bins (plastic, paper, glass, and metal). Once a person is about to throw a piece of trash, a camera sensor captures an image, and then the system recognizes the type of trash and compares it to a database of images. Then, the right bin is opened to deposit the trash.
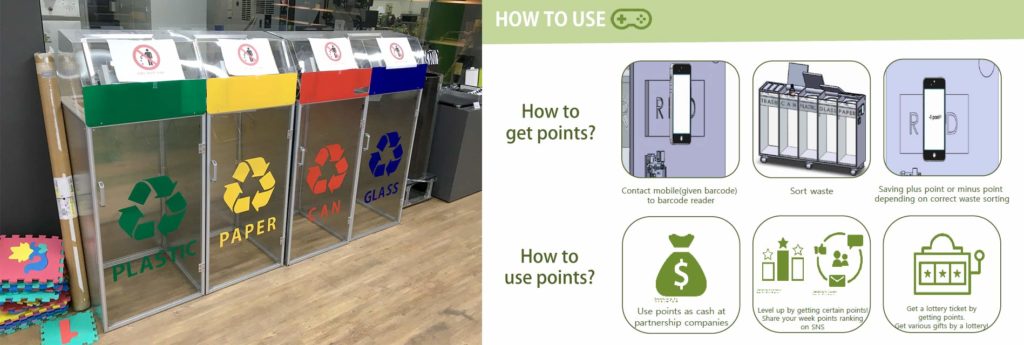
Imagga jumped on board to help the SNU team with the image recognition technology to power up the recycling machine prototype. Our team trained the custom classifier for waste categorization and helped with improving its accuracy, reaching precision rates of 95%. Our experts also advised on the sensor positioning and illumination of the hardware setup.
Learn How Image Recognition Can Power Up Your Waste Management System
Imagga’s robust image recognition platform holds a huge potential for waste management systems due to our custom model training. In the context of waste management, custom model training is essential to provide the necessary flexibility to work in different demographic areas.
Our customizable tool allows you to define the categories in which you want to classify visuals so that the classification system is tailored to your particular needs. First, specific datasets are selected, and then we build a deep learning classification model trained with the particular categories and data. Afterward, our image categorization API does the rest of the job by fully automating the image categorization process.
Want to learn more about how image recognition can fuel the efficiency of waste management systems? Get in touch with us.
FAQ
AI-powered image recognition systems are used to automatically identify and sort different types of waste by analyzing images or video streams of waste items. Cameras installed on conveyor belts or smart bins capture visuals, and AI models classify items into categories such as plastic, metal, paper, or organic waste. This automation improves sorting accuracy and efficiency compared to manual processes.
Yes, to an extent. Advanced AI models can detect visual cues like contamination from food residue, grease, or liquids. This helps flag items that may be unsuitable for recycling. However, the accuracy depends on the quality of the training data and imaging setup. It is an area where ongoing improvements are being made to enhance precision.
One of the main challenges of using AI for image recognition in waste management is the high variability in how waste materials appear—items can be crushed, soiled, or partially obscured, making accurate identification difficult. Additionally, there’s often a lack of sufficient training data for uncommon or region-specific waste types, which limits the model’s performance. Waste facilities can also be harsh environments for sensitive hardware, posing durability concerns for cameras and sensors. While the long-term benefits are significant, the initial investment in equipment, AI model development, and system integration can be costly. Finally, ensuring the AI system works seamlessly with existing sorting infrastructure and operational workflows requires careful planning and customization.
High-quality training data in the context of waste recognition refers to a large, diverse, and accurately labeled set of images representing different types of waste materials in various conditions - clean, dirty, crushed, partially visible, and under different lighting or backgrounds. This kind of dataset enables AI models to learn the subtle differences between materials like plastics, metals, paper, and organics, even when they don’t look perfect. If the training data is limited, biased, or poorly labeled, the model’s performance in real-world scenarios can drop significantly, leading to misclassification, lower recycling efficiency, and even costly system errors. Simply put, the accuracy and reliability of an AI waste recognition system depend heavily on the quality of the data it learns from.
Not likely. While it might perform decently in controlled conditions, an AI model trained only on clean, idealized images of waste will struggle when faced with the unpredictable reality of actual waste streams—where items are dirty, overlapping, torn, or obscured. Real-world waste doesn’t arrive neatly sorted or photogenic. To build robust recognition systems, training data must reflect that messiness. This includes variations in lighting, camera angles, and the visual noise of mixed materials. Otherwise, you risk building an AI that excels in the lab but fails in the field - what works in a dataset won't always work on a conveyor belt.
A Detailed Guide on Content Moderation for Trust & Safety
Ensuring a safe digital environment has become a top priority for forward-looking companies in the dynamically changing online landscape.
Trust and Safety (T&S) programs are the essential building blocks of these efforts — both in order to deliver the necessary protection for users and to comply with local and global safety rules and regulations.
Content moderation is one of the main and most powerful methods within Trust and Safety company policies. It ensures that all user-generated content published and distributed on a digital platform or app has passed a check for its appropriateness and safety. Moderation has become an indispensable tool for businesses of all venues — from social media and gaming to dating and media.
But content moderation doesn’t come without its challenges, including large volumes of content to be reviewed, balancing between moderation and free expression, and misuse of AI technologies, among others.
Different moderation methods offer different advantages and disadvantages, and below we take a look at how the various approaches can be used and combined — to achieve a company’s Trust & Safety objectives in the most effective way.
The Content Moderation Challenges that Trust and Safety Teams Face
Trust and Safety teams, whether in-house or external, are entrusted with a challenging task. They have to make the digital channels and platforms of a business safe and trustworthy for its customers by establishing and running highly effective T&S processes — while at the same time delivering on ROI expectations.
No pressure at all!
T&S teams have to shape and run a T&S program that identifies and manages risks that can negatively impact users and their experience with a brand. The programs have to be quite comprehensive so that they can ensure a safe and comfortable environment where customers can achieve their goals and feel at ease. This is how people’s trust in the brand can be enhanced — setting the ground for long-lasting relationships with customers.
Most importantly, T&S policies have to protect users from any kind of abuse while also adhering to safety and privacy rules applicable at local and international levels. And content moderation is the key to achieving both.
All of this sounds straightforward, but it is certainly not an easy feat. The challenges of getting content moderation right are numerous — and have different contexts and specifics.
Volume
First, there’s the volume. The amount of user-generated content that has to be sifted through is enormous — and it’s not only text and static images, but also includes more and more videos and live streams.
Striking a balance between moderation and censorship
Then there’s the delicate balance between removing harmful content, protecting free speech and expression, and avoiding bias while ensuring a great user experience. This complex balancing act involves both ethical and practical considerations that account for legal requirements, cultural specificities, and company goals — all at the same time.
Regulations
Naturally, legal compliance is a challenge on its own. Safety rules and regulations keep evolving along with new technology, and the EU’s Digital Services Act (DSA), the UK Online Safety Act, and Australia’s Online Safety Act are some of the prominent examples in this respect. Content moderation efforts have to be fully in tune with the latest regulatory activity — to ensure full protection for users and no liability for companies.
Generative AI content
Last but not least, there’s generative AI. While AI is powering content moderation, on the other side are deepfake, misinformation and fraud. Voice cloning and deepfake videos are a major threat to a safe online environment, and they create a pervasive sense that nothing can be trusted anymore. As it becomes more and more difficult to spot what’s genuine and what’s fabricated content, content moderation efforts have to keep up.
The Pros and Cons of the Different Content Moderation Approaches
While the present and future of content moderation are tightly linked to technology and automation, there are different approaches — and each of them has its benefits.
Currently, the most employed approach is hybrid, as it combines the best of manual human moderation and full automation. But let’s go briefly through each of the approaches.
Manual Moderation
In the first days of content moderation, it was all up to human moderators to clean up harmful and illegal content. This seems like madness from today’s point of view because people who did the job were exposed to the most horrific content. The growing amounts of user-generated content were unmanageable. The process was harmful, slow, and ineffective.
Luckily, these days are gone — but human input remains important for the nuanced and balanced content moderation of many online platforms.
Automated Moderation
The development of AI created the possibility of automating content moderation, and this has certainly proved to be a big breakthrough in the field. Automation allows for the processing of huge amounts of text and visual data, as well as the real-time moderation of complex content like live streams. Automated moderation is very good at identifying and removing content that is clearly illegal, explicit, or spam.
Naturally, automation has its downfalls. While precision has dramatically improved since the early days of AI content moderation, social and cultural nuances and contexts can still be challenging.
Hybrid Moderation
The hybrid approach puts together the best of both worlds — reaping the power of AI automation that provides scale and efficiency, while adding the precision and subtlety that human moderation allows for.
The combination provides for a constant balance between the productivity of technology and the social veracity and accuracy that only people can provide. The moderation tools mark content that is not straightforwardly inacceptable — and then it undergoes human review.
With continuous use, machine learning algorithms get better and better. The input from human moderators helps the AI platform develop a better understanding of more delicate elements in content, as well as their cultural meanings. The amount of content that gets processed also helps the platform learn and improve.
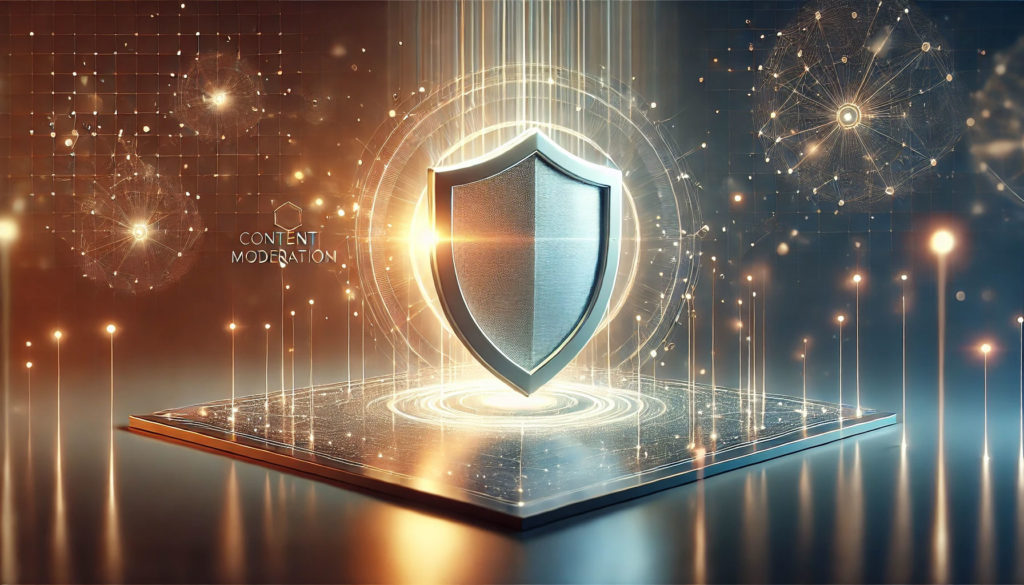
Buy vs. Build а Content Moderation Solution
Besides the different content moderation approaches, Trust & Safety teams have two main options for AI content moderation that they can choose from. They may decide to develop in-house content moderation tools or to use third-party vendors — also known as the build-or-buy dilemma.
Each option has its benefits and challenges — and the choice should be tailored to the particular needs of the company and its Trust & Safety team.
In-House
The path of creating in-house content moderation solutions is seen as giving the highest level of ownership over the tool and ability to craft it according to the specific business needs. However, it is certainly the most labor-intensive one and requires significant internal expertise in the field.
More specifically, companies have to add to their teams experts in advanced machine learning and AI, AI model training and optimization, and image and video processing. They also have to ensure the necessary infrastructure and resources, which entails computational power and data management. Last but not least, a major factor are the high development costs involved in creating an in-house moderation platform, as well as the lengthy time-to-market of the solution.
While building an in-house content moderation system might seem like the only way to maintain control and customization within the company, this path poses substantial challenges, especially for companies lacking expertise in image recognition and AI model training.
The in-house option usually makes the most sense for companies that are involved in digital security, Trust and Safety, and similar fields.
Third-Party Providers
With the growth and development of content moderation platforms, the option to use third-party vendors has become popular for many companies of all sizes.
Content moderation platform providers are top specialists in the field, employing the most cutting-edge AI content moderation tools. Since their focus is on building the best possible moderation platforms, they have the know-how and bandwidth to keep up with technological advancements, legal requirements, and usability expectations.
Using third-party content moderation providers ensures a high level of expertise and efficiency in the moderation process, as well as a guarantee for staying on top of digital and legal threats, but ownership of the moderation tool is not with the business. However, vendors provide solid options for data protection and privacy, as well as a high level of flexibility in terms of customization and features.
Introducing Imagga’s Robust Content Moderation Solution
Imagga has been developing AI-powered content moderation tools for more than a decade — and the results are impressive.
Our state-of-the-art platform identifies and automatically removes illegal and harmful content in images, videos, or live streams — including adult content, violence, drugs, hate, and weapons, among others. It boasts eight classification and detection models that target different types of unwanted content. The tool is also equipped to detect AI-generated visuals so that users can be warned about fabricated or fake content and protected from fraud and hate speech.
Packed with all these capabilities, Imagga’s content moderation platform provides a robust tool for Trust and Safety teams to get their job done in an easier and faster way.
Rolling out Imagga in your systems is a straightforward process. You can easily deploy the content moderation API and start using it in no time.
In case you want to make a hybrid mix between automatic AI content moderation and human input for subtleties, you can use our AI Mode hybrid visual content moderation platform. It allows for the seamless coordination and connection between automation that allows large-scale processing and human moderation for precision and nuances.
Get Started with AI Content Moderation Today
Ready to explore how AI content moderation can boost your Trust and Safety program? Get in touch today to learn how you can seamlessly integrate Imagga’s content moderation solution in your workflow.
Content Moderation for Dating Sites - Key Benefits
Online dating platforms have to be a safe harbor for people looking for romance and honest human connections — or else they cannot serve their purpose. But the dangers posed by various malicious actors like scammers and catfishers are numerous.
Protection has thus become a high priority for dating sites and apps in their efforts to ensure a safe environment for their users, to shield their brand reputation, and to increase the satisfaction with their products.
Content moderation based on AI is a powerful ally of dating platforms in their battle with harmful content, scams, and fraud. Besides user privacy and safety, content moderation for dating sites is the key to scalable and immediate filtering, enforcing age restrictions, and protecting human moderators from exposure to harmful content.
In the visual age of our digital world today, especially important is the robust AI-based visual content moderation. It is the only way to perform powerful and accurate real-time monitoring of images, videos and live streams.
Below you can find the seven top ways in which automated AI content moderation helps dating platforms protect their users and create thriving and safe online communities.
1. Dealing with Inappropriate Content
Harmful, illegal, and offensive content is ubiquitous online — and dating platforms are a primary target for malicious actors that want to distribute such content. It is often included in user profiles, and of course, in private messaging between users.
Having to constantly deal with irrelevant, abusive or explicit content is not optimal for people who turn to a platform to seek companionship and romance. In fact, it is a primary factor in dropping out of an online community — which is exactly the opposite of what dating platforms aim for. Inclusivity and safety are thus of primary concern.
Content moderation solutions based on AI tackle the big issue of inappropriate and harmful content with growing efficiency, accuracy and speed. Powered by constantly evolving machine learning algorithms, these solutions automatically filter out the content that is categorically not fit for the platform. They also flag content that is dubious — which then gets reviewed by human moderators to balance out protection and freedom of expression.
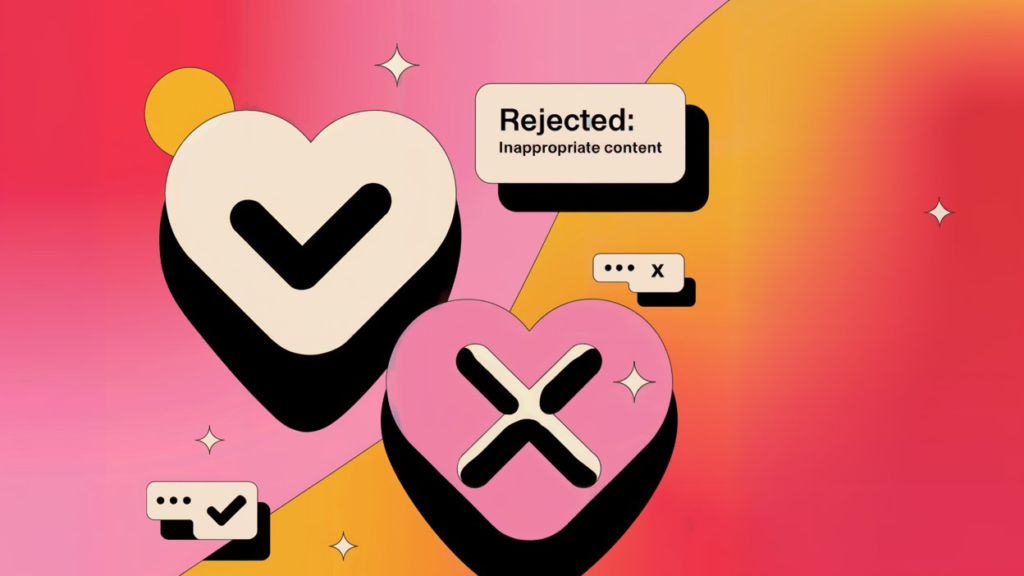
2. Scam and Fraud Prevention
Looking for love — and getting scammed instead. This is a classic scenario that many people either experience or are afraid of, and for a good reason. Dating platform users need, more than ever, reassurance that they are communicating online with real people with honest intentions.
With the help of AI content moderation, platforms are now able to provide this reassurance and protection. Checking the authenticity of user profiles can be automated with scanning of profile photos and analysis of profile information and patterns of behavior that point to scam and fraud.
Content moderation also provides effective monitoring for catfishing and bot activities. It allows platforms to minimize unwanted promotions, spam messaging, ads, money requests, and harmful links sharing, among other inappropriate content.
The AI-powered verification of profile authenticity has become an essential way to provide a safe and trustworthy environment where people can relax and focus on meaningful exchanges with potential soulmates.
3. User Privacy and Safety First
Ensuring a safe online environment is crucial for dating platforms, with user privacy and safety at the core. Private communications may lead users to share sensitive data like addresses or bank details.
Content moderation can block the sharing of such data, protecting users from harm. It also monitors for stalking and abusive behavior, allowing platforms to act proactively rather than relying solely on user reports. Additionally, moderation can offer personalized safety options, enabling users to set stricter protection filters if desired.
4. Safeguarding Minors
Enforcing age restrictions is a must for dating platforms, since they are targeted at individuals that are not minors. But checking the age of each and every user — especially when minors may try to conceal their age — can be a daunting task.
Content moderation comes in handy in this situation as well. Visual moderation helps analyze the images shared by users to assess their actual age and crosscheck it with the information they are sharing. In case the visual materials show a minor, the platform will be able to apply the age restrictions effectively.
The protection of minors is also active in in-app communication, where content moderation provides monitoring for harmful content sharing, abuse, and other potential dangers for younger users who have illegally gained access to the platform.
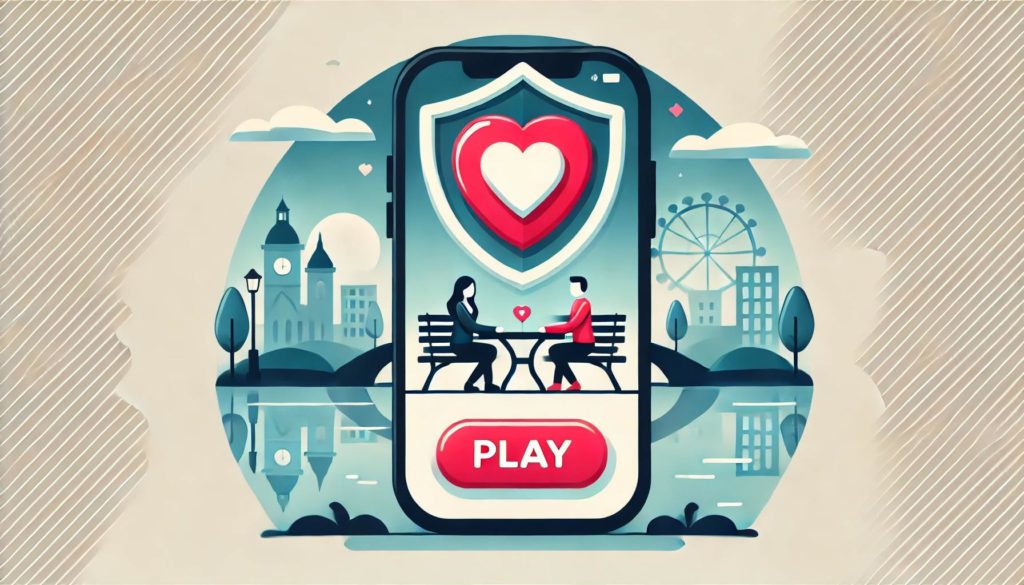
5. Protecting Human Moderators
With the focus on protecting users through content moderation, it’s important not to lose sight of other actors that need protection — in this case, human moderators. Most digital platforms still employ people to check content, especially in more tricky situations when automated content moderation needs human input for precision.
But AI-powered content moderation, nonetheless, has taken an immense burden off of human moderators. In the early days of moderation, it was all up to the people who had to manually go through every piece of user-generated content. The psychological harm that this can lead to is massive, including desensitizing, depression, and more.
Automated content moderation thus is central to protecting human moderators who, at the end of the day, have to make some tough decisions. However, they are no longer exposed to the most horrific content — because it is automatically removed by effective machine-learning algorithms.
Plus, when AI content moderation gets the heavy lifting done, people on the job can focus on tackling the truly complicated cases where human judgment is needed, rather than sifting through unimaginable amounts of harmful and explicit content.
6. Scalable Real-Time Monitoring
Scale and speed of content moderation might seem like factors that only concern the efficiency that businesses seek. But in this case, they are essential for dating platform users too — because they need real-time protection mechanisms that can handle huge amounts of data.
AI-powered content moderation solutions have developed significantly in the last decade. They are now able to process immensely large volumes of textual and visual information and to identify and remove unwanted and illegal content.
What’s more, only automated content moderation can provide the necessary speed of action to prevent the spreading of inappropriate content and dating users’ exposure to it — and 24/7 availability of protection. Visual content moderation powered by AI can analyze and filter out harmful content in real time, at any time, from images, videos, and, most impressively, live streams.
With the growing use of content moderation, machine learning algorithms get better and better. Their accuracy and ability to recognize nuances and different contexts improve — making it an indispensable element in the protection of dating platforms.
7. Positive User Experience
What makes for a positive user experience on a dating platform? It’s the feeling of being in a safe space, backed up by solid community standards and Trust and Safety protocols. It also entails that the dating app or site is consistent and predictable in its policies and actions.
The factors that contribute to people enjoying a dating platform are numerous, and of course, they include how much luck they have in striking meaningful conversations with matching partners. But besides the streak of luck, the focus is on this feeling of safety and of being cared for.
Protection from harmful content, fraud, spam, stalking, predatory behavior, minor abuse, as well as ensuring inclusivity, respect and safeguarding of dignity — all of these factors contribute to the positive experience of a person who has become a member of a dating platform in order to seek love and companionship.
This, in turn, helps solidify the brand reputation of a dating site or app, making it more popular and preferred by people — which in turn, grows the pool of potential candidates for matchmaking.
How to Overcome the Common Challenges in Moderating Dating Platforms
Content moderation for dating sites is a complex task that goes beyond standard content moderation practices. These platforms deal with unique challenges due to the personal and emotional nature of user interactions, global reach, and the sensitive data involved. To maintain a safe and welcoming environment, dating platforms need to address several common challenges effectively. Below are key obstacles and strategies to overcome them.
Read how Imagga helped a leading dating platform to transform its content moderation.
False positives and negatives in moderation for dating sites
False positives flag legitimate content as inappropriate, while false negatives let harmful content go undetected, both harming user trust and experience. On dating platforms, false positives may remove genuine profiles or messages, frustrating users, while false negatives allow harmful behavior like harassment or scams. Advanced AI trained for dating conversations can address this by recognizing context, such as playful banter versus harassment. Feedback mechanisms for user appeals provide a second review, further improving accuracy.
Diverse cultural norms in global dating platforms
Dating sites serve users from diverse cultural backgrounds, where norms and expectations vary widely. What’s acceptable in one culture might be offensive in another.
To address this, platforms can implement localized moderation rules and employ diverse moderators with multilingual support. Engaging with user communities to understand their preferences ensures a more culturally inclusive environment.
Automation and human oversight balance for sensitive decisions
Automation is essential for managing large volumes of content efficiently, but it can struggle with nuanced or context-heavy situations. Sensitive decisions, such as identifying harassment or policy violations, often require human judgment.
Imagga’s State-of-the-Art Content Moderation Solution
Our solution helps dating platforms, among others, follow their Trust and Safety regulations with state-of-the-art content moderation. Imagga’s platform monitors and automatically gets rid of harmful, illegal, and fraudulent content that can affect dating users — including images, videos, and live streams. It prevents exposure to content containing violence, drugs, hate crimes, not safe for work, weapons, and more.
Imagga has a dedicated adult image content moderation solution that comes in especially handy for dating platforms. The NSFW categorizer ranks content in three categories: NSFW, underware, and safe. We also boast custom model training that allows for tailoring models to your specific needs.
Integrating Imagga’s content moderation tools in your systems is seamless and quick. The deployment of our API is a clear process that allows you to provide effective user protection.
When a user reports harassment, the platform reviews it using automated tools and human moderators. If guidelines are violated, actions like warnings, suspensions, or bans are taken. Reporting users may receive updates, ensuring transparency and trust.
To ensure privacy and security during the moderation process, platforms use encrypted systems to handle and store user data, limiting access to authorized personnel only. Moderators are trained to follow strict confidentiality protocols, and sensitive information is anonymized wherever possible. Additionally, platforms comply with data protection regulations like GDPR or CCPA to safeguard user privacy at every step.
Platforms verify user age by implementing measures such as requiring users to provide their date of birth during sign-up and using document verification or AI tools to analyze identification documents when necessary. Some platforms also employ AI-driven age estimation technology to flag potential underage users, ensuring compliance with regulations and preventing minors from accessing adult content.
Platforms balance expression and safety with clear guidelines that allow diverse opinions while prohibiting harmful content like hate speech or threats. Automated tools and human reviewers enforce rules fairly, addressing harmful behavior without silencing respectful discourse. Transparent policies build trust and foster a safe, inclusive environment.
The Intersection of Visual Search and Social Media: New Avenues for User Engagement
Visual search is among the most popular uses of tech novelties that consumers can see and experience firsthand. It’s changing the ways in which we search for information and products online, make purchasing decisions, and share digital assets with peers.
Technology is often ruling the headlines these days, with new frontiers constantly being surpassed, especially in the field of Artificial Intelligence.
In a nutshell, visual search technology allows people to look for information online not on the basis of text queries, but by providing an image of the item they’re searching for. This is a tremendous change, as it shifts the focus from keyword-based queries and makes the process of searching online much more vigorous and flexible.
A major application of visual search that we can already see in motion is in the field of e-commerce businesses. With its help, users can easily upload a photo of the item they’re looking for — and get purchasing suggestions. This has been a major attracting force for younger customers — Gen Z and millennials — because of the convenience, ease of product discovery, and high level of personalization.
But AI visual search is being employed in other cases too: such as in search engines like Google, in websites, and more.
Then social media comes in the mix: how do visual search and social media join forces to boost user engagement?
Let’s get started with the basics first and go over the way visual search functions and where it’s already being used. Then we can dive into its intersection with social media and the exciting opportunities that it presents to both businesses and individuals.
What Is Visual Search?
Visual search entails performing an online search by using a visual query instead of a text-based one.
By employing AI, search engines are able to ‘understand’ the images and videos that users provide for their queries. Computer vision allows search engines to grasp the content, as well as the context, of the visuals in the blink of an eye — and then provide relevant visual and text content to the user.
The implications of visual search in terms of user experience are immense: it makes online searching a completely different and improved process. It brings a high level of flexibility and convenience to users, especially in cases when people can’t remember or define their query in text form.
Visual search has proven quite attractive for younger people who are highly tech-savvy and are used to operating with visuals in their everyday digital use. At the same time, the new technology is becoming prevalent and more and more people are discovering its perks — such as the speed of searching and ability to get much more robust results from visual queries.
But how exactly does visual search work? It’s all about AI, obviously, or more precisely — image recognition and computer vision. By using machine-learning algorithms, visual search engines are able to recognize and understand what an image contains.
Image recognition is, in fact, the powerhouse behind this capability. It allows the identification and categorization of objects, backgrounds, and various details about an image. The most interesting aspect of image recognition, being based on AI, is that it improves with use due to the use of machine-learning algorithms. The more images it processes, the better it becomes — and its accuracy and speed increase.
Let’s consider an example. When an image recognition platform processes images of clothes, for example, it examines each pixel and compares the new data with its previous knowledge gathered from identifying images of clothes. With each new image, it improves its ability to recognize colors, size, styling, and other details about clothes. Thus, through image recognition, the search engine is able to provide search results of similar clothes to the user’s query from its database.
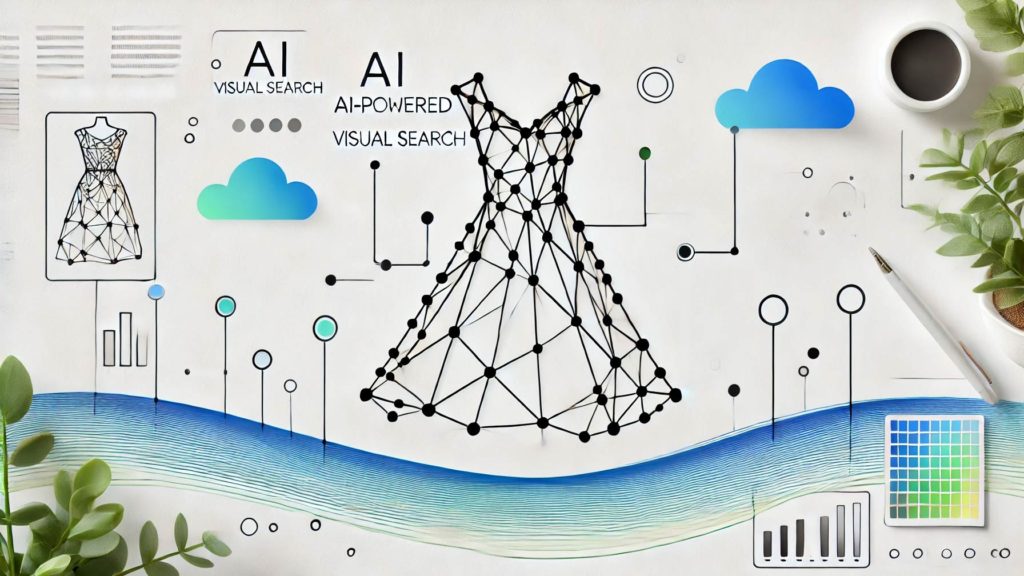
The Practical Uses of Visual Search Today
Visual search is already abundantly used online — from Google search, Amazon and Microsoft through a myriad of e-commerce websites to image-based social media platforms like Pinterest and Instagram.
The visual search functionality in Google is available through Google Lens. Users can select an image or a segment of a website to start their visual query. The search engine will yield results connected to the visual query in the form of relevant websites, images, products, and text content.
E-commerce companies are already using the opportunities of visual search extensively. Some of the fields in which it has become quite prominent include fashion and home decoration, among many others.
For example, users can provide a screenshot of a sofa they like — and the search engine of the e-commerce platform would provide them with similar products from its database. Or, users can upload an image of a fashion outfit they want to have, and they get matching suggestions for products they can buy immediately. Thus consumers can easily make their purchasing decision without a lengthy search process.
Visual Search and Social Media
At the intersection between social media and visual search, Pinterest has been a clear trailblazer. Being one of most popular visual social media, it has long been experimenting with the power of visual search — and has seen high levels of user engagement because of this.
Pinterest has been employing visual search capabilities since 2017, when it released
three visual search tools. With Pinterest Lens, users can take a photo with their phone’s camera and get related pins on the platform. Instant Ideas allows users to control the relevance of their Pinterest feed by selecting their most preferred pins and thus getting more accurate results in their feed. Last but not least, with the Shop the Look capability, Pinterest users can select items inside a pinned visual and search product listings to find that item for purchase.
While Instagram does not support reverse image search, when combined with Google Lens, it does provide a powerful visual search combo. On Instagram, you can perform a visual search on a computer with a right-click, which makes a Google search on the basis of the selected image.
In this respect, it’s worth mentioning that Google Lens has brought an immense advancement in visual search overall, particularly in terms of how social media and visual search interact. While Pinterest was the main force with its three visual search tools, Google Lens has been key in making visual search popular in a general sense. Beyond e-commerce and shopping, with Google Lens users also get quick and easy access to QR code identification, item recognition (like identifying plants and animals), location identification to provide business spots, reviews and more, and even translation of street signs and restaurant menus on the basis of photos made on the go.
Bing Visual Search, Snapchat Camera Search, and Amazon StyleSnap are just a few other examples of prominent uses of visual search at the cross-section of social media, search engines, and e-commerce.
Boosting User Engagement by Searching Visually
Visual search has clearly revolutionized the way people are interacting with social media platforms — and both brands and digital marketers are trying to catch up with the technological advancements.
It’s apparent that the only way forward is through visuals — and companies from all industries are aiming to adapt to this new digital reality. In order to stay ahead of the competition, brands can tailor to the specificities of visual search so they can provide seamless, intuitive, and personalized experiences to online users.
The main goal for businesses that want to grow their user base and engage with existing and future customers is proper optimization of content for visual search — both on social media and beyond.
In the case of visual social media platforms like Instagram and Pinterest, the optimization process for brands entails inclusion of appropriate hashtags and using the features for visual search to expand the company’s presence. Products can be included in single social media posts and promoted for better visibility — and the images used can then be included in various visual search queries.
But there’s much more that can be done to unleash the potential of visual search. For example, brands can put effort in creating high-quality visual content that is both visually pleasing and informative — including infographics, product visuals, videos, and much more. These visual assets can then be shared on social media where they can reach a wider audience through the visual search capabilities and thus engage more users. Visual search also opens up numerous new possibilities for influencer marketing, especially for fashion and home decoration brands.
Brand discovery can also be boosted through visual search optimization of the company website. More specifically, brands can bet on good images in optimal size and format, accurate image titles, adequately written alt texts, and informative descriptions and captions for CMS platforms. Using geotagging and long-tail keywords for images are also recommended techniques.
All of these aspects would allow for better search engine indexation. In turn, the more a brand’s visual content is understood by visual search engines, the higher would the discoverability of its products and engagement with end users.
Use the Power of Image Recognition with Imagga
At Imagga, we are helping businesses in various industries reap the benefits of AI-powered image recognition. Our solutions offer unprecedented accuracy and flexibility in image categorization, tagging, and visual search, among others.
Get in touch today to explore Imagga’s robust image recognition solutions and see how we can help your business grow.
The Image Recognition Market is Growing
Self-driving cars, face detection, social media content moderation — it’s already difficult to imagine our world without image recognition powered by Artificial Intelligence. Digital image processing has become an essential element of the operations and progress for companies across industries, locations, and sizes — and for each of us as individuals, too. The Image recognition market is rapidly growing!
In the last decade, the AI image recognition market, based on ever-evolving machine learning algorithms with growing accuracy, is making impressive steps. In 2023, the global market was evaluated at $53.29 billion. The projections are that it will reach 12.5% compound annual growth rate until 2030 — to hit the impressive $128.28 billion.
But what is driving this massive development?
It’s the perfect storm of diverse factors: major technological advancements, availability of funding, the ubiquity of mobile devices and cameras, and last but not least, shifts in consumer expectations about interactive visual user experience.
The result is that numerous big industries have already implemented image recognition in various contexts — from retail, ecommerce, media and entertainment to automobile, transportation, manufacturing and security. The prospects are that adoption of image recognition will continue on a massive scale across verticals.
In the sections below, we go over the basics about image recognition, the growth statistics and projections, and the industries driving the change, as well as Imagga’s pioneering role in the field.
The Basics About Image Recognition and Computer Vision
Before we delve into the numbers about the image recognition market growth, let’s get some definitions about the technology — and the nuances involved in image recognition and computer vision and their place within the broader fields of Artificial Intelligence and machine learning.
While the two terms are very close and sometimes even used interchangeably, there are some differences to note.
Image recognition entails the identification and categorization of visual content, including objects, text, people, actions, colors and other attributes within an image or photo. The typical actions that can be executed with the help of image recognition tools include image tagging, image classification, detection and localization, as well as feature identification and extraction. They’re often powered by technology called convolutional neural networks (CNNs).
Computer vision, on the other hand, is a broader term. It includes not only image recognition, but also the process of grasping context and gathering data from visuals. The main techniques in AI computer vision include object detection, image classification, and image segmentation, as well as progressive new ones like generation of visuals, restoration, and 3D vision.
The Numbers Behind the AI Image Recognition Market Growth
What stands behind the $53.29 billion global market value of image recognition? Let’s delve into the findings of Maximize Market Research’s AI Image Recognition Market- Forecast and Analysis (2023-2029) and of Grand View Research’s Image Recognition Market Size, Share & Trends Analysis Report covering the period 2017-2030.
Naturally, the biggest share is North America, which made up 34.8% of the market in 2022 because of cloud-based streaming services’ expansion in the U.S. It also has the highest growth momentum at the moment, compared to Europe, Asia Pacific, Latin America, and the Middle East and Africa.
The most quickly growing market is Asia Pacific, with a compound annual growth rate of 16.7% until 2030. The driving forces there include increased use of mobile devices and online streaming, as well as security cloud solutions and ecommerce. As for Europe, the projections until 2030 are promising major growth too, fueled by technological advancements in obstacle-detection for automobiles.
The regional market penetration is the highest in North America, followed by Europe and Asia Pacific.
In terms of field of application, the five main areas where image recognition has a major role include marketing and advertising (biggest share), followed by scanning and imaging, security, visual search, and augmented reality (with the smallest share).
As for segments, the facial recognition one was the most prominent in 2023, driven by the increased security needs in the banking, retail and government sectors. In the next few years, the sectors projected to be the main driving forces for the image recognition market growth are marketing and advertising, as well as the service segment. In marketing and advertising, the need for improved user experience and better ad placement are key. As for the service segment, customization of image recognition tools for specific business needs is central.
The main techniques in wide use include recognition of faces, objects, barcode and QR, patterns, and optical characters. In 2022, facial recognition accounted for 22.1% of the image recognition market, driven by the growing requirements for safety and security. Pattern recognition is expected to grow significantly, with its use in areas like detection of anomalies, recommendation systems, and more.
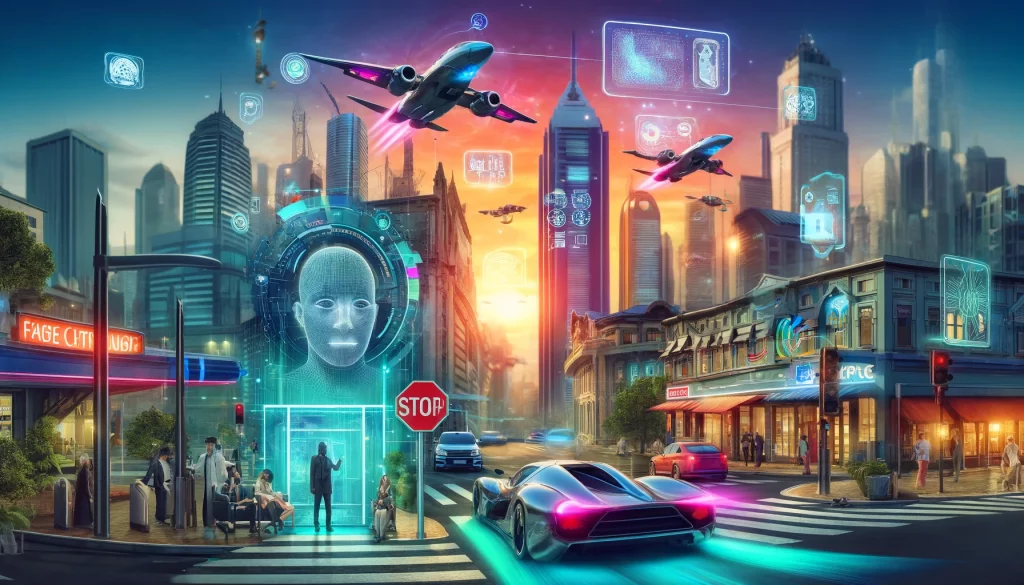
Industries and Verticals Driving the Expansion
The expansion of the image recognition market is driven by the technological adoption in a couple of key industries and verticals, as observed in the Image Recognition Market Size, Share & Trends Analysis Report by Grand View Research and the AI Image Recognition Market- Forecast and Analysis (2023-2029) by Maximize Market Research.
The major ones include media and entertainment, retail and ecommerce, banking, financial services and insurance (BFSI), automobile and transportation, telecom & IT, government, and healthcare, as well as education, gaming, and aerospace and security.
In the last few years, the retail and ecommerce segment had the biggest market share of more than 22%. This was largely due to the use of image recognition in online shopping, through which customers can search for items by making a photo of their desired object, shop the look, and similar functionalities. They have found wide adoption in fashion, furniture and other ecommerce sections.
The other major segments were media and entertainment, followed by banking, financial services and insurance where image recognition is widely used for ensuring security and identification, and automobile and transportation, driven by the use of image recognition in self-driving vehicles.
In 2023, the revenue share was the biggest in retail and ecommerce, while the lowest was in healthcare. As for the forecast for the compound annual growth rate until 2030, the banking, financial services and insurance segment has the lowest projection, while healthcare has the highest.
Imagga: A Pioneer and Major Player on the Image Recognition Arena
Imagga has been working on cutting edge AI technology in the field of image recognition for more than 14 years now. We’re proud to be one of the pioneers and to continue our inventive approach to automating visual tasks for businesses across industries. We’re also happy to be on the list of key players in the field in the AI Image Recognition Market- Forecast and Analysis report by Maximize Market Research, alongside IBM, Amazon, Google, Microsoft, and more.
Our product portfolio is constantly expanding and currently includes technology that powers up image and video understanding, content moderation, and pre-trained computer vision machine learning models.
Imagga’s image recognition technology is packaged in easy-to-integrate APIs. They can be easily rolled out in the cloud or on premise, depending on the customer’s needs. We also offer custom model training which is essential for building custom models tailored to the specificities of the client’s data.
Imagga’s mission is to provide companies with the most powerful tools to get the greatest value from their visual content. We help businesses improve their workflows, employ automation of visual tasks, and gain deeper insights into their visual data.
The Exponential Development of Content Moderation
Image recognition is at the core of AI content moderation — a particular use of visual processing and identification that has gained significant traction in recent years.
With the unseen growth of social platforms and websites, as well as ecommerce and retail digital channels, the amount of user generated content posted online every second is simply unimaginable. But not all content is appropriate, legal, or safe from harm. That’s why many different types of online businesses have turned to content moderation — so they can provide user-friendly and safe platforms for shopping and socializing to their customers.
Automated content moderation has brought a major change in the field. It has a high rate of efficiency and protects human content moderators from being exposed to horrific visual content on a daily basis. In a nutshell, AI content moderation offers visual content screening and filtering at scale, full legal compliance, protection of content moderators, multilingual moderation, and an unseen level of productivity.
While the most known field where content moderation is king is social media, its uses are numerous. Dating platforms, travel booking websites, gaming platforms, all types of online marketplaces and ecommerce, fashion apps and websites, video platforms, forums and communities, educational platforms, networking websites, and messaging apps — this is just a short list of the applications that content moderation has today.
Unleash the Power of Image Recognition for Your Business
With its solid pioneering role in image recognition, Imagga has been the trusted partner of all types of companies in integrating the capabilities of machine learning algorithms in their workflows.
Want to learn how you can boost your business performance and tools with image recognition? Get in touch with us to explore our ready and tailor-made solutions for image tagging, categorization, visual search, face recognition, and more.
8 Prominent Use Cases of Visual Content Moderation Today
Visual content moderation is everywhere in our online experiences today, whether we realize it or not. From social media and online gaming to media outlets and education platforms, the need to make digital environments safe for everyone has brought about the necessity for filtering user-generated content—especially the abundant images, videos, and live streamings.
Content moderation has changed dramatically over the last decade thanks to machine learning algorithms. A while ago, most content had to be reviewed by human moderators, which was a slow and potentially harmful process for the people involved. With the rapid growth of online content, it became apparent that content moderation needs a technical boost.
Artificial intelligence has contributed to a massive upgrade in the moderation process. It has brought scalability that can match the sheer amount of content constantly uploaded online. Moderation platforms powered by the evolution of image recognition have additionally revolutionized the filtering process for visual content, as they can automatically remove the most harmful, inappropriate, and illegal items.
To get an idea of visual content moderation's current applications, let’s review eight of its most prominent use cases and how it’s improving users' online environments.
1. Social Media Platforms
Visual content moderation is of utmost importance for social media platforms. They are often under scrutiny because they handle inappropriate content, such as violence, extremism, hate speech, explicit content, and more.
Moderation for social media platforms is especially challenging due to a few key factors — the sheer amount of user-generated content, real-time publishing and circulation, and nuances in context. It takes time and adjustments for algorithms to attune to spotting cultural differences in content. The same goes for finding the right approach and applying different tolerance levels towards sensitive content depending on the cultural background.
Striking the balance between being lenient with harmful content and unnecessary censoring is a daunting task. The scalability and growing accuracy of computer vision is thus of immense help in content moderation for images, video, and live streaming.
2. E-commerce Platforms and Classified Ads
The application of visual content moderation in e-commerce and classified ads is wide today. These platforms collect a vast amount of user data—not only through user-generated content. They also gather information through cookies, user profiling, and preference tracking, which feeds into the analysis of their user base and respective strategies.
Some of the biggest issues that such platforms face include controlling and removing inappropriate and counterfeit product listings, as well as scams and fraud by bad actors.
AI-powered image content moderation provides a way to handle the diversity and volume of product listings and to ensure the policy compliance of all posts and user accounts.
3. Online Gaming and Virtual Worlds
Just like social media and e-commerce, online gaming, and virtual world platforms deal with abundant amounts of user-generated content.
All the different elements in the virtual environment, such as user avatars, gaming assets, and exchanges between users, require moderation to prevent exposure to harmful and inappropriate content.
Live streams are a particularly challenging aspect of visual content moderation for online gaming and virtual worlds. Real-time moderation is specific and requires a robust and well-planned moderation approach that AI can offer.
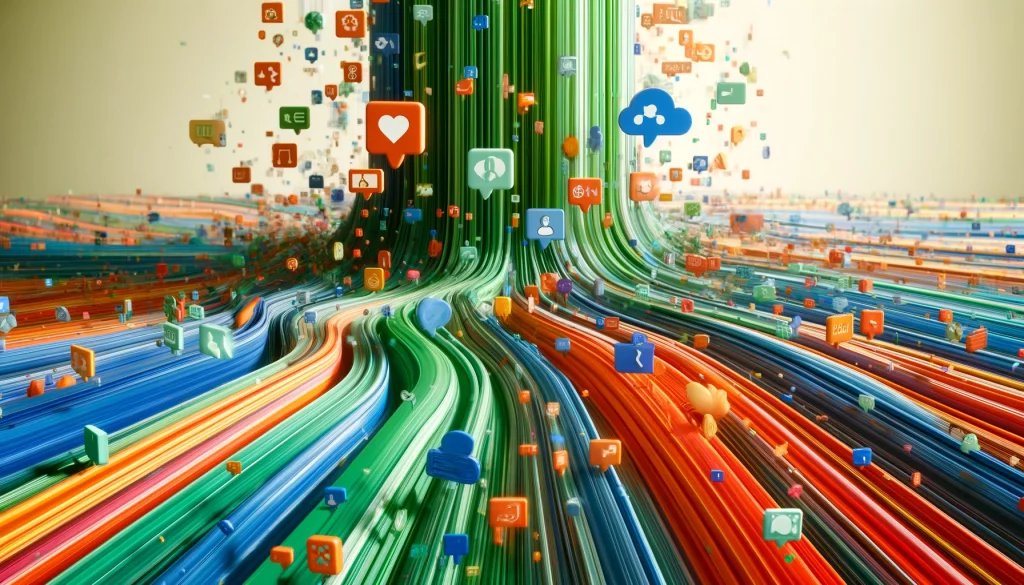
4. Online Forums and Community Platforms
Online forums and community platforms are other types of platforms that rely heavily on user-generated content, which automatically means extensive content moderation.
Online forums are often thematic, while community platforms can be attached to a certain brand, game, or product.
In both cases, users contribute text and visual content in their exchanges with other community members. Content moderation thus aims to make the online environment safe while also providing users with the freedom to express themselves and communicate.
5. Dating Platforms
Dating websites and apps need to be particularly careful in their functioning because users expect a truly safe environment for their personal exchanges.
As with other online platforms, user-generated content must be screened to protect the community and allow free and safe communication.
Visual AI is of immense help in moderating the visual content shared among dating platform users.
6. Education and Training Platforms
Upholding quality standards is key for the success of education platforms, and like all other online platforms, they are susceptible to a number of content risks.
Stopping plagiarism and copyright infringement, as well as monitoring the quality and compliance of educational content are thus of utmost importance for educational platforms.
This requires robust visual content moderation, having in mind that a large part of educational materials today are in the form of videos — and AI-powered content moderation is the logical answer.
7. News and Media Outlets
News and media outlets are facing unprecedented challenges in the digital age. Fake news, doctored content, and misinformation are abundant, creating a constant sense of uncertainty about what we can accept as true.
To protect the truth in these interesting times, news channels and media platforms have to rely also on content moderation for their digital outlets — both for their own content and for user-generated content.
Platforms that allow user comments on news content have to moderate large amounts of data to filter out misinformation, hate speech, and spam — and computer vision is a trusted tool in this process.
8. Corporate Compliance and Governance
Content moderation is not applied only in user online platforms but has its place in corporate management, too.
Data protection is of utmost importance for large companies that need to handle large amounts of data and have big teams.
Visual content moderation based on AI comes in especially handy in spotting sensitive data being shared or distributed in breach of privacy policies.
Learn How Visual Content Moderation Can Help Your Business
Visual content moderation is a must-have tool for ensuring user safety across various types of industries today.
Powered by artificial intelligence and machine learning algorithms, Imagga’s computer vision platform is a trusted partner in optimizing visual content moderation.
To learn how you can embed it in your business and get started, just contact us.
5 Innovative Uses of Image Recognition in Healthcare
Image recognition in Healthcare powered by Artificial Intelligence holds immense promise for revolutionizing the healthcare field—and it is already delivering on this promise. Through accurate object detection and ever-improving image classification and segmentation, AI-powered image recognition is the leading source of innovation in medical services.
The most groundbreaking uses of computer vision today are in medical diagnosis. With the precision of image recognition in processing medical imagery and identifying various conditions, the overall accuracy of diagnostics and early detection can rise exponentially. The same goes specifically for cancer and tumor detection, whose early discovery is of the utmost importance for saving lives and improving patients' conditions.
Yet another revolutionary use of image recognition-powered tools is in surgical assistance. Robotic guidance and analysis based on AI allows for unseen levels of precision and speed in performing operations, all the while reducing hospital stay and time needed for patient recovery.
Image recognition also offers great advancements in inpatient treatment and rehabilitation. It allows flexible and tailor-made solutions for each individual and saves time and resources for medical institutions and practitioners. Computer vision capabilities also bring a powerful boost to human error prevention in medical diagnostics and treatment, along with improvements in specialist training and excellence.
While privacy considerations around the use of AI in medicine are important and should always be addressed and resolved, the possibilities that image recognition offers in healthcare are massive. In fact, making the best use of the life-saving power that AI-based image recognition tools offer can be an important milestone for humanity.
Let’s go through five of the most promising and innovative uses of image recognition in healthcare that are not science fiction — but are already in use in various places across the globe.
#1. Powerful Medical Diagnostics Based on AI
Speed and precision are crucial when it comes to saving people’s lives. Diagnostics is the first step to preventing detrimental conditions, prolonging life, and improving health. With the help of image recognition, medical specialists today are able to dramatically improve the accuracy of their diagnostics and the time needed for the process.
Medical imaging based on AI, also known as medical image analysis, is especially helpful in the diagnostics process. It is being used in magnetic resonance imaging (MRI), X-ray, ultrasound, and other methods. Medical image analysis renders a visual model of internal tissues and organs, making detecting abnormalities easier and faster. This allows medical specialists to get better visual data and thus make informed diagnosing decisions.
Besides the actual image recognition capabilities, AI-powered medical platforms can process large amounts of data in minimal time and use deep learning to acquire new knowledge — which complements the well-informed human judgment necessary in diagnostics. In addition, image recognition tools access and create large databases of medical cases that are irreplaceable in the process of comparing images and medical conditions in order to help diagnostics. Thus, AI-powered algorithms are better equipped to spot patterns and detect even minor condition changes — which in turn helps medical practitioners in their clinical decision-making and consecutive patient treatment.
Some of the common uses of computer vision tools in medical diagnostics include detecting cardiovascular diseases, musculoskeletal injuries, and neurological diseases, among others.
#2. Early Cancer and Tumor Detection Using Image Recognition
Medical tools based on image recognition can detect and analyze anomalies in computerized tomography (CT), magnetic resonance imaging (MRI), and positron emission tomography (PET) scans. Machine learning algorithms trained with enormous amounts of images can identify, compare, and process large data chunks to provide quick and precise detection of malignant formations.
Computer vision can help medical specialists, especially radiologists and pathologists, improve the early and accurate detection of different types of cancer and tumors. Platforms powered by image recognition can spot anomalies faster and more efficiently, thus helping medical practitioners in their assessment and diagnosis.
Image recognition tools based on AI are already being used to detect and diagnose skin and breast cancer. They are particularly good at differentiating between cancerous skin lesions and other skin problems that are not life-threatening. Computer vision has also brought innovation and precision in breast cancer diagnosis, as it can identify cancerous areas faster than the rest of the tissue.
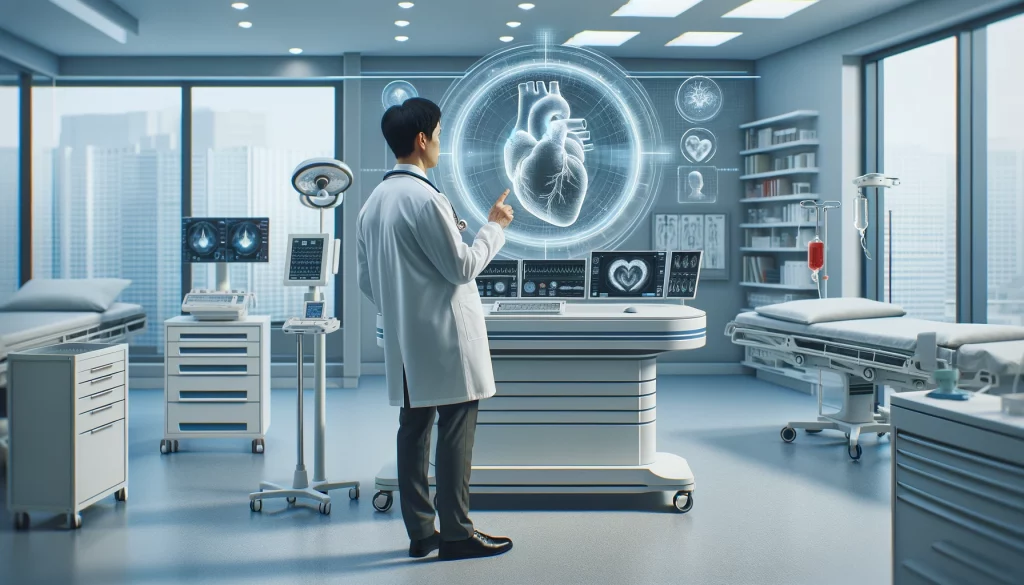
#3. Precise Surgical Guidance Assisted by Image Recognition
Robot surgical guidance is another highly promising use of image recognition in healthcare. Based on the patient's medical records, robots process and analyze data so that they can assist surgeons on the operating table. Using AI, robots draw information from previous surgeries to provide surgeons with guidance and the best applicable techniques.
The benefits of using robotic guidance are already clear. They include precision in incisions and faster hospital rehabilitation for patients. Robot surgical guidance has also been shown to reduce medical complications and the invasiveness of surgery procedures. Overall, robotic surgery assistance is boosting the safety and accuracy of surgeries in which it has been applied because it provides surgeons with a much higher level of control over the process.
Robot-guided surgeries have already been practiced in a number of medical fields and in very complicated cases, including eye surgeries, heart interventions, and orthopedics, among many others. One of the biggest benefits that they bring to surgeons is the precise localization of the surgical area based on image analysis of CT, MRI, ultrasound, PET, and other techniques. This allows for better preoperative planning based on the patient’s exact 3D modeling and further feeds into improved guidance during the actual performing of incisions.
#4. Better Patient Personalized Treatment and Rehabilitation
Image recognition provides innumerable benefits in providing personalized treatment for patients, as well as effective rehabilitation and chronic illness care.
An individual approach to diagnostics and personalized treatment is difficult to achieve without the help of technology because they are too time-consuming. Creating treatment plans that are specifically prepared to cater to the needs of the patient can be much more effective and can be realized with the help of AI-powered tools.
In the field of rehabilitation, computer vision tools allow medical practitioners to provide care and advice virtually so that patients can recover at ease in their homes. Specialists can provide the needed physical therapy consultations, while also tracking patients’ progress. In addition, image recognition technologies are also being used in fall prevention systems for elderly and injured patients.
Virtual nursing assistants are another innovation that can further improve personalized treatment and rehabilitation. Virtual assistants can communicate with patients to help them with their medication, as well as to direct them to the most appropriate medical service providers. This extra care, available around the clock, can reduce hospital visits and provide better rehabilitation support to patients.
AI-based image recognition applications are also very helpful in monitoring chronic diseases by tracking health metrics like vital signs, levels of activity, nutrition, and many other factors. Patients can thus stay on top of the data and take the necessary steps to alleviate symptoms. The data can then be used by medical practitioners to spot patterns and adjust treatment based on them.
#5. Improved Error Prevention and Medical Excellence with AI
Decreasing the level of human error in medical services is a major area in which image recognition can provide significant innovation and improvement. For example, using convolutional neural networks in brain tumor detection has resulted in a reduction in human error and a boost in early identification and treatment.
Besides reducing misdiagnosing and various other errors that lead to worsened health or even death of patients, AI-powered systems can also be useful from an administrative point of view. They can provide a repository for the diagnoses and processes for each patient, thus allowing for a higher degree of accountability and transparency in decisions and treatment.
Image recognition has also found an important place in specialist training. One such area is surgeon training. Surgical platforms based on simulation offer a practical and effective way for novel surgeons to train their skills, gain confidence, and get feedback on their performance.
In addition, image recognition models are used to help junior medical staff in their training and in actual diagnosing. Computer vision can assist them in deep analysis and accurate interpretation of patients’ scans so that they are sure they are not missing important details. Senior doctors can also use such capabilities to monitor and guide the work of medical staff in training. This can lead to a reduction of stress and improved confidence for junior doctors and the overall improvement of diagnostics.
Image recognition allows for great improvements in various other areas to boost medical excellence. Some examples include tracking hospital hygiene and upholding high standards, preventing diseases and infections in hospitals, applying lean management techniques in healthcare, and many more.
Imagga: Exploring The Power of Image Recognition in Healthcare
Imagga offers all-in-one image recognition solutions that find powerful applications in a wide variety of fields, including healthcare. Our tools are based on the robust object and shape recognition and image classification, as well as power-up diagnostics, scanning, prevention, and more.
Get in touch to learn more about how our machine learning-powered image recognition can boost your healthcare services.
Check out Kelvin Health, our medical spin-off, to explore the capabilities of Thermography AI for easy diagnostics in a variety of medical contexts.
Beyond Human Vision: The Evolution of Image Recognition Accuracy
Technologies based on Artificial Intelligence are all the rage these days — both because of their stunning capabilities and the numerous ways in which they make our lives easier and because of the unknown future that we project they may bring.
One particular stream of development in the field of AI is image recognition based on machine learning algorithms. It’s being used in so many fields today that it’s challenging to start counting them.
In the fast-paced digital world, image recognition powers up crucial activities like content moderation on a large scale, as required by the exponentially growing volume of user-generated content on social platforms.
It’s not only that, though: image recognition finds great uses in construction, security, healthcare, e-commerce, entertainment, and numerous other fields where it brings unseen benefits in terms of productivity and precision. Think also about innovations like self-driving cars, robots, and many more — all made possible by computer vision.
But how did image recognition start, and how did it evolve over the decades to reach the current levels of broad use and accuracy that sometimes even surpass the capabilities of human vision?
It all started with a scientific paper by two neurophysiologists in the distant 1959, which was dealing with research on cat’s neurons…
Let’s dive into the history of this field of AI-powered technology in the sections below.
1950s: The First Seeds of the Image Recognition Scientific Discipline
As with many other human discoveries, image recognition started out as a research interest in different other fields.
In the last years of the 1950s, two important events occurred that were far away from the creation and use of computer systems but were central to developing the concept of image recognition.
In 1959, the neurophysiologists David Hubel and Torsten Wiesel published their research on the Receptive Fields of Single Neurons in the Cat's Striate Cortex. The paper became popular and widely recognized, as the two scientists made an important discovery while studying the responses of visual neurons in cats and in particular, how their cortical architecture is shaped.
Hubel and Wiesel found that the primary visual cortex has simple and complex neurons. They also discovered that the process of recognizing an image begins with the identification of simple structures, such as the edges of the items being seen. Afterward, the details are added, and the whole complex image is understood by the brain. Their research on cats thus, by chance, became a founding base for image recognition based on computer technologies.
The second important event from the same year was the development of the first technology for the digital scanning of images. Russel Kirsch and a group of researchers led by him invented a scanner that could transform images into numbers so that computers can process them. This historical moment led to our current ability to handle digital images for so many different uses.
1960s and 1970s: Image Recognition Becomes an Official Academic Discipline
The 1960s were the time when image recognition was officially founded. Artificial intelligence, and hence image recognition as a significant part of it, was recognized as an academic discipline with growing interest from the scientific community. Scientists started working on the seemingly wild idea of making computers identify and process visual data. These were the years of dreams about what AI could do — and the projections for revolutionary advancements were highly positive.
The name of the scientist Lawrence Roberts is linked to the creation of the first image recognition or computer vision applications. He put the start of it all by publishing his doctoral thesis on Machine Perception of Three-Dimensional Solids. In it, he details how 3D data about objects can be obtained from standard photos. Roberts’ first goal was to convert photos into line sketches that could then become the basis for 3D versions. His thesis presented the process of turning 2D into 3D representations and vice versa.
Roberts’ work became the ground for further research and innovations in 3D creation and image recognition. They were based on the processes of identifying edges, noting lines, construing objects as consisting of smaller structures, and the like, and later on included contour models, scale-space, and shape identification that accounts for shading, texture, and more.
Another important name was that of Seymour Papert, who worked at the AI lab at MIT. In 1966, he created and ran an image recognition project called “Summer Vision Project.” Papert worked with MIT students to create a platform that had to extract the background and front parts of images, as well as to detect objects that were not overlapping with others. They connected a camera to a computer to mimic how our brains and eyes work together to see and process visual information. The computer had to imitate this process of seeing and noting the recognized objects — thus, computer vision came to the front. Regretfully, the project wasn’t deemed successful, but it is still recognized as the first attempt at computer-based vision within the scientific realm.
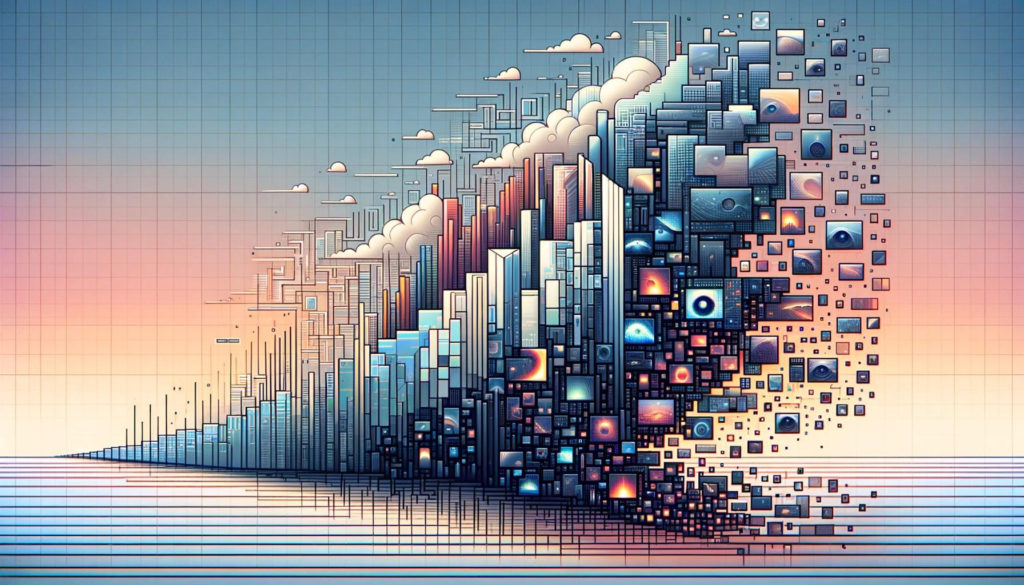
1980s and 1990s: The Moves to Hierarchical Perception and Neural Networks
The next big moment in the evolution of image recognition came in the 1980s. In the following two decades, the significant milestones included the idea of hierarchical processing of visual data, as well as the founding blocks of what later came to be known as neural networks.
The British neuroscientist David Marr presented his research "Vision: A computational investigation into the human representation and processing of visual information" in 1982. It was founded on the idea that image recognition’s starting point is not holistic objects. Instead, he focused on corners, edges, curves, and other basic details as the starting points for deeper visual processing.
According to Marr, the image processing had to function in a hierarchical manner. His approach stated that simple conical forms can be employed to put together other complex objects.
The evolution of the Hough Transform, a method for recognizing complex patterns, was another important event around this period. The algorithm was foundational for creating advanced image recognition methods like edge identification and feature extraction.
At the beginning of the 1980s, another significant step forward in the image recognition field was made by the Japanese scientist Kunihiko Fukushima. He invented the Neocognitron, seen as the first neural network categorized as ‘deep’. It is believed to be the predecessor of the present-day convolutional networks used in machine learning-based image recognition.
The Neocognitron artificial network consisted of simple and complex cells that identified patterns irrespective of position shifts. It was made up of a number of convolutional layers, each triggering actions that served as input for the next layers.
In the 1990s, there was a clear shift away from David Marr’s ideas about 3D objects. AI scientists focused on the area of recognizing features of objects. David Lowe published the paper Object Recognition from Local Scale-Invariant Features in 1999, which detailed an image recognition system that employs features that are not subject to changes from location, light, and rotation. Lowe saw a resemblance between neurons in the inferior temporal cortex and these features of the system.
Gradually, the idea of neural networks came to the front. It was based on the structure and function of the human brain — with the idea of teaching computers to learn and spot patterns. This is how the first convolutional neural networks (CNNs) came about, equipped to gather complex features and patterns for more complicated image recognition tasks.
Again, in the 1990s, the interplay between computer graphics and computer vision pushed the field forward. Innovations like image-based rendering, morphing, and panorama stitching brought about new ways to think about where image recognition could go.
2000s and 2010s: The Stage of Maturing and Mass Use
In the first years of the 21st century, the field of image recognition reshifted towards object recognition as a primary goal. The first two decades were a time of steady growth and breakthroughs that eventually led to the mass adoption of image recognition in different types of systems.
In 2006, Princeton Alumni Fei-Fei Lin, who later became a Professor of Computer Science at Stanford, was conducting machine learning research and was facing the challenges of overfitting and underfitting. To address them, in 2007, she founded Imagenet, an ameliorated dataset that could power machines to make more accurate judgments. In 2010, the dataset consisted of three million visual items, tagged and categorized in over 5,000 sections. Imagenet served as a major milestone for object recognition as a whole.
In 2010, the first Imagenet Large Scale Visual Recognition Challenge (ILSVRC) brought about the massive evaluation of object identification and classification algorithms.
It led to another significant step in 2012 — Alexnet. The scientist Alex Krizhevsky was behind this project, which employed architecture based on convolutional neural networks. Alexnet was recognized as the first use of deep learning. This brought about a significant reduction in error rates and boosted the whole field of image recognition.
All in all, the progress with Imagenet and its subsequent initiatives was revolutionary, and the neural networks set up back then are still being used in various applications, such as the popular photo tagging on social networks.
2020s: The Power of Image Recognition Today
Our current decade is a witness to a powerful move in image recognition to maximize the potential of neural networks and deep learning algorithms. With their help, deep learning algorithms are constantly evolving and gaining higher levels of accuracy, as well as pushing further the advancement of the whole field with a focus on classification, segmentation, and optical flow, among others.
The industries and applications in which image recognition is being used today are innumerable. Just a few of them include content moderation on digital platforms, quality inspection and control in manufacturing, project and asset management in construction, diagnostics and other technological advancements in healthcare, automation in areas like security and administration, and many more.
Learn How Image Recognition Can Boost Your Business
At Imagga, we are committed to the most forward-looking methods in developing image recognition technologies — and especially tailor-made solutions such as custom categorization — for businesses in a wide array of fields.
Do you need image tagging, facial recognition, or a custom-trained model for image classification? Get in touch to see how our solutions can power up your business.