Image Recognition Explained - Part 1
Image recognition and the technologies behind it are quickly finding application in industries spanning from media, retail and telecoms to automotive, healthcare, security and surveillance. Still fairly new as a concept and turned into a hype word, image recognition often lies within the blind-spot of many businesses that can benefit significantly from putting it to use. Talking to prospects we find out that a huge part of the potential users of the technology don’t realize that they have a use case for the technology. And that it is affordable too.
In the Image Recognition Explained series we’ll cover the major technologies related to it and their applications to help business owners understand if they have a use case for image recognition or not.
What is image recognition?
Image recognition is the ability of computer software to recognize objects, places, people, faces, logos, and even emotions in digital images and videos. A computer algorithm is being trained with a large amount of visual data using machine learning and as a result the algorithm starts recognizing the type of visual content it has been trained with. Unlike the human brain, artificial intelligence sees the images as complex mathematical matrices, and can recognize people and objects within very high confidence levels.
Which are the typical applications of image recognition in different industries?
Automatic classification and categorization – technologies such as image tagging and categorization allow automation of processes that alternatively involve gigantic manual effort and are often times impossible to tackle. Automatic tagging and classification of huge visual databases are used for organization and discovery of media content by DAM systems, stock photography platforms, hotel and travel booking systems, and can be used by virtually any platform hosting visual content in the range of hundreds of thousands to billions of images.
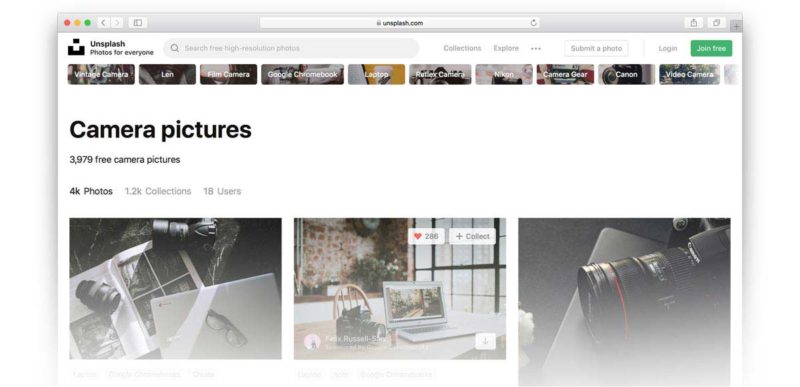
Read how Unsplash improved their image search and user experience.
Photo and video organization – technologies such as image tagging, facial recognition and Not Safe for Work (NSFW) models are used to enable image organization and discovery for Telecom clouds, Dropbox and Google Photos-like apps. Beside securely storing user media content, companies need to provide value by offering better search and discovery capabilities on a level equal or superior to the widely used iCloud Photos and Google Photos.
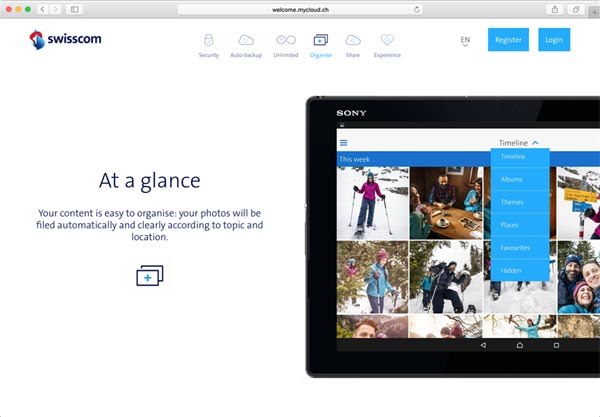
Read how Imagga Image Recognition technology helped Swisscom to provide better visual content organization for individuals and businesses.
Users profiling & insight – image recognition can be used for matching interests & behavior with visual content. Used by advertisement platforms and social media analytics systems image recognition technology can provide valuable insights on users’ preferences and sentiment for a product or brand based on analyzing the content of the pictures she shares online.
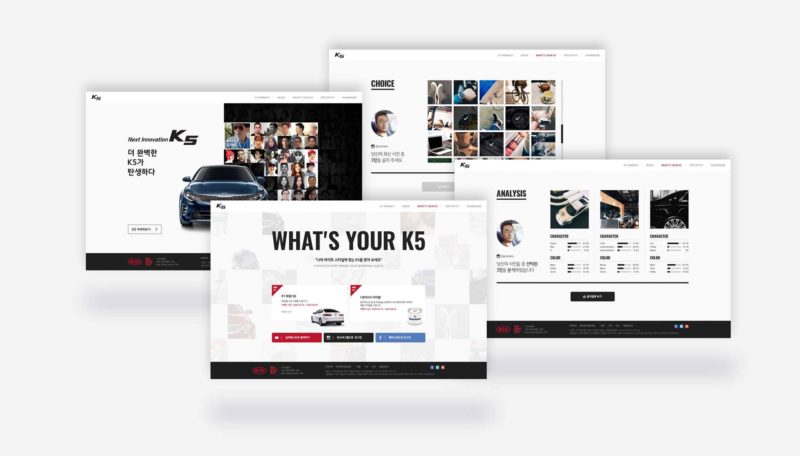
Easier product discovery – fashion retail, e-commerce and home goods leaders are building visual search within their websites and mobile apps to make product discovery easier, offer product recommendations and alternatives to products out of stock.
Facial detection and recognition – companies such as telecoms use face recognition technology allow their users to organize their personal photos by the people in the images. Typical applications of face recognition are in video surveillance and analysis and access control.
Visual and text content moderation – virtually any platform that operates with user generated content (UGC) needs to monitor it and remove illegal and abusive images, videos and text. Learn how Imagga Content Moderation solution can help you address the screening of massive content in order to avoid hurting vulnerable groups and legal issues.
What’s next?
Check out the video for Image Tagging
Check out the video for Content Moderation
Stay tuned for the technologies behind image recognition explained…
How Imagga Facial Recognition Works?
We’re excited to share that we’ve just added another powerful image recognition capability to our offering – Facial Recognition API for face detection and recognition of faces in still images and live stream videos.
Using the API, companies can implement face recognition functionality in their web, desktop, and mobile applications and systems.
How the facial recognition algorithm sees a face?
The face recognition algorithm “sees” a face as a complex numerical code containing hundreds of facial measurements. Examples of facial measurements include: the distance between the eyes, the width of the nose, length of the jawline, depth of the eye sockets, shape of your cheekbones, and more. These specific facial measurements are called nodal points, and each face has 500 such points. The unique combination of all these measurements creates an anonymous numerical code, which represents the face in the database.
How facial recognition works?
- Face detection
First, the face is extracted from the rest of the image using a technology called object localization. - Face analysis
Often the head is tilted, which can distort the data, so the technology locates the face attributes like eyes, nose and ears to correct the face rotation or tilt. Then it takes the facial measurements of the reference image and creates the unique numerical code we mentioned above. - Comparison
The last step is looking for the exact match or one with a high level of confidence in a pre-defined or custom, still or dynamic database.
Is face verification possible with Imagga Facial Recognition API?
Face verification compares a reference image of a face to another already present in the database and checks if there is a match. Our API now supports this functionality out-of-the-box and you can implement it directly in your application. Read how.
Facial recognition use cases
The facial recognition market is expected to grow at a fast pace due to increased adoption in both law enforcement and non-law enforcement sectors.
Source: Markets and Markets
Editorial Management Systems use facial recognition to identify and recognize celebrities, politicians, and other public figures — for efficient organization and search in large media archives.
It can be used for personal photo organizations by companies such as telecoms allowing their users to organize their personal photos by the people in the images.
In the video surveillance and analysis sector, facial recognition can be used for the analysis of video footage to identify key people and their attributes like age, gender and ethnicity.
Another typical application of facial recognition is access control. The technology can be used to ensure that only authorized individuals enter facilities like bank vaults, labs, and other sensitive locations, as well as for the time-tracking of employees.
Read more about the image recognition trends in 2020.
You have a case for image recognition? Let’s talk
ViewBug Uses Imagga AI Content Moderation to Filter Through Millions of Images
ViewBug is a web and mobile platform for visual creators with more than 2 million members and tens of thousands of image uploads every day. Like a Behance for the enthusiast photographer it gives photographers opportunities to be discovered and improve their skills offering a full suite of tools that help them learn, work better, and make more money.
As many user generated content platforms, ViewBug needs a safety shield against offensive content that could harm the brand’s reputation, disturb vulnerable groups and cause compliance and legal issues. Filtering out adult images, which are not allowed in their platform, posed a challenge for the ViewBug team given the large scale volume of visual content generated by their users every day. They needed an automated content moderation software that was reliable, precise, accessible and easy to implement.
After looking at a few solutions, they chose Imagga Not Safe for Work (NSFW) classifier - adult image content moderation categorizer trained on state of the art image recognition technology. It was integrated in the code, verifying every single image upload in real-time to make sure that it is not explicit content.
The NSFW classifier is part of Imagga AI-powered Content Moderation solution. It’s designed to help visual content platforms of any size keep their reputation and content safe in a cost-efficient and scalable way. It will offer automated detection of diverse and customizable categories of inappropriate content and can be deployed in the cloud and on-premise.
You have a similar need and you’re not sure what’s the best solution for you? Get in touch with us to discuss your case.
Image Recognition Trends in 2020
The image recognition market is estimated to reach USD 38.9 billion by 2021, compared to USD 15.9 billion in 2016 — more than 100% increase for the projected period, according to data by Markets and Markets research. Companies in e-commerce, automotive, content sharing, healthcare and security are rapidly implementing image recognition and driving the adoption of the technology. Other contributing factors are the sophistication of facial recognition, content moderation and other algorithms, the rise in popularity of media cloud services, the surge in mobile devices equipped with cameras and hardware technological advancements.
What is Image Recognition?
Image recognition refers to technologies that can detect and identify people, places, objects, logos, emotions and other variables in still images and videos. Image recognition is a subset of computer vision and is based on AI and machine learning.
What is image recognition used for?
One of the most widely used applications of image recognition is the automatic detection and tagging of people, objects and places in large visual databases. Other technologies with increasing popularity are facial recognition used in security, biometrics and personal photos platforms, and content moderation for filtering offensive and illegal visual content.
Which Image Recognition Technologies Will be on the Rise in 2020?
Content Moderation
Billions of users share content online and tons of it falls within a range from inappropriate to outright illegal. Platforms operating with user-generated content (UGC) face a significant challenge — how to efficiently and effectively monitor UGC and block inappropriate and offensive images, videos and text. Content moderation done poorly can disturb vulnerable groups, hurt the brands reputation and cause compliance and legal issues.
Who takes care of the “toxic digital garbage”?
Content moderation can be performed by humans, AI or both. More than 100,000 people worldwide monitor the most violent, pornographic, exploitative and illegal content to protect internet users from online bullies and criminals. The job is tough and can take its emotional and mental toll.
Cognizant, one of the top content moderation companies, just recently announced its decision to exit the business and laid off 6,000 people, performing content moderation for clients such as Facebook among others. A memo from the CEO of the company to its employees stated that while thousands of jobs will be eliminated, Cognizant will contribute to the development of machine-learning systems that can replace the human moderators job.
AI-powered or automated content moderation addresses both the ethical and economic side of the problem. The algorithms, processing the majority of the content and sending just a small fraction of it to human moderators can significantly mitigate the detrimental effect this job has on people. Furthermore it is vastly more productive, easier to scale and ultimately less expensive than human moderation.
Automated content moderation explained
Image Tagging
Image tagging is one of the earliest applications of image recognition in businesses, however its adoption will continue to grow as more companies embrace AI to offer better user experience or improve internal workflows.
What is image tagging?
Image tagging is the automatic assignment of relevant tags or keywords to vast collections of images and videos. In order for this to happen a deep learning model is trained to analyze the pixel content of visuals, to extract their features and detect objects of interest. It is a cost-effective and time-saving solution for companies operating with massive amounts of image content often coming from different sources.
This technology, often times combined with complementing capabilities such as image categorization solves a common pain of platforms operating with large image databases — inadequate image descriptions making the images hard to find, while searchability is critical for these platforms. Often times the images are uploaded by different users, vendors or employees which results in incoherent meta-data describing the images or even lack of any description. This is where image tagging comes in — it can tag coherently a multi million image database in a matter of hours.
Image tagging is widely used in stock photography and photo sharing. Read why Unsplash chose to incorporate Imagga image recognition API in their tagging system.
Advertisers, publishers and ad agencies also use the image tagging technology to get better at contextual advertising. SEEDPOST used Imagga Image Tagging and Color Extraction APIs to analyze image content form user’s social accounts and created, based on image understanding, 36 different lifestyles that match the highly customizable experience of KIA’s new K5 (Optima) model. Read the case study.
Visual Search
Visual search means searching for an image with a similar image. The technology allows users to search and find products similar to the ones they shot with their camera or downloaded from the web. In contrast, the search for images as we know it today relies on the accuracy of the images text description. Visual search delivers, where text search fails.
Apparel, home decor brands and retailers are among the early adopters of the technology, as it allows for easier product discovery and shortens the path to conversion.
Another benefit of the technology lies in the ability to provide product recommendations based on true similarity rather than other users’ preferences. Visual search can power similar products suggestions to offer a bigger choice or to provide alternatives to products out of stock.
Read more about how retailers use visual search for a competitive advantage
Facial Recognition
Facial recognition is widely used in government applications and law enforcement, and is gaining more popularity in commercial applications for use cases such as contactless payments, access control through facial biometrics and more. The technology is expected to witness the highest growth rate in the upcoming years due to increased adoption in both law enforcement and non-law enforcement sectors.
Image source: https://www.alliedmarketresearch.com/image-recognition-market
Facial recognition can be used as a key component in every photo organization application or service — allowing its users to search and filter photos by people in the shot.
Editorial Management Systems use facial recognition to identify and recognize celebrities, politicians, and other public figures — for efficient organization and search in large media archives.
In the security and law enforcement sectors, facial recognition is used for detection and prevention of crimes. An example of such application is an app that helps police officers identify individuals on the field giving them data about these individuals, so they know who they are dealing with from a safe distance.
Another typical application of facial recognition is access control. The technology can be used to ensure that only authorized individuals enter facilities like bank vaults, labs and other sensitive locations, as well as for time-tracking of employees.
Are you future proof?
If you are operating with large visual databases on the front-end and/or back-end of your business applications you most probably have a case for visual AI. The wide array of technologies commonly known as image recognition can help you achieve results which would be impossible without AI, or deliver a better user experience to your end users. Our 10+ years of expertise in delivering image recognition solutions to companies across diverse industries, large and small, has shown us that most cases require a custom approach and a combination of 2 or more technologies. We’ve helped companies coming to us with a vague definition of a need or a straightforward list of requirements.
You have a case for image recognition? Let’s talk!
Imagga Among the Top Performers in Recent Comparison of Image Recognition APIs
Image understanding relies heavily on accurate multi-label classification, which has significantly improved with the appearance of deep learning technologies. Researchers from the Department of Software and Information System Engineering Ben-Gurion University of the Negev, Israel evaluated and compared 10 of the most prominent publicly available APIs for deep learning multi-label image classification, i.e. image object classification, in a best-of-breed challenge.1 These include Imagga API, Watson IBM Visual Recognition API, Clarifai API, Microsoft Computer Vision API, Wolfram Alpha Image Identification API, Google Cloud Vision API, as well as several open-source frameworks with the capability of image classification, such as Caffe, DeepDetect, OverFeat, and TensorFlow.
We are proud to share the results of this independent study by the Ben-Gurion University team, as Imagga made it to the top four performing APIs together with Microsoft’s Computer Vision, TensorFlow and IBM’s Visual Recognition.
The evaluation was performed on 1000 images of the Visual Genome benchmark dataset, using 12 well recognized similarity metrics and an additional semantic similarity metric allowed deeper insights for comparison. These metrics evaluate the prediction performance of the APIs based on whether a predicted label exists in the ground truth label or how semantically close it is to it. Three APIs outperformed the rest when evaluating the APIs labels’ predictions with these well-known metrics: Microsoft’s CV, IBM’s, and Imagga’s APIs.
The authors of the paper concluded, that if one is looking for a solution able to handle with high recall and precision a dataset with as many predicted labels as possible, including several which might not relevant (false positives), the Imagga API should be considered as top choice.
PAPER REFERENCE
1Adam Kubany, Shimon Ben Ishay, Ruben-sacha Ohayon, Armin Shmilovici, Lior Rokach, Tomer
Doitshman, April 2019. Semantic Comparison of State-of-the-Art Deep Learning Methods for Image Multi-Label Classification. arXiv:1903.09190v2
Imagga image recognition API features image tagging, image categorization, smart cropping and visual search. The technology can be delivered as a Cloud API or/and an on-Premise solution.
How Retailers Use Visual Search to Gain Competitive Advantage
As computer vision AI evolves and computers start “to see and understand” images better, visual search implementation and usage is growing in popularity. Apparel and home goods leaders are building visual search within their websites and mobile apps to make product discovery easier and increase conversions. Brands in the e-commerce space need to prepare to incorporate it in their digital shopping experience to take advantage of the shift in users search habits.
What is visual search and why should e-commerce business care about it?
In a text-based search, the user searches for an image by describing it with words or tags. In visual search, also called visual similarity search or image-based search, the user snaps an image with her camera and uploads it to the mobile application or website. Machine learning and computer vision technology in the background scrutinize the product/image inventory analyzing shapes, colors, and patterns and returns results that are visually similar to the reference image.
Visual search opens new possibilities in scenarios where the user doesn’t know how to describe the item she’s looking for but has a visual reference.
The adoption of visual search is growing
We are visual creatures able to process images faster than text. The human brain processes images hundreds of thousands of times faster than text and the majority of information transmitted to the brain is visual. It doesn’t come as a surprise that given the opportunity to search for information with visuals users are quickly adopting this search behavior.
Visual search stats in a nutshell
- The image recognition market is expected to grow to $25.65 billion by 2019
- The human brain processes images 60,000 times faster than text, and 90 percent of information transmitted to the brain is visual.
- The human brain can identify images seen for as little as 13 milliseconds. MIT research
- Pinterest reported more than 600 million visual searches every month across its visual search engine one year after its official release
- Images are returned for 19% of search queries on Google
- 85% of respondents put more importance on visual information than text information when shopping online for clothing or furniture. The Intent Lab
- Companies who redesign their websites and apps to support visual and voice search are expected to see 30% increase in digital commerce revenue by 2021. Gartner
ASOS, Farfetch, Target, Wayfair and Macy’s, to name just a few of the giant retailers, have visual search capabilities built into their e-commerce websites or mobile apps.
Consumers are increasingly using visual search and brands need to start thinking about incorporating it in their digital shopping experience.
What are the benefits of visual search?
Enhances product discovery
Visual search makes product discovery easier by allowing users to search with images for the closest possible or exactly the same representation of the item they are looking for.
Delivers where text search fails
It helps to answer questions which are hard to verbalize such as “I’m looking for a jacket like this one” or “what’s the best price for this sofa”.
Product recommendation made easy
Visual search provides the technology for a product recommendation system based on actual similarity and not other users preferences. Brands use it to power similar products suggestions to offer a bigger choice.
Alternatives to products out of stock
Visual search can help to decrease shopping cart abandon rate by using it to offer an alternative to products out of stock.
Visual search improves product discovery, delivers where text search fails, increases conversions, and decreases shopping cart abandonment while also offering rich media experience to users.
How can Imagga help my company implement visual search on our website or app?
Imagga Visual Search API can be easily integrated with your database. It’s very flexible and you can get feedback and advice from our team before you start. With Imagga Image Recognition API no task is too hard. We offer scalable pricing which adapts to your volume, whether you scale down or up. The functionality is supported by a full feature suite of companion API’s like auto-tagging, color extraction, custom categorization and smart cropping.
For highest accuracy results retrieval, the features relevant for the image database can be custom-defined. Furthermore, our expert machine learning team can help you customize the features relevant to your image database to achieve the highest possible search precision rate.
Not ready to implement visual search in your application yet but looking to maximize the searchable potential of your images ?
There are other ways to improve your products discovery and pave your way to computer vision AI. Many of our customers start with auto-tagging. The technology automatically assigns the proper tags from a list of hundreds of predefined tags. The true potential of Imagga auto-tagging technology though lies in its ability to be trained. It allows you to define tags specific for your particular use case and learns to recognize and suggest them. The AI-powered auto-tagging saves long hours of expensive manual labor and eliminates the inconsistencies resulting from the input of different employees or numerous vendors. In result, you maximize the searchable potential of your images for users and image search engines.
Learn more about Imagga Visual Search